Preface and Acknowledgments
Peter W. Wood
President
National Association of Scholars
This report uses statistical analyses to provide further evidence that our country’s public health bureaucrats gravely mishandled the federal government’s response to the COVID-19 pandemic. Other competent observers have been documenting lapses by the Centers for Disease Control and Prevention (CDC), the National Institutes of Health (NIH), the Chief Medical Advisor to the President, and other authorities since the early days of the pandemic. This report adds to and substantiates many of the previously published criticisms.
The National Association of Scholars (NAS) has been publicizing the dangers of the irreproducibility crisis for years, and now the crisis has played a major role in a public health policy catastrophe. Why does the irreproducibility crisis matter? What, practically, has it affected? Now our Exhibit A is the government’s COVID-19 public health policy.
I don’t need to explain what COVID-19 is to the general reader, but I do need to explain the nature and the extent of the irreproducibility crisis. It has had an ever more deleterious effect on a vast number of the sciences and social sciences, from epidemiology to social psychology. What went wrong in COVID-19 public health policy has gone wrong in a great many other disciplines.
The irreproducibility crisis is the product of improper research techniques, a lack of accountability, disciplinary and political groupthink, and a scientific culture biased toward producing positive results. Other factors include inadequate or compromised peer review, secrecy, conflicts of interest, ideological commitments, and outright dishonesty.
Science has always had a layer of untrustworthy results published in respectable places, and “experts” who were eventually shown to have been sloppy, mistaken, or untruthful in their reported findings. Irreproducibility itself is nothing new. Science advances, in part, by learning how to discard false hypotheses, which sometimes means dismissing reported data that does not stand the test of independent reproduction.
But the irreproducibility crisis is something new. The magnitude of false (or simply irreproducible) results reported as authoritative in journals of record appears to have dramatically increased. “Appears” is a word of caution, since we do not know with any precision how much unreliable reporting occurred in the sciences in previous eras. Today, given the vast scale of modern science, even if the percentage of unreliable reports has remained fairly constant over the decades, the sheer number of irreproducible studies has grown vastly. Moreover, the contemporary practice of science, which depends on a regular flow of large governmental expenditures, means that the public is, in effect, buying a product rife with defects. On top of this, the regulatory state frequently builds both the justification and the substance of its regulations on the basis of unproven, unreliable, and, sometimes, false scientific claims.
In short, many supposedly scientific results cannot be reproduced reliably in subsequent investigations and offer no trustworthy insight into the way the world works. A majority of modern research findings in many disciplines may well be wrong.
That was how the National Association of Scholars summarized matters in our report The Irreproducibility Crisis of Modern Science: Causes, Consequences, and the Road to Reform (2018).1 Since then we have continued our work toward reproducibility reform through several different avenues. In February 2020, we co-sponsored with the Independent Institute an interdisciplinary conference on Fixing Science: Practical Solutions for the Irreproducibility Crisis, to publicize the irreproducibility crisis, exchange information across disciplinary lines, and canvass (as the title of the conference suggests) practical solutions for the irreproducibility crisis.2 We have also provided a series of public comments in support of the Environmental Protection Agency’s rule Strengthening Transparency in Pivotal Science Underlying Significant Regulatory Actions and Influential Scientific Information.3 We have publicized different aspects of the irreproducibility crisis by way of podcasts and short articles.4
And we have begun work on our Shifting Sands project. In May 2021 we published Keeping Count of Government Science: P-Value Plotting, P-Hacking, and PM2.5 Regulation.5 In July 2022 we published Flimsy Food Findings: Food Frequency Questionnaires, False Positives, and Fallacious Procedures in Nutritional Epidemiology. This report, The Confounded Errors of Public Health Policy Response to the COVID-19 Pandemic, is the third of four that we will publish as part of Shifting Sands, each of which will address the role of the irreproducibility crisis in different areas of federal and state policy. In these reports we address a central question that arose after we published The Irreproducibility Crisis.
You’ve shown that a great deal of science hasn’t been reproduced properly and may well be irreproducible. How much government regulation is actually built on irreproducible science? What has been the actual effect on government policy of irreproducible science? How much money has been wasted to comply with regulations that were founded on science that turned out to be junk?
This is the $64 trillion dollar question. It is not easy to answer. Because the irreproducibility crisis has so many components, each of which could affect the research that is used to inform regulatory policy, we are faced with many possible sources of misdirection.
The authors of Shifting Sands include these just to begin with:
malleable research plans;
legally inaccessible data sets;
opaque methodology and algorithms;
undocumented data cleansing;
inadequate or non-existent data archiving;
flawed statistical methods, including p-hacking;
publication bias that hides negative results; and
political or disciplinary groupthink.
Each of these could have far-reaching effects on government regulatory policy—and for each of these, the critique, if well-argued, would most likely prove that a given piece of research had not been reproduced properly, not that it had actually failed to reproduce. (Studies can be made to “reproduce,” even if they don’t really.) To answer the question thoroughly, one would need to reproduce, multiple times, to modern reproducibility standards, every piece of research that informs governmental regulatory policy.
This should be done. But it is not within our means to do so.
What the authors of Shifting Sands did instead was to reframe the question more narrowly. Governmental regulation is meant to clear a high barrier of proof. Regulations should be based on a very large body of scientific research, the combined evidence of which provides sufficient certainty to justify reducing Americans’ liberty with a governmental regulation. What is at issue is not any particular piece of scientific research, but, rather, whether the entire body of research provides so great a degree of certainty as to justify regulation. If the government issues a regulation based on a body of research that has been affected by the irreproducibility crisis so as to create the false impression of collective certainty (or extremely high probability), then, yes, the irreproducibility crisis has affected government policy by providing a spurious level of certainty to a body of research that justifies a governmental regulation.
The justifiers of regulations based on flimsy or inadequate research often cite a version of what is known as the “precautionary principle.” This means that, rather than basing a regulation on science that has withstood rigorous tests of reproducibility, they base the regulation on the possibility that a scientific claim is accurate. They do this with the logic that it is too dangerous to wait for the actual validation of a hypothesis, and that a lower standard of reliability is necessary when dealing with matters that might involve severely adverse outcomes if no action is taken.
This report does not deal with the precautionary principle, since the principle summons a conclusiveness that lies beyond the realm of actual science. We note, however, that an invocation of the precautionary principle is not only non-scientific but is also an inducement to accept meretricious scientific practice, and even fraud.
The authors of Shifting Sands addressed the more narrowly framed question posed above. They applied a straightforward statistical test, Multiple Testing and Multiple Modeling (MTMM), and applied it to a body of meta-analyses used to justify government research. MTMM provides a simple way to assess whether any body of research has been affected by publication bias, p-hacking, and/or HARKing (Hypothesizing After the Results were Known)—central components of the irreproducibility crisis. In this third report, the authors applied this MTMM method to portions of the research underlying two aspects of nonpharmaceutical-intervention response to the COVID-19 pandemic that were formally or informally promoted by the Centers for Disease Control and Prevention (CDC): lockdowns and masking. Both these interventions were intended to reduce COVID-19 infections and fatalities, but the authors found persuasive circumstantial evidence that lockdowns and masking had no proven benefit to public health outcomes. Their technical studies suggest a far greater frailty (failure) in the system of epidemiological modeling and policy recommendations. That system, generally, grossly overestimated the potential effects of COVID-19 and, particularly, overestimated the potential benefit of lockdowns and masking. Their technical studies support recommendations for policy change to restructure the entire system of government policy based on epidemiological modeling, and not simply to apply cosmetic reforms to the existing system.
Confounded Errors broadens our critique of federal agencies from the Environmental Protection Agency (EPA) and the Food and Drug Administration (FDA) to include the CDC. More importantly, it highlights a whole new aspect of the irreproducibility crisis. The CDC and associated professions now rely heavily on a combination of epidemiology, statistics, and mathematical modeling. They do so to alter all sorts of individual and collective behavior, in the name of public health. This is alarming in itself, because public health agencies have taken it upon themselves to shift, for example, how people eat and whether or not to smoke. Of course, there are public health justifications—but this also allows the state and its servants to determine how citizens should live. Even with this relatively narrow scope, it is an astonishing expansion of state authority over individual lives.
Epidemiology already concerns itself with “surveillance” in the health context. It is reasonable to worry about the conflation of public health modeling and the parallel work by computer scientists to establish a broader surveillance state, to fear the marriage of the epidemiological model and the computer science algorithm. Meme transmission can be modeled; so can “public health” efforts to inhibit the reproduction of memes.
Put another way, Gelman and Loken’s “garden of forking paths” applies peculiarly to the world of modeling public health interventions. Gelman and Loken wrote of the world of statistical analysis that,
When we say an analysis was subject to multiple comparisons or “researcher degrees of freedom,” this does not require that the people who did the analysis were actively trying out different tests in a search for statistical significance. Rather, they can be doing an analysis which at each step is contingent on the data. The researcher degrees of freedom do not feel like degrees of freedom because, conditional on the data, each choice appears to be deterministic. But if we average over all possible data that could have occurred, we need to look at the entire garden of forking paths and recognize how each path can lead to statistical significance in its own way. Averaging over all paths is the fundamental principle underlying p-values and statistical significance and has an analogy in path diagrams developed by Feynman to express the indeterminacy in quantum physics.6
Researcher degrees of freedom apply to mathematical modeling. But modeling public health interventions translates these degrees of freedom from understanding the world to recommending policy; researcher degrees of freedom become intervention degrees of freedom, a phrase coined by the authors of Confounded Errors.
We may add to this the critique that modeling, by its nature, is intended to facilitate state action and, generally, forecloses serious consideration of the advantages of doing nothing.7 Modeling justifies state action; modeling relies on intervention degrees of freedom.
In my previous introductions I have written of the economic consequences of the irreproducibility crisis—of the costs, rising to the hundreds of billions annually, of scientifically unfounded federal regulations issued by the EPA and the FDA. I also have written about how activists within the regulatory complex piggyback upon politicized groupthink and false-positive results to create entire scientific subdisciplines and regulatory empires. The authors of Confounded Errors now bring into focus the deep connection between the irreproducibility crisis and the radical-activist state by their focus on intervention degrees of freedom. Americans have ceded governmental authority to professionals who claim the mantle of scientific authority—Jekylls who have imbibed too much of the potion of power and have become Hydes. The irreproducibility crisis in government is the intervention crisis. Intervention degrees of freedom mean the freedom of radical activists in federal bureaucracies to make policy, unrestrained by law, prudence, consideration of collateral damage, off-setting priorities, our elected representatives, or public opinion.
The use of the techniques of epidemiological modeling and computer algorithms to control public opinion—to remove any check to radical activist policy—is even more alarming. The silver lining is that our would-be Svengalis may fool themselves with their own false-positives and disseminate inefficient propaganda. But we cannot rely on their errors to check their malice.
We base our critique of COVID-19 public health policy on the narrow grounds of its relationship to the irreproducibility crisis and the intervention crisis. I am keenly aware that there are far more profound grounds with which to criticize COVID-19 public health policy. These criticisms levy charges of bad faith, politicized misconduct, and hysteria at much of our public health establishment, from Anthony Fauci on down. I certainly agree with the authors of Confounded Errors that the federal government should establish a commission to undertake a full-scale investigation and report on the origins and nature of COVID-19, as well as of public health policy errors committed during the response to COVID-19 by the CDC. Commission reports, of course, come and go. Such a report by itself, no matter how rigorously carried out, will mean little if it fails to attract widespread attention and to intensify public indignation at the misdeeds perpetrated in the name of “science.” Gaining the necessary level of attention will be hindered by the complicity of much of the national press and much of the science press in reinforcing the government’s false narratives.
What, then, is to be done? We must rely on the slowly crystallizing public recognition that the COVID-19 shutdown and many of the related measures taken in the name of public health were ill-founded. The regime of falsehoods cannot stand forever, and its collapse is already evident in the efforts of leaders such as Anthony Fauci, Francis Collins, and Rochelle Walensky to present exculpatory stories about their previous actions or simply to deny saying or doing what the record plainly shows. Nothing shows their vulnerability to serious, fact-based criticism more than their eagerness to flee from the positions they once touted as either impregnable scientific truths or the most promising precautionary measures given the uncertainties of the time.
The NAS also has written to oppose errors in COVID-19 public health policy as they apply to higher education.8 I do not judge it appropriate at this moment for the NAS to levy such charges—and I am glad that the authors of Confounded Errors have focused the report on scientists’ errors rather than scientists’ motives. But it certainly would be appropriate for Confounded Errors’ readers to use the evidence it presents to inform their broader judgment about the American public health establishment’s implementation of COVID-19 policy. Americans justly may wonder, and make informed conclusions, about whether such an extended period of scientific incompetence is accidental or intended.
Confounded Errors bolsters the case for policy reforms that would strengthen federal agencies’ procedures to assess research results, especially those grounded on statistical analyses—in environmental epidemiology, in nutritional epidemiology, in public health epidemiology and modeling, and in every government regulatory agency that justifies its actions with scientific or social-scientific research. It also justifies a new approach to science policy more generally. Americans must work to understand fully the connections between the irreproducibility crisis and the radical-activist state, to recognize the wide-ranging intervention crisis as a political problem of the first magnitude, and to draft policy solutions that will reassert the primacy of law, elected representatives, and the public over the arbitrary actions of the Lysenkos in government service who pretend to be Lavoisiers. We must reform not only the procedures of scientific research but also the procedures and powers of government expertise.
The National Association of Scholars, informed by Shifting Sands, will work on this larger problem. I hope we will have many colleagues to join us in this vital work.
Confounded Errors puts into layman’s language the results of several technical studies by members of the Shifting Sands team of researchers, S. Stanley Young and Warren Kindzierski. Some of these studies have been accepted by peer-reviewed journals; others have been submitted and are under review. As part of the NAS’s own institutional commitment to reproducibility, Young and Kindzierski pre-registered the methods of their technical studies. And, of course, the NAS’s support for these researchers explicitly guaranteed their scholarly autonomy and the expectation that these scholars would publish freely, according to the demands of data, scientific rigor, and conscience.
Confounded Errors is the third of four scheduled reports, each critiquing different aspects of the scientific foundations of federal regulatory policy. We intend to publish these reports separately and then as one long report, which will eliminate some necessary duplication in the material of each individual report. The NAS intends these four reports, collectively, to provide a substantive, wide-ranging answer to the question What has been the actual effect on government policy of irreproducible science?
I am deeply grateful for the support of many individuals who made Shifting Sands possible. The Arthur N. Rupe Foundation provided Shifting Sands’ funding—and, within the Rupe Foundation, Mark Henrie’s support and goodwill got this project off the ground and kept it flying. Two readers invested considerable time and thought to improve this report with their comments: William M. Briggs and Douglas W. Allen. David Acevedo copyedited Confounded Error with exemplary diligence and skill. David Randall, the NAS’s director of research, provided staff coordination for Shifting Sands—and, of course, Stanley Young has served as director of the Shifting Sands Project. Reports such as these rely on a multitude of individual, extraordinary talents.
Executive Summary
Scientists’ use of flawed statistics and editors’ complaisant practices both contribute to the mass production and publication of irreproducible research in a wide range of scientific disciplines. Far too many researchers use unsound scientific practices. This crisis poses serious questions for policymakers. How many federal regulations reflect irreproducible, flawed, and unsound research? How many grant dollars have funded irreproducible research? How widespread are research integrity violations? Most importantly, how many government regulations based on irreproducible science harm the common good?
The National Association of Scholars’ (NAS) project Shifting Sands: Unsound Science and Unsafe Regulation examines how irreproducible science negatively affects select areas of government policy and regulation governed by different federal agencies. We also seek to demonstrate procedures which can detect irreproducible research. This third policy paper in the Shifting Sands project focuses on failures by the U.S. Centers for Disease Control and Prevention (CDC) and the National Institutes of Health (NIH) to consider empirical evidence available in the public domain early in the COVID-19 pandemic.
The COVID-19 virus is not the plague or the Spanish flu. In effect, it is a very ordinary, new respiratory virus. It has a rather low case fatality rate. Over time it has become less lethal and more infectious, in line with viral evolutionary thinking. Historical wisdom for dealing with a new virus was to protect the weak and let natural immunity lead to herd immunity. Whereas COVID-19 infections were lethal primarily to elderly persons with comorbidities, the virus was sold to us by public health officials as a lethal danger to one and all.
Technical studies in our paper focused on two aspects of nonpharmaceutical intervention response to the COVID-19 pandemic: lockdowns and masking, which were both meant to reduce COVID-19 infections and fatalities. We used a novel statistical technique—p-value plotting—as a severe test to study specific claims made about the benefit to public health outcomes of these responses.
We found persuasive circumstantial evidence that lockdowns and masking had no proven benefit to public health outcomes. Our technical studies suggest a far greater frailty (failure) in the system of epidemiological modeling and policy recommendations. That system, generally, grossly overestimated the potential effects of COVID-19 and, particularly, overestimated the potential benefit of lockdowns and masking. We believe our technical studies support recommendations for policy change to restructure the entire system of government policy based on epidemiological modeling, and not simply to apply cosmetic reforms to the existing system.
We offer several recommendations to the CDC in particular, to government more generally, to the modeling profession, and to Americans as a whole about public health interventions.
Regarding civil liberty:
- Congress and the president should jointly convene an expert commission to set boundaries on the areas of private life which may be the subject of public health interventions.
- This commission’s rules should explicitly limit the scope of public health interventions to physical health, narrowly and carefully defined.
- All such public health interventions should be required to receive explicit sanction from both houses of Congress.
Regarding epidemiological (mathematical) modeling that forms a basis for CDC policymaking:
- Require pre-registration of mathematical modeling studies.
- Require mathematical modeling transparency and reproducibility.
- Formulate rules to reduce intervention degrees of freedom (see definition below) for modeling public health interventions to limit state action.
- Formulate guidelines that make explicit that modeling is meant to quantify the uncertainty of action, and that the CDC should convey to policymakers a quantification of the uncertainty of action rather than a prescription of certainty to justify action.
- The CDC should charter a commission to advise it in how to achieve these goals.
Regarding further commissions:
- The federal government should establish a commission to undertake a full-scale investigation and report on the origins and nature of COVID-19, as well as of public health policy errors committed during the response to COVID-19 by the CDC.
- As public health modeling naturally aligns with the use of computer science algorithms, social media censorship of heterodox, COVID-19-related posts depended on both. The federal government should establish a commission to provide guidelines for the federal funding, conduct, and regulation of the use of computer science algorithms, particularly as they are used by the federal government and by social media companies.
We have subjected the science underpinning the COVID-19 nonpharmaceutical interventions of lockdowns and masking to serious scrutiny. We believe the CDC should take account of our methods as it considers pandemic responses. Yet we care even more about reforming the procedures the CDC uses in general to assess pandemic responses.
The government should use the very best science—whatever the regulatory consequences. Scientists should use the very best research procedures—whatever result they find to assess pandemic responses. Those principles are the twin keynotes of this report. The very best science and research procedures involve building evidence on the solid rock of transparent, reproducible, and actually reproduced scientific inquiry, not on shifting sands.
Introduction
On March 11, 2020, the World Health Organization (WHO) officially declared COVID-19 a pandemic. In the next years, public health professionals largely formed policy responses to the pandemic. The response in most developed countries was a strategy of “suppression”9 or establishing rigorous “control regimes.”10 This strategy included various combinations of:
- widespread COVID-19 virus testing, contact tracing, and isolating;
- use of face masks in public;
- physical distancing;
- “lockdowns,” closed schools, and stay-at-home orders;
- limitations on mass gatherings; and
- improved ventilation systems at workplaces.
It also included the most extreme “zero-COVID” strategy (as it was known in China), which involved completely locking down the population.11
Public health professionals’ consensus strategy, crucially, assumed that COVID-19 itself was the great driver of mortality, that COVID-19 fatality rates were very high across a broad range of population subsets, and that these policies could substantially alter COVID-19 mortality. These three assumptions were used to justify the recommendation that as much of the general population as possible isolate itself in individual and family groups indefinitely, regardless of other costs.
We should note that these assumptions were based on the putative success of China in eradicating COVID-19 by means of a draconian lockdown regime, and, significantly, on mathematical modeling.12 Dr. Neil Ferguson of the Imperial College of London played a particularly important role by developing a pandemic mathematical model that projected that there would be hundreds of millions of deaths worldwide unless governments undertook such extreme protective actions.13 This reliance on mathematical modeling partly was an attempt to calculate proper policy in a timely fashion based on limited data about COVID-19 itself. Partly it was a consequence of modern scientific culture and institutions’ increasing dependence on highly complex and insufficient-quality mathematical models.
Sweden provided the most significant national departure from this strategy. Sweden also relied on public health professionals to determine its COVID-19 policy response, but these professionals, constrained by a constitution that did not allow for a state of emergency to be declared in peacetime,14 stuck to their own judgment rather than relying on an emerging quasi-consensus among their global peers. Sweden focused on protecting the most imperiled population sub-groups and allowed the population at large to interact freely and build up natural immunities.15 The Sweden strategy, we may note, essentially treated COVID-19 as a quasi-novel virus to which many had some prior immunity. Sweden persisted in its strategy despite substantial condemnation from the global public health establishment—condemnation which even extended to censorship of public justification of the strategy.16 Sweden ended up tied among Organisation for Economic Co-operation and Development countries for the lowest number of excess deaths from COVID-19.17
Within the United States, several states enacted policies that modified federal measures. Governor Ron DeSantis of Florida, notably, championed a strategy of protecting the most imperiled population sub-groups and preventing a continued total lockdown.18 As Sweden provided the most notable alternative on the world stage, Florida provided the most notable alternative strategy within the United States.
We now may conclude that Sweden and Florida enacted better public health policies than the world public health experts who relied on models such as Ferguson’s.
Implications of COVID-19 Suppression Strategies Used in the U.S.
Yet federal policy and CDC recommendations set the broad parameters for America’s COVID-19 public health policy. The suppression strategy that was enacted imposed severe costs on the American economy and society. What follows is a sampling of these costs, which were observed by November 2020:
- Between March 25 and April 10, 2020, nearly one-third of adults (31%) reported that their families could not pay the rent, mortgage, or utility bills; were food insecure; or went without medical care because of the cost.
- Q2 2020 GDP decreased at an annual rate of 32.9%, and Q1 2020 GDP decreased at an annual rate of 5%.
- Between March 25 and April 10, 2020, 41.5% of nonelderly adults reported having lost jobs, reduced work hours, or less income because of COVID-19.
- The unemployment rate increased to 14.7% in April 2020. This was the highest rate of increase (10.3%) and largest month-over-month increase in the history of available data (since 1948).
- In March, 39% of people with a household income of $40,000 and below reported a job loss.
- Mothers of children aged 12 and younger lost 2.2 million jobs between February and August (12% drop), while fathers of small children lost 870,000 jobs (4% drop).
- Preschool participation sharply fell from 71% pre-pandemic to 54% during the pandemic; the decline was steeper for young children in poverty.19
Similar consequences have been measured in later research.20 Such extraordinarily deleterious consequences require a very high public health justification—and the evidence that has emerged suggests that the assumptions used to justify the suppression-and-lockdown strategy were incorrect. More broadly, they suggest that the procedures of the public health establishment bear significant responsibility for their errors in judgment.
Reforming Government Regulatory Policy: The Shifting Sands Project
The National Association of Scholars’ (NAS) project Shifting Sands: Unsound Science and Unsafe Regulation examines how irreproducible science negatively affects select areas of government policy and regulation governed by different federal agencies.21 We also aim to demonstrate procedures which can detect irreproducible research. We believe government agencies should incorporate these procedures as they determine what constitutes “best available science”—the standard that judges which research should inform government regulation.22
In Shifting Sands we use an analysis strategy for all our policy papers⸺p-value plotting (a visual form of Multiple Testing and Multiple Modeling (MTMM) analysis)⸺as a way to demonstrate weaknesses in different agencies’ use of meta-analyses. MTMM corrects for statistical analysis strategies that produce a large number of false positive statistically significant results—and, since irreproducible results from base studies produce irreproducible meta-analyses, allows us to detect these irreproducible meta-analyses. (For a longer explanation of Multiple Testing Multiple Modeling and of statistical significance, see Appendixes 1 and 2.) Researchers doing epidemiological modeling studies should correct their work to take account of MTMM.23
Scientists generally are at least theoretically aware of this danger, albeit they have done far too little to correct their professional practices. Methods to adjust for MTMM have existed for decades. The Bonferroni method simply adjusts the p-value by multiplying the p-value by the number of tests. Westfall and Young provide a simulation-based method for correcting an analysis for MTMM.24
In practice, however, far too much “research” simply ignores the danger. Researchers can use MTMM until they find an exciting result to submit to the editors and referees of a professional journal—in other words, they can p-hack.25 Editors and referees have an incentive to trust, with too much complaisance, that researchers have done due statistical diligence, so they can publish exciting papers and have their journal recognized in the mass media.26 Some editors are part of the problem.27
The public health establishment’s practices are a component of the larger irreproducibility crisis, which has led to the mass production and publication of irreproducible research.28 Many improper scientific practices contribute to the irreproducibility crisis, including poor applied statistical methodology, bias in data reporting, publication bias (the skew toward publishing exciting, positive results), fitting the hypotheses to the data after looking at the data, and endemic groupthink.29 Far too many scientists use improper scientific practices, including an unfortunate portion who commit deliberate data falsification.30 The entire incentive structure of the modern complex of scientific research and regulation now promotes the mass production of irreproducible research.31 (For a longer discussion of the irreproducibility crisis, see Appendix 3.)
Many scientists themselves have lost overall confidence in the body of claims made in scientific literature.32 The ultimately arbitrary decision to declare p<0.05 as the standard of “statistical significance” has contributed extraordinarily to this crisis. Most cogently, Boos and Stefanski have shown that an initial result likely will not replicate at p<0.05 unless it possesses a p-value below 0.01, or even 0.001.33 Numerous other critiques about the p<0.05 problem have been published.34 Many scientists now advocate changing the definition of statistical significance to p<0.005.35 But even here, these authors assume only one statistical test and near-perfect study methods.
Researchers themselves have become increasingly skeptical of the reliability of claims made in contemporary published research.36 A 2016 survey found that 90% of surveyed researchers believed that research was subject to either a major (52%) or a minor (38%) crisis in reliability.37 Begley reported in Nature that 47 of 53 research results in experimental biology could not be replicated.38 A coalescing consensus of scientific professionals realizes that a large portion of “statistically significant” claims in scientific publications, perhaps even a majority in some disciplines, are false—and certainly should not be trusted until they are reproduced.39
Shifting Sands aims to demonstrate that the irreproducibility crisis has affected so broad a range of government regulation and policy that government agencies should now thoroughly modernize the procedures by which they judge “best available science.” Agency regulations should address all aspects of irreproducible research, including the inability to reproduce:
- the research processes of investigations;
- the results of investigations; and
- the interpretation of results.40
Our common approach supports a comparative analysis across different subject areas, while allowing for a focused examination of one dimension of the effect of the irreproducibility crisis on government agencies’ policies and regulations.
Keeping Count of Government Science: P-Value Plotting, P-Hacking, and PM2.5 Regulation focused on irreproducible research in environmental epidemiology that informs the Environmental Protection Agency’s policies and regulations.41
Keeping Count of Government Science: Flimsy Food Findings: Food Frequency Questionnaires, False Positives, and Fallacious Procedures in Nutritional Epidemiology focused on irreproducible research in nutritional epidemiology that informs much of the U.S. Food and Drug Administration’s nutrition policy.42
This third policy paper in the Shifting Sands project, Keeping Count of Government Science: The Confounded Errors of Public Health Policy Response to the COVID-19 Pandemic, focuses on failures by the U.S. Centers for Disease Control and Prevention (CDC) and the National Institutes of Health (NIH) to consider empirical evidence available in the public domain early in the pandemic.43 These mistakes eventually contributed to a public health policy that imposed substantial economic and social costs on the United States, with little or no public health benefit.
Confounded Error
Confounded Error provides an overview of the relevant history of the CDC and the NIH, of the history and character of the COVID-19 pandemic, and of the consequent public health policy response. We focus, however, on two aspects of COVID-19 and the related policy response:
- the effectiveness of lockdowns to reduce COVID-19 infections and fatalities; and
- the effectiveness of masking to reduce COVID-19 infections and fatalities.
We have applied Multiple Testing and Multiple Modeling analysis to both of these questions. P-value plots were used to independently assess the “reproducibility” of meta-analytical research claims made in literature for both cases (lockdowns, masks).
Informally, our report adopts Karl Popper’s empirical falsification approach, which underscores the importance to scientific theory of the falsification of hypotheses.44 CDC and NIH policy was predicated on the hypothesis that the United States’ suppression policy substantially benefited public health. We believe that our report provides substantial evidence, both collected from the existing literature and produced in our original research, to falsify this hypothesis.
In addition to presenting our research, other sections of this report include:
- discussion of our findings;
- our recommendations for policy changes; and
- appendixes.
Our policy recommendations include specific technical recommendations directly following from our technical analyses, with broader application for future federal regulatory pandemic policy response. They also include recommendations for a broader reform of the relation of professional expertise to policy formation.
COVID-19: Fumbling Forecasts and Ill-Planned Interventions
COVID-19 was an epidemic foretold. The 2002–2003 SARS epidemic presaged COVID-19 most closely, but, by 2019, epidemiologists had been engaged in contingency planning for a virulent outbreak of some sort for a generation—and in using modeling for several real disease outbreaks. The 9/11 terrorist attack, and the simultaneous use of anthrax as a bioweapon, made policymakers keenly aware of the need to plan for terrorist or state weaponization of infectious disease. The 2002–2003 SARS epidemic was followed by the possibility of an H5N1 influenza epidemic (2005), the H1N1 influenza pandemic (2009), the Ebola outbreak (2014–2016), and the Zika epidemic (2016–2017). The CDC and other epidemiologists used mathematical modeling throughout to estimate transmission, risks, and the effects of different public health interventions. Neil Ferguson’s work to model influenza directly influenced his later model for COVID-19.45
Forewarned, however, was not forearmed. Ferguson’s first COVID-19 model proved spectacularly misguided—and spectacularly influential, not least from the nightmare scenario it painted of COVID-19 response absent social distancing: “At one point, the [Ferguson] model projected over 2 million U.S. deaths by October 2020.” But even though models are supposed to be evaluated by their usefulness, scientists’ enthusiasm for Ferguson’s model was not dampened by its failure: “This model proved valuable not by showing us what is going to happen, but what might have been.”46 Even this encomium would appear to be misguided, since Ferguson’s model also predicted a nightmarishly high level of deaths, even with full lockdown policies enacted.
More precisely, Ferguson’s model failure, and the failures of other COVID-19 models, did not dampen enthusiasm among a large part of the professional community of epidemiological statisticians and modelers.47 This part of the professional community, which dominates the CDC and peer institutions, takes model failure to be a temporary shortcoming, data to be used to improve the next generation of models. Such professionals make carefully delimited suggestions for methodological reform: “It has been observed previously for other infectious diseases that an ensemble of forecasts from multiple models perform better than any individual contributing model.”48 They note the rationales for models whose simplicity led to profound policy errors, e.g., that modelers frequently prefer simple, parsimonious models, particularly to allow policy interventions to proceed quickly.49 Their retrospective on the history of COVID-19 modeling is one of bland, technocratic success:
In collaboration with academic, private sector, and US government modeling partners, the CDC rapidly built upon this modeling experience to support its coronavirus disease 2019 (COVID-19) response efforts. … The CDC Modeling Team collaborated with multiple academic groups to evaluate the potential impact of different reopening strategies in a simulated population. The evaluated strategies included: (1) closure throughout the 6-month prediction period; (2) reopening when cases decline below 5% of the peak daily caseload; (3) reopening 2 weeks after peak daily caseload; and (4) immediate reopening. This unique collaboration concluded that complete cessation of community spread of the disease was unlikely with any of these reopening strategies and that either additional stay-at-home orders or other interventions (eg, testing, contact tracing and isolation, wearing masks) would be needed to reduce transmission while allowing workplace reopening. This finding provided strong, timely evidence that control of the COVID-19 pandemic would require a balance of selected closure policies with other mitigation strategies to limit health impacts. The modeling results indicated that even moderate reductions in NPI [nonpharmaceutical intervention] adherence could undermine vaccination-related gains during the subsequent 2–3 months and that decreased NPI adherence, in combination with increased transmissibility of some SARS-CoV-2 variants, was projected to lead to surges in hospitalizations and deaths. These findings reinforced the need for continued public health messaging to encourage vaccination and the effective use of NPIs to prevent future increases in COVID-19.50
The policies that these researchers so blandly endorsed, meanwhile, were astonishingly and troublingly open-ended. In April 2020, for example, the WHO recommended that governments continue lockdowns until such time as they could achieve a set of six conditions alternately arbitrary or implausibly rigorous.
- Disease transmission is under control
- Health systems are able to “detect, test, isolate and treat every case and trace every contact”
- Hot spot risks are minimized in vulnerable places, such as nursing homes
- Schools, workplaces and other essential places have established preventive measures
- The risk of importing new cases “can be managed”
- Communities are fully educated, engaged and empowered to live under a new normal51
The last of these conditions left undefined “a new normal,” but it would seem to imply that governments should continue lockdowns until such time as the citizenry’s “fully educated” views and behavior coincided in all respects with the recommendations of public health experts. A technical model submitted to the public for judgment should not have the alteration of the public’s judgment as a component—much less hold the public hostage to continued lockdowns until they assent to supporting the lockdown policies.
Another part of the professional community has highlighted COVID-19 models’ methodological flaws, and their basic failure to predict events—presumably a sine qua non in a model.52 Collins and Wilkinson conducted a systematic review of 145 COVID-19 prediction models published or preprinted between January 3 and May 5, 2020, and discovered pervasive statistical flaws: different models suffered from small sample size, many predictors, arbitrarily discarded data and predictors, overfitted models, and a general lack of transparency about how they were created. These flaws frequently overlapped. In sum, “all models to date, with no exception, are at high risk of bias with concerns related to data quality, flaws in the statistical analysis, and poor reporting, and none are recommended for use.”53 Nixon et al. likewise stated of a sample of 136 papers that
a large fraction of papers did not evaluate performance (25%), express uncertainty (50%), or state limitations (36%). … Papers did not consistently state the precise objective of their model (unconditional forecast or assumption-based projection), detail their methodology, express uncertainty, evaluate performance across a long, varied timespan, and clearly list their limitations.54
Ioannidis et al. provided a scathing cumulative judgment:
Epidemic forecasting has a dubious track-record, and its failures became more prominent with COVID-19. Poor data input, wrong modeling assumptions, high sensitivity of estimates, lack of incorporation of epidemiological features, poor past evidence on effects of available interventions, lack of transparency, errors, lack of determinacy, consideration of only one or a few dimensions of the problem at hand, lack of expertise in crucial disciplines, groupthink and bandwagon effects, and selective reporting are some of the causes of these failures. … Even for short-term forecasting when the epidemic wave waned, models presented confusingly diverse predictions with huge uncertainty.55
Ioannidis et al. added to this judgment a larger critique of previous epidemiological modeling: “Predictions may work in ‘ideal’, isolated communities with homogeneous populations, not the complex current global world.”56
Ioannidis et al. address the argument that we need to consider the possibility of ‘doomsday pandemics’ with the sensible observation that we need to be sure that doomsday actually has arrived—and that we have tools available to help us make that assessment judiciously: “Upon acquiring solid evidence about the epidemiological features of new outbreaks, implausible, exaggerated forecasts should be abandoned. Otherwise, they may cause more harm than the virus itself.”57 They concluded with a catalogue of recommendations to modelers that constitute a devastating critique of standard operating practices among epidemiological modelers.
- Invest more on collecting, cleaning, and curating real, unbiased data, and not just theoretical speculations
- Model the entire predictive distribution, with particular focus on accurately quantifying uncertainty
- Continuously monitor the performance of any model against real data and either re-adjust or discard models based on accruing evidence
- Avoid unrealistic assumptions about the benefits of interventions; do not hide model failure behind implausible intervention effects
- Use up-to-date and well-vetted tools and processes that minimize the potential for error through auditing loops in the software and code
- Maintain an open-minded approach and acknowledge that most forecasting is exploratory, subjective, and non-pre-registered research
- Beware of unavoidable selective reporting bias58
Ioannidis, who has articulated his skepticism of many aspects of institutional COVID-19 research, and corollary policy, by appearing as an author in a very large body of scientific literature,59 is the foremost figure in the study of irreproducible research, and more generally of metaresearch, the study of scientific research’s “methods, reporting, reproducibility, evaluation, and incentives.”60 If Ioannidis says that epidemiological models are an irreproducible mess, and if thousands of epidemiologists assure the public that their models are excellent, a prudent man would give Ioannidis’ word greater weight.
The public ought to be able to do more than simply take the word of one scientist or another. Unfortunately, the very complexity of models makes it extraordinarily difficult to provide a standard by which to hold them accountable—aside from the common-sense standard, did they predict well? Then, too, while models are considered sufficiently solid to inform policy immediately, they are tentative enough in their claims that a disproven model can always be disclaimed with a shrug and a reply that we updated the data. The failure of one parameter informs a new parameterization, not a skepticism of parameters in general. The failure of one prediction can be ignored with resort to the general and the counterfactual: if you hadn’t followed our advice generally, millions would be dead. To say that a model failed is to invite the inevitable riposte, we’re doing it better now.
We focus our critique on two particular areas that speak to the accuracy of COVID-19 modeling: masks and lockdowns. We focus on these partly for their intrinsic importance.
- Lockdowns of the entire population were the most rigorous nonpharmaceutical intervention (NPI) response to the COVID-19 pandemic. Such lockdowns always were deeply controversial, and while China claimed to have successfully ended COVID-19 by means of particularly draconian COVID-19 policies, Sweden, Florida, and other entities rejected them in part or in whole.61 A substantial amount of political debate about COVID-19 policy turns upon the justification of lockdowns, and their efficacy.
- Masks, meanwhile, became a highly visual “condensed” symbol of the entire COVID-19 policy regime.62
We chose these two metrics in particular because Ioannidis published a critique of COVID-19 modeling on March 19, 2020, that focused on the effects of both lockdowns and masks: “Maintaining lockdowns for many months may have even worse consequences than an epidemic wave that runs an acute course. … randomized trials should evaluate also the real-world effectiveness of simple measures (eg face masks in different settings).”63 Ioannidis’ contemporary critique further justifies a retrospective critique of these aspects of COVID-19 modeling.
Technical Studies: Methods
Our technical studies include their own methods sections, written for a professional audience. We provide this methods section for a lay audience.
P-value Plots
Epidemiologists traditionally use confidence intervals instead of p-values from a hypothesis test to demonstrate or interpret statistical significance. Since researchers construct both confidence intervals and p-values from the same data, the one can be calculated from the other.64 We then develop p-value plots, a method for correcting Multiple Testing and Multiple Modeling (MTMM), to inspect the distribution of the set of p-values.65 (For a longer discussion of p-value plots, see Appendix 4.) The p-value is a random variable derived from a distribution of the test statistic used to analyze data and to test a null hypothesis.66 In a well-designed study, the p-value is distributed uniformly over the interval 0 to 1 regardless of sample size under the null hypothesis, and the distribution of true null hypothesis points in a p-value plot should form a straight line.67
A plot of p-values corresponding to a true null hypothesis, when sorted and plotted against their ranks, should conform to a near 45-degree line. Researchers can use the plot to assess the reliability of base study papers used in meta-analyses. (For a longer discussion of meta-analyses, see Appendix 5.)
We construct and interpret p-value plots as follows:
- We compute and order p-values from smallest to largest and plot them against the integers, 1, 2, 3, …
- If the points on the plot follow an approximate 45-degree line, we conclude that the p-values resulted from a random (chance) process, and that the data therefore supported the null hypothesis of no significant association.68
- If the points on the plot follow approximately a line with a flat/shallow slope, where most of the p-values were small (less than 0.05), then the p-values provide evidence for a real (statistically significant) association.
- If the points on the plot exhibit a bilinear shape (divided into two lines), then the p-values used for meta-analysis are consistent with a two-component mixture and a general (overall) claim is not supported; in addition, the p-value reported for the overall claim in the meta-analysis paper cannot be taken as valid.69
The formal meta-analysis process is strictly analytic. It computes an overall statistic for those test statistics combined, whereupon a research claim is made from the overall statistic. The meta-analysis computational method is flawed, given that, as Nelson et al. (2018) state, “if there is some garbage in, then there is only garbage out.”70 P-value plotting is an independent method to assess heterogeneity of the test statistics combined in meta-analysis to examine whether garbage is present.
P-value plotting is not by itself a cure-all. P-value plotting cannot detect every form of systematic error. P-hacking, research integrity violations, and publication bias will alter a p-value plot. But it is a useful tool which allows us to detect a strong likelihood that questionable research procedures, such as HARKing (see below) and p-hacking, have corrupted base studies used in meta-analysis and, therefore, have rendered the meta-analysis unreliable. We may also use it to plot “missing papers” in a body of research, and thus to infer that publication bias has affected a body of literature.
We may also use p-value plotting to plot “missing papers” in a body of research, and thus to infer that publication bias has affected a body of literature.
To HARK is to hypothesize after the results are known—to look at the data first and then come up with a hypothesis that has a statistically significant result.71 (For a longer discussion of HARKing, see Appendix 6.)
P-hacking involves the relentless search for statistical significance and comes in many forms, including MTMM without appropriate statistical correction.72
Irreproducible research hypotheses produced by HARKing and p-hacking send whole disciplines chasing down rabbit holes. This allows scientists to pretend their “follow-up” research is confirmatory research; but in reality, their research produces nothing more than another highly tentative piece of exploratory research.73
P-value plotting is not the only means available by which to detect questionable research procedures. Scientists have come up with a broad variety of statistical tests to account for frailties in base studies as they compute meta-analyses. Unfortunately, questionable research procedures in base studies severely degrade the utility of the existing means of detection.74 We proffer p-value plotting not as the first means to detect HARKing and p-hacking in meta-analysis, but as a better means than alternatives which have proven ineffective.
We proffer p-value plotting not as the first means to detect HARKing and p-hacking in meta-analysis, but as a better means than alternatives which have proven ineffective.
Public Health Interventions: Lockdowns
1. Introduction
On March 11, 2020, the WHO officially declared COVID-19 a pandemic.75 Many governments subsequently adopted aggressive pandemic policies.76 Examples of these policies, imposed as large-scale restrictions on people, included: quarantine (stay-at-home) orders; masking orders in community settings; nighttime curfews; closures of schools, universities, and many businesses; and bans on large gatherings.77
The objective of this study was to use a p-value plotting statistical method (after Schweder & Spjøtvoll) to independently evaluate specific research claims related to COVID-19 quarantine (stay-at-home) orders in published meta-analysis studies.78 This was done in an effort to illustrate the importance of the reproducibility of research claims arising from this nonpharmaceutical intervention in the context of the surge of COVID-19 papers in literature over the past few years.
2. Method
We first wanted to gauge the number of reports of meta-analysis studies cited in literature related to some aspect of COVID-19. To do this we again used the Advanced Search Builder capabilities of the PubMed search engine.79 Our search returned 3,204 listings in the National Library of Medicine database. This included 633 listings for 2020, 1,301 listings for 2021, and 1,270 listings thus far for 2022. We find these counts astonishing, in that a meta-analysis is a summary of available papers.
Given our understanding of the pre-COVID-19 research reproducibility of published literature discussed above, we speculated that there may be numerous meta-analysis studies relating to COVID-19 that are irreproducible. We prepared and posted a research plan on the Researchers.One platform.80 This plan can be accessed and downloaded without restrictions from the platform. Our plan was to use p-value plotting to independently evaluate four selected published meta-analysis studies specifically relating to possible health outcomes of COVID-19 quarantine (stay-at-home) orders—also referred to as ‘lockdowns’ or ‘shelter-in-place’ in literature.
2.1 Data sets
As stated in our research plan,81 we considered four meta-analysis studies in our evaluation:
- Herby et al. (2022) – mortality82
- Prati & Mancini (2021) – psychological effects (specifically, mental health symptoms)83
- Piquero et al. (2021) – reported incidents of domestic violence84
- Zhu et al. (2022) – suicidal ideation (thoughts of killing yourself)85
We downloaded and read electronic copies of each meta-analysis study (and any corresponding electronic supplementary information files).
Herby et al. (2022)86 – The Herby et al. (2022) meta-analysis examined the effect of COVID-19 quarantine (stay-at-home) orders implemented in 2020 on mortality based on available empirical evidence. These orders were defined as the imposition of at least one compulsory, non-pharmaceutical intervention. Herby et al. initially identified 19,646 records that could potentially address their purpose.
After three levels of screening by Herby et al., 32 studies qualified. Of these, estimates from 22 studies could be converted to standardized measures for inclusion in their meta-analysis. For our evaluation, we could only consider results for 20 of the 22 studies (data they provided for two studies could not be converted to p-values). Their research claim was: “lockdowns in the spring of 2020 had little to no effect on COVID-19 mortality.”
Prati & Mancini (2021)87 – The Prati & Mancini (2021) meta-analysis examined the psychological effects of COVID-19 quarantine (stay-at-home) orders on the general population. These included: mental health symptoms (such as anxiety and depression), positive psychological functioning (such as well-being and life-satisfaction), and feelings of loneliness and social support as ancillary outcomes.
Prati & Mancini initially identified 1,248 separate records that could potentially address their purpose. After screening, they identified and assessed 63 studies for eligibility and ultimately considered 25 studies for their meta-analysis. For our evaluation, we used all 20 results they reported on for mental health symptoms. Their research claim was: “lockdowns do not have uniformly detrimental effects on mental health and most people are psychologically resilient to their effects.”
Piquero et al. (2021)88 – The Piquero et al. (2021) meta-analysis examined the effect of COVID-19 quarantine (stay-at-home) orders on reported incidents of domestic violence. They used the following search terms to identify suitable papers with quantitative data to include in their meta-analysis: “domestic violence,” “intimate partner violence,” or “violence against women.”
Piquero et al. initially identified 22,557 records that could potentially address their purpose. After screening, they assessed 132 studies for eligibility and ultimately considered 18 studies in their meta-analysis. For our evaluation, we used all 17 results (effect sizes) that they presented from the 18 studies. Their research claim was: “incidents of domestic violence increased in response to stay-at-home/lockdown orders.”
Zhu et al. (2021)89 – The Zhu et al. (2021) meta-analysis examined the effect of COVID-19 quarantine (stay-at-home) orders on suicidal ideation and suicide attempts among psychiatric patients in any setting (e.g., home, institution, etc.). They used the following search terms to identify suitable papers with quantitative data to include in their meta-analysis: “suicide,” “suicide attempt,” “suicidal ideation,” or “self-harm”; “psychiatric patients,” “psychiatric illness,” “mental disorders,” “psychiatric hospitalization,” “psychiatric department,” “depressive symptoms,” or “obsessive-compulsive disorder.”
Zhu et al. initially identified 728 records that could potentially address their purpose. After screening, they assessed 83 studies for eligibility and ultimately considered 21 studies in their meta-analysis. For our evaluation, we used all 12 results that they reported on for suicidal ideation among psychiatric patients. Their research claim was: “estimated prevalence of suicidal ideation within 12 months [during COVID] was … significantly higher than a world Mental Health Survey conducted by the World Health Organization (WHO) in 21 countries [conducted 2001−2007].”
2.2 P-value plots
Epidemiologists traditionally use risk ratios and confidence intervals instead of p-values from a hypothesis test to demonstrate or interpret statistical significance. Altman & Bland show that both confidence intervals and p-values are constructed from the same data, that they are inter-changeable, and that one can be calculated from the other.90
Using JMP statistical software (SAS Institute, Cary, NC), we estimated p-values from risk ratios and confidence intervals for all data in each of the meta-analysis studies. In the Herby et al. meta-analysis, standard error (SE) was presented instead of confidence intervals. Where SE values were not reported, we used the median SE of the other base studies used in the meta-analysis (6.8). The p-values for each meta-analysis are summarized in an Excel file (.xlsx format) that can be downloaded at our posted Researchers.One research plan.91
We then created p-value plots after Schweder & Spjøtvoll to inspect the distribution of the set of p-values for each meta-analysis study.92
3. Results
3.1 Mortality
Our independent evaluation of the effect of COVID-19 quarantine (stay-at-home) orders on mortality—the Herby et al. (2022) meta-analysis—is shown in Figure 1. There are 20 studies that we included in the figure. Six of the 20 studies had p-values below 0.05, while four of the studies had p-values close to 1.00. Ten studies fell roughly on a 45-degree line, implying random results.
This data set comprises mostly null associations (14), as well as five or six possible non-null associations (1-in-20 of these could be a chance, i.e., false positive, association). While not perfect, this data set is a closer fit to a sample distribution for a true null association between two variables. Our interpretation of the p-value plot is that COVID-19 quarantine (stay-at-home) orders are not supported for reducing mortality, consistent with Herby et al.’s claim.
3.2 Psychological effects (mental health symptoms)
Our independent evaluation of the effect of COVID-19 quarantine (stay-at-home) orders on mental health symptoms—the Prati & Mancini (2021) meta-analysis—is shown in Figure 2. Figure 2 data present as a bilinear shape showing a two-component mixture. This data set clearly does not represent a distinct sample distribution for either true null associations or true effects between two variables. Our interpretation of the p-value plot is that COVID-19 quarantine (stay-at-home) orders have an ambiguous (uncertain) effect on mental health symptoms. However, as discussed below, there are questions about their research claim.
Figure 1. P-value plot (p-value versus rank) for Herby et al. (2022) meta-analysis of the effect of COVID-19 quarantine (stay-at-home) orders implemented in 2020 on mortality. Symbols (circles) are p-values ordered from smallest to largest (n=20).
Figure 2. P-value plot (p-value versus rank) for Prati & Mancini (2021) meta-analysis of the effect of COVID-19 quarantine (stay-at-home) orders on mental health symptoms. Symbols (circles) are p-values ordered from smallest to largest (n=20).
3.3 Incidents of domestic violence
Our independent evaluation of the effect of COVID-19 quarantine (stay-at-home) orders on reported incidents of domestic violence—the Piquero et al. (2021) meta-analysis—is shown in Figure 3. Thirteen of the 17 studies had p-values less than 0.05. While not shown in the figure, eight of the p-values were small (<0.001).
This data set comprises mostly non-null associations (13), as well as four possible null associations. While not perfect, this data set is a closer fit to a sample distribution for a true alternative association between two variables. Our interpretation of the p-value plot is that COVID-19 quarantine (stay-at-home) orders have a negative effect (increase) in reported incidents of domestic violence.
Figure 3. P-value plot (p-value versus rank) for Piquero et al. (2021) meta-analysis of the effect of COVID-19 quarantine (stay-at-home) orders on reported incidents of domestic violence. Symbols (circles) are p-values ordered from smallest to largest (n=17).
3.4 Suicidal ideation
Our independent evaluation of the effect of COVID-19 quarantine (stay-at-home) orders on suicidal ideation—the Zhu et al. (2021) meta-analysis—is shown in Figure 4. The p-values for all 12 studies were less than 0.05. Ten of the 12 studies had p-values less than 0.005. While not shown in the figure, eight of the p-values were small (<0.001).
This data set presents as a distinct sample distribution for true effects between two variables. Our interpretation of the p-value plot is that COVID-19 quarantine (stay-at-home) orders have an effect on suicidal ideation (thoughts of killing yourself). However, as discussed below, there are valid questions about how the meta-analysis was formulated.
Figure 4. P-value plot (p-value versus rank) for Zhu et al. (2021) meta-analysis of the effect of COVID-19 quarantine (stay-at-home) orders on suicidal ideation (thoughts of killing yourself). Symbols (circles) are p-values ordered from smallest to largest (n=12).
4. Discussion and Implications
As stated previously, an independent evaluation of published meta-analyses on a common research question can be used to assess the reproducibility of a claim coming from that field of research. We evaluated four meta-analysis studies of COVID-19 quarantine (stay-at-home) orders implemented in 2020 and corresponding health benefits and/or harms. Our intent was to illustrate the importance of reproducibility of research claims arising from this nonpharmaceutical intervention in the context of the surge of COVID-19 papers in literature over the past few years.
4.1 Mortality
The Herby et al. meta-analysis examined the effect of COVID-19 quarantine orders on mortality. Their research claim was: “lockdowns in the spring of 2020 had little to no effect on COVID-19 mortality.” Here, they imply that the intervention (COVID-19 quarantine orders) had little or no effect on the reduction of mortality. To put their findings into perspective, Herby et al. estimated that the average lockdown in the United States (Europe) in the spring of 2020 avoided 16,000 (23,000) deaths. In contrast, they report that there are about 38,000 (72,000) flu deaths each year in the United States (Europe).93
Our evidence agrees with their claim. Our p-value plot (Figure 1) is not consistent with the expected behavior of a distinct sample distribution for a true effect between the intervention (quarantine) and the outcome (reduction in mortality). More importantly, our plot shows considerable randomness (many null associations, p-values > 0.05), supporting no consistent effect. Herby et al. further stated: “costs to society must be compared to the benefits of lockdowns, which our meta-analysis has shown are little to none.”
4.2 Psychological effects (mental health symptoms)
The Prati & Mancini meta-analysis examined the psychological effects of COVID-19 quarantine orders on the general population. Their research claim was: “lockdowns do not have uniformly detrimental effects on mental health and most people are psychologically resilient to their effects.” We evaluated a component of psychological effects—i.e., whether COVID-19 quarantine orders affect mental health symptoms (Figure 2). Figure 2 clearly exhibits a two-component mixture, implying an ambiguous (uncertain) effect on mental health symptoms. However, our evidence does not necessarily support their claim.94
Digging deep into their study reveals an interesting finding. Their study looked at a variety of psychological symptoms that differed from study to study. Although not shown here, when they examined these symptoms separately—a meta-analysis of each symptom—there was a strong signal for anxiety (p-value less than 0.0001). This is less than the Boos & Stefanski–proposed p-value action level of 0.001 for expected replicability.95 Here, the term ‘action level’ means that if a study is replicated, the replication will give a p-value less than 0.05. We note with interest that, at the height of the pandemic, news coverage of COVID-19 was constantly saying you could die of the virus. It should be no wonder that there was a strong signal for anxiety.
We also note that Prati & Mancini appear to take the absence of evidence of a negative mental health effect of COVID-19 quarantine orders in their meta-analysis as implying that it does not affect mental health. But “absence of evidence does not imply evidence of absence.”96 Just because meta-analysis failed to find an effect, it does not imply that “most people are psychologically resilient to their [lockdowns’] effects.” A more plausible and valid inference is that this statement of claim is insufficiently researched at this point.
4.3 Incidents of domestic violence
The Piquero et al. meta-analysis examined the effect of COVID-19 quarantine orders on reported incidents of domestic violence. Their research claim was: “incidents of domestic violence increased in response to stay-at-home/lockdown orders.” Our evidence suggests agreement with this claim. Our p-value plot (Figure 3) is more consistent with the expected behavior of a distinct sample distribution for a true effect between the intervention (quarantine) and the outcome (increase in incidents of domestic violence).97
We note that Figure 3 has 13 of 17 p-values less than 0.05, with eight of these less than 0.001, and only a few null association studies (4). Our evidence supports that COVID-19 quarantine orders likely increased incidents of domestic violence.
4.4 Suicidal ideation
The Zhu et al. meta-analysis examined COVID-19 quarantine orders on suicidal ideation (thoughts of killing yourself). Their research claim was: “estimated prevalence of suicidal ideation within 12 months [during COVID] was … significantly higher than a world Mental Health Survey conducted by the World Health Organization (WHO) in 21 countries [conducted 2001−2007].”98
The p-value plot (Figure 4) strongly supports their claim. The plot is very consistent with the expected behavior of a distinct sample distribution for a true effect between the intervention (quarantine) and the outcome (increased prevalence of suicidal ideation). However, digging deep into their study reveals a problem in the formulation of their meta-analysis.
In strong science, a research question is measured against a control. Zhu et al. effectively ignore controls in their meta-analysis. They compared the incidence of suicidal ideation to a zero standard and not to control groups. The issue is that a pre-COVID-19 (i.e., background) suicidal ideation signal is ignored in their meta-analysis.
Indeed, in their Table 1 they present results from the base papers where data for control groups is available. For example, the Seifert et al. (2021) base paper notes suicidal ideation presented in 123 of 374 patients in the psychiatric emergency department of Hannover Medical School during the pandemic, and in 141 of 476 in the same department before the pandemic—32.9% versus 29.6%. The difference is not significant.99
Comparing their Table 1 data set with their Figure 1 forest plot, Zhu et al. only carried 32.9% into their meta-analysis for the Seifert et al. (2021) base paper; in effect, they ignored the control data. All data-set entries in their Figure 1 suffer from this problem. Zhu et al. only considered pandemic incidence in their meta-analysis; they ignored any control data. This approach calls their claims into serious question. We conclude that the Zhu et al. results are unreliable.
4.5 Implications
COVID-19 quarantine orders were implemented on the notion that this nonpharmaceutical intervention would delay and flatten the epidemic peak and benefit public health outcomes overall. P-value plots for three of four meta-analyses that we evaluated do not support public health benefits of this form of nonpharmaceutical intervention. The fourth meta-analysis study is unreliable.
One meta-analysis that we evaluated—Herby et al. (2022)—questions the benefits of this form of intervention for preventing mortality. Our p-value plot supports their finding that COVID-19 quarantine orders had little or no effect on the reduction of mortality.
A second meta-analysis—Prati & Mancini (2021)—offers conflicting evidence. Our p-value plot clearly exhibits a two-component mixture implying an ambiguous (uncertain) effect between COVID-19 quarantine orders and mental health symptoms. However, data for a component of mental health symptoms (anxiety) suggests a negative effect from COVID-19 quarantine orders. Further, Prati & Mancini (2021) lack evidence to claim that “most people are psychologically resilient to their [lockdowns’] effects.”
Our evaluation of the Piquero et al. (2021) meta-analysis—assessment of domestic violence incidents—supports a true effect between the intervention (quarantine) and the outcome (increase in incidents of domestic violence), with additional confirmatory research needed. Finally, the meta-analysis of Zhu et al. (2021) on suicidal ideation (thoughts of killing yourself) is wrongly formulated. Their results should be disregarded until or unless controls are properly included in their analysis.
Stepping back and looking at the overall findings of these studies, the claim that COVID-19 quarantine orders reduce mortality is unproven.
Also, the risks (negative public health consequences) of this intervention cannot be ruled out for mental health symptoms and incidents of domestic violence. Given that the base studies and the meta-analyses themselves were, for the most part, rapidly conducted and published, we acknowledge that confirmatory research for some of these outcomes is needed.
Our interpretations of COVID-19 quarantine benefits/risks are consistent, for example, with the research of James (2020) and conventional wisdom on disease mitigation measures used for the control of pandemic influenza.100 James holds that is it unclear whether there were benefits from this intervention relative to less restrictive measures aimed at controlling “risky” personal interactions (e.g., mass gatherings and large clusters of individuals in enclosed spaces).
James (2020) also noted numerous economic and public health harms in the United States as of May 1, 2020:
- Over 20 million people newly unemployed.
- State-wide school closures across the country.
- Increased spouse and child abuse reports.
- Increased divorces.
- Increased backlog of patient needs for mental health services, cancer treatments, dialysis treatments, and everyday visits for routine care.
- Increased acute emergency services.101
This is consistent with interim quantitative data as of September 2020 presented by the American Institute of Economic Research (2020) on the cost and negative public health implications of pandemic restrictions in the United States and around the world.102
Public Health Interventions: Masks
1. Introduction and Background
The World Health Organization (WHO) declared COVID-19 a pandemic on March 11, 2020.103 Early in the pandemic, the U.S. Centers for Disease Control and Prevention (CDC) recommended that patients in health-care settings under investigation for symptoms of suspected COVID-19 infection should wear a medical mask as soon as they were identified.104 On April 30, 2020, the CDC recommended that all people wear a mask outside of their home.105
This recommendation came about after emerging data reported transmission of the COVID-19 virus from persons without symptoms and after recognition that COVID-19 could spread by airborne transmission. Although Balazy et al. and Inglesby et al. had reported in 2006 that even medical masks do little to prevent the inhalation of small droplets bearing influenza virus,106 the CDC recommended using cloth face coverings that could be made more widely available in the community than medical masks, and which would allow public health authorities to allocate personal protective equipment such as medical masks and N95 respirators to the highest-risk health-care settings.107
Given the potentially large data sets available to medical researchers today, intervention−health outcome studies require a strong statistical component to establish informative and interpretable intervention−risk/benefit associations and research claims made from these associations. A statistical approach (p-value plotting after Schweder & Spjøtvoll (1982)108) was used in a study of medical (surgical) masks to evaluate the reproducibility of meta-analysis research claims related to the benefit of mask use in community settings to prevent COVID-19 infection.
Mask study background
Background characteristics of respiratory virus airborne transmission are presented in Section 7.1 of Appendix 7. Details about the selection of meta-analysis studies and methods are presented in Section 7.2 of Appendix 7. Briefly, we searched scientific literature to identify meta-analysis studies of randomized controlled trials (RCTs) examining medical-mask use in the community for the prevention of influenza and COVID-19 virus infections. We searched two databases: The Cochrane Central Register of Controlled Trials (CENTRAL) and PubMed.
Outcomes of medical diagnosis of viral illness and lab-confirmed diagnosis of viral illness were of interest. Data from RCTs based on self-reported symptoms of viral illness were excluded because of awareness bias (we provide further explanation in Section 7.2 of Appendix 7).
Epidemiologists traditionally use risk ratios or odds ratios and confidence intervals instead of p-values from a hypothesis test to demonstrate or interpret statistical significance. Both confidence intervals and p-values are constructed from the same data, and they are interchangeable. Altman and Bland provide formulae showing how one can be calculated from the other.109 Standard statistical software packages—such as SAS and JMP (SAS Institute, Cary, NC) or STATA (StataCorp LLC, College Station, TX)—can also be used to estimate p-values from risk ratios or odds ratios and confidence intervals.
We estimated p-values using JMP statistical software from risk ratios or odds ratios and confidence intervals for all data in each of the eligible meta-analysis studies evaluated. We then developed p-value plots to inspect the distribution of the set of p-values for each meta-analysis study.
2. Results
2.1 Search results
CENTRAL – Using search procedures described in Section 7.3 of Appendix 7, we identified 61 Cochrane Reviews published from January 1, 2020, to December 7, 2022. These are listed in Section 7.3 of Appendix 7. After examining full abstracts for these reviews online, we found one eligible meta-analysis study that met the search criteria: Jefferson et al. (2021).110
PubMed (medical research literature) – Also, using search procedures described in Section 7.3 of Appendix 7, we identified 73 records published for the period. These are listed in Section 7.3 of Appendix 7. After examining full abstracts for these studies online, we found six eligible meta-analysis studies that met the search criteria: Aggarwal et al. (2020), Xiao et al. (2020), Nanda et al. (2021), Tran et al. (2021a), Kim et al. (2022), and Ollila et al. (2022).111 Coincidentally, the Xiao et al. (2020) meta-analysis used the exact same RCT data as an earlier World Health Organization study.112
Gray literature – A final study included from gray literature was Liu et al. (2021), a systematic review by the CATO Institute, a public-policy research organization.113 In total, we evaluated seven meta-analyses and one systematic review using p-value plots.
2.2 P-value plots
We describe the characteristics of all eight studies evaluated in Section 7.4 of Appendix 7. This information includes the following for each study: the databases searched, the details about viral-illness outcomes reported, tables of outcome measures (risk ratio and 95% confidence intervals) and estimated p-values, and other unique evidence and/or limitations worth noting.
We constructed and presented p-value plots below for six meta-analyses: Jefferson et al. (2020) (Figure 5a), Xiao et al. (2020) (Figure 5b), Nanda et al. (2021) (Figure 6a), Tran et al. (2021a) (Figure 6b), Kim et al. (2022) (Figure 7a), and Liu et al. (2021) (Figure 7b). We did not construct p-value plots for two meta-analyses—Aggarwal et al. (2020) and Ollila et al. (2022)—because of their over-reliance on self-reported outcomes and/or irregularities or biases (refer to Section 7.4 of Appendix 7 for further details).
2.2.1 Cochrane literature review
Jefferson et al. (2020)114 – The authors used fifteen community (non–healthcare worker) RCTs—base studies—comparing medical masks to no masks in this meta-analysis (Appendix 7, Table 7.4.1). Their research claim—i.e., cause−effect scientific claim—was (Authors’ conclusions, p. 3): “pooled results of randomised trials did not show a clear reduction in respiratory viral infection with the use of medical/surgical masks during seasonal influenza.” We present the p-value plot for this study in Figure 5a.
Figure 5. Meta-analysis p-value plots: (a) 15 RCT base studies (Jefferson et al. 2020), (b) 7 RCT base studies (Xiao et al. 2020)
2.2.2 Medical research literature
Aggarwal et al. (2020)115 – The authors used five cluster-RCT base studies comparing medical masks to no masks in this meta-analysis (Appendix 7, Table 7.4.2). The research claim, taken from their abstract, was: “data pooled from randomized controlled trials do not reveal a reduction in occurrence of ILI [influenza-like illness] with use of facemask alone in community settings.” We did not construct a p-value plot for this study because two of the five outcome measures failed to meet the eligibility criteria as they were based on self-reported outcomes (with attendant awareness bias) (refer to Appendix 7 for further explanation).
Xiao et al. (2020)116 – The authors used seven RCT base studies comparing medical masks to no masks in this meta-analysis (Appendix 7, Table 7.4.3). The research claim, taken from their abstract, was: “Although mechanistic studies support the potential effect of hand hygiene or face masks, evidence from 14 randomized controlled trials of these measures did not support a substantial effect on transmission of laboratory-confirmed influenza.” We present the p-value plot for this study in Figure 5b.
Incidentally, the Xiao et al. meta-analysis and results replicate exactly an earlier World Health Organization investigation of mask use related to epidemic and pandemic influenza.117 WHO (2019) used the exact same seven base studies in a meta-analysis and reported the exact same quantitative results. The WHO research claim was: “There are a number of high-quality randomized controlled trials demonstrating that personal measures (e.g. hand hygiene and face masks) have at best a small effect on transmission.”118
Nanda et al. (2021)119 – The authors used seven RCT base studies comparing medical masks to no masks in this meta-analysis (Appendix 7, Table 7.4.4). These are the same seven base studies that Xiao et al. (2020) used. However, data extracted by Nanda et al. from the base studies and used for calculating risk ratios and confidence interval differed from that of Xiao et al. The research claim, taken from their abstract, was: “There is limited available preclinical and clinical evidence for face mask benefit in sars-cov-2. RCT evidence for other respiratory viral illnesses shows no significant benefit of masks in limiting transmission.” We present the p-value plot for this study in Figure 6a.
Figure 6. Meta-analysis p-value plots: (a) 7 RCT base studies (Nanda et al. 2021), (b) 8 RCT base studies (Tran et al. 2021)
Also incidentally, for their meta-analysis of RCTs comparing masks alone to no masks for laboratory-confirmed infections, Nanda et al. identified and used the exact same seven base studies as WHO (2019) and Xiao et al. (2020).120 However, data extracted by Nanda et al. from the base studies and used for calculating risk ratios and confidence interval differed compared to WHO (2019) and Xiao et al. (2020).
Tran et al. (2021)121 – The authors used eight RCT base studies comparing medical masks to no masks in this meta-analysis (Appendix 7, Table 7.4.5). Seven of the eight RCT base studies used in their meta-analysis were the exact same as those used by Xiao et al. and Nanda et al. The research claim, taken from their abstract, was: “Given the body of evidence through a systematic review and meta-analyses, our findings supported the protective benefits of MFMs [medical face masks] in reducing respiratory transmissions, and the universal mask-wearing should be applied—especially during the COVID-19 pandemic.” We present the p-value plot for this study in Figure 6b.
Kim et al. (2022)122 – The authors used seven RCT base studies comparing medical masks to no masks in this meta-analysis (Appendix 7, Table 7.4.6). The viral illness outcome they reported was lab-confirmed infection for influenza (6 base studies) and COVID-19 (1 base study). The research claim, taken from their abstract, was: “Evidence supporting the use of medical or surgical masks against influenza or coronavirus infections (SARS, MERS and COVID‐19) was weak.” We present the p-value plot for this study in Figure 7a.
Figure 7. Meta-analysis p-value plots: (a) 7 RCT base studies (Kim et al. 2022), (b) 14 RCT base studies (Liu et al. 2021)
Ollila et al. (2022)123 – The authors used eight RCT base studies comparing medical masks to no masks in this meta-analysis (refer to Table 7.4.7 of Appendix 7). The research claim, taken from their abstract, was: “Our findings support the use of face masks particularly in a community setting and for adults.” We did not construct a p-value plot for this study because six of the eight outcome measures failed to meet the eligibility criteria. Specifically, five of these measures were based on self-reported symptoms (with awareness bias), and we could not confirm the origin of one measure Ollila et al. used for another base study.
Ollila et al. initially registered a protocol for their study in PROSPERO on November 16, 2020, and changed the protocol on May 12, 2022, and again on September 22, 2022, before it was published on December 1, 2022. Also, test statistics used for three of the base studies for self-reported symptoms showing a benefit of mask use (Appendix 7, Table 7.4.7) are opposite to other published data of lab-confirmed statistics for the exact same studies. We present a more-detailed explanation of these and other discrepancies in Section 7.4 of Appendix 7.
2.2.3 Gray literature
Liu et al. (2021)124 – Liu et al. examined available clinical evidence of the effect of face-mask use in community settings on respiratory infection rates, including by COVID-19. This review was different from meta-analyses evaluated here in that it did not specify methodologies for the identification of RCT base studies. However, the authors did present and discuss the results of RCTs that they identified.
As a result of their different methodology, we attempted to obtain original copies of the base studies to confirm their results. They reported outcome measures as p-values for 16 RCT base papers. We obtained only 14 of the 16 base papers. We present these results for the 14 base papers in Table 7.4.8 of Appendix 7.
The research claim, taken from their abstract, was: “Of sixteen quantitative meta-analyses, eight were equivocal or critical as to whether evidence supports a public recommendation of masks, and the remaining eight supported a public mask intervention on limited evidence primarily on the basis of the precautionary principle.” We present the p-value plot for this study, showing results from 14 of the 16 base papers, in Figure 7b.
For all the plots presented here (Figures 5, 6, and 7), we observed no evidence of distinct (single) sample distributions for true effects between two variables. Specifically, this is characterized by ranked p-value points in a plot forming a line with a flat/shallow slope, where most (the majority) of p-values are small, (< 0.05).125
The Jefferson et al. (Figure 5a) and Liu et al. (Figure 7b) p-value plots show evidence of distinct (single) sample distributions for null effects—chance or random associations—between two variables (i.e., p-value points plot as an approximate 45-degree line).126
The Xiao et al. (Figure 5b) and Kim et al. (Figure 7a) p-value plots are only based on seven points, and yet both show evidence of distinct sample distributions for null effects between two variables. The Nanda et al. p-values (Figure 6a) plot closer to a 40-degree line. However, it still clearly supports null effects rather than true effects.
The Tran et al. p-value plot (Figure 6b) exhibits a bilinear shape (divides into two lines)—three p-values are small (<0.05), and five p-values >0.05 are oriented on an approximate 45-degree line. This data set of test statistics is consistent with a two-component mixture and, thus, does not prove a general (overall) claim.
P-values are interchangeable with traditional epidemiology risk statistics (i.e., risk ratios or odds ratios and confidence intervals). Table 3 summarizes the p-values we estimated for risk statistics drawn from base studies used in six meta-analyses. We did not estimate p-values for the Ollila et al. (2022) meta-analysis, and we do not show p-values for the Liu et al. (2021) systematic review. Including those listed in Table 3, Table 7.4.7 in Appendix 7 (Ollila et al. 2022), and Table 7.4.8 in Appendix 7 (Liu et al. 2021), we used a total of 18 base studies across the seven meta-analyses and one systematic review.127
Table 3. Summary of p-values used in six meta-analysis studies.
Meta-analysis: Base study, 1st Author Year | Jefferson et al. | Aggarwal et al. | Xiao et al. | Nanda et al. | Tran et al. | Kim et al. |
Aiello 2010a | 0.0369 | 0.5663 | 0.1626 | 0.007 | ||
Aiello 2012 | 0.4334, 0.7056 | 0.5046 | 0.3187 | 0.4368 | 0.373 | 0.3148 |
Alfelali 2020 | 0.7452 | |||||
Barasheed 2014 | 0.0222 | 0.8815 | 0.2095 | 0.0155 | ||
Bundgaard 2020 | 0.2994 | |||||
Canini 2021 | 0.9367 | 0.9432 | ||||
Cowling 2008 | 0.8074, 0.8763 | 0.2812 | 0.8746 | 0.8063 | 0.4417 | 0.8763 |
Jacobs 2009 | 0.9882 | |||||
MacIntyre 2009 | 0.7342, 0.4456 | 0.4744 | 0.9115 | 0.3404 | 0.4695 | 0.8671 |
MacIntyre 2015 | 0.2421, 0.5483 | |||||
Macintyre 2016 | 0.3868, 0.9939 | 0.7411 | 0.485 | 0.0116 | ||
Simmerman 201 | ||||||
Suees 2012 | 0.36, 0.0241 | 0.4785 | 0.0009 | 0.0167 | 0.0648 | 0.0002 |
Recall that a meta-analysis first involves a systematic review. The meta-analysis then integrates results of identified studies from the systematic review. One would anticipate that well-conducted, independent meta-analysis studies examining the same research question—does medical-mask use in community settings prevent COVID-19 infection?—would identify similar or even the same base studies published within the same period for their analyses. Table 3 shows that while most of the base studies used are similar across the meta-analyses, they are not the same.
An inconsistency apparent in Table 3 is that various, independent meta-analysis researchers have drawn different data from a base study for the exact same research question. Take the Aiello 2010a base study, which is used in four meta-analyses (Table 3). Two meta-analyses used risk statistics that are significant (i.e., p-value < 0.05)—Aggarwal et al. and Tran et al.128 The other two meta-analyses used risk statistics that are non-significant (i.e., p-value > 0.05)—Xiao et al. and Nanda et al.129
This raises the question of why different quantitative results are used by meta-analysis researchers examining the same research question. Is it due to the researchers’ selective analysis and reporting or to some other limitation of the meta-analysis process itself?
4. Discussion and Implications
We aimed to evaluate the reproducibility of research claims in meta-analysis or systematic review studies of mask use in community settings to prevent COVID-19 infection. We identified and evaluated eight eligible studies—seven meta-analyses and one systematic review.
These studies were published between January 1, 2020, and December 7, 2022. We constructed p-value plots to visually inspect the heterogeneity of test statistics combined in these studies. Table 4 compares research claims made in the seven meta-analyses and one systematic review to findings using p-value plots.
A true effect between two variables in meta-analysis should comprise a set of homogeneous (similar) statistics that represent a distinct (single) sample distribution in a p-value plot. This type of effect should show points that align with a shallow slope in the plot. A null effect (chance or random association) between two variables in meta-analysis should show points uniformly distributed over the interval 0 to 1, regardless of sample size, in a p-value plot. This type of effect should show points aligned approximately 45 degrees in the plot.
Table 4. Comparison of meta-analysis research claims to independent results using p-value plots.
Study Detail | Study Research Claim+ | Independent Finding of p-value plot | Is study research claim supported |
Cochrane Review literature: | |||
Jefferson et al. (2020) Meta-analysis | no significant benefit to medical-mask use | null (no) effect | yes |
Medical research literature | |||
Aggarwal et al. (2020) meta-analysis | no significant benefit to medical-mask use | insufficient data to examine | unable to determine |
Xiao et al. (2020) met-analysis | " | null effect | yes |
Nanda et al. (2021) meta-analysis | " | null effect | yes |
Tran et al. (2021) | benefit to medical-mask use | finding is ambiguous (uncertain) | no |
Kim et al. (2022) | " | null effect | no |
Ollila et al. (2022) | " | insufficient data to examine | unable to determine |
Gray literature: | |||
Liu et al. (2021) systematic review | no significant benefit to medical-mask use | null effect | yes |
* All studies examined randomized control of trials of medical-mask versus no-mask use in community settings for the reduction of viral infection (influenza or VOVID-19 virus). |
For six p-value plots constructed (five meta-analyses and one systematic review), we observed no evidence of distinct sample distributions for true effects between two variables. Five of these plots showed points aligned approximately with 45 degrees—indicating null effects. These p-value plots are consistent with chance or random associations (i.e., no proven benefit) for medical-mask use in community settings to prevent viral, including COVID-19, infection.
One other plot, of the data set of Tran et al. (2021) (Figure 6b), had p-value points divided into two lines, consistent with a heterogenic or dissimilar data set (two-component mixture). Here there is insufficient evidence to make a research claim because of ambiguity (uncertainty) in the data set used for meta-analysis.
We did not construct p-value plots for two other meta-analyses—Aggarwal et al. (2020) and Ollila et al. (2022)—because of their overreliance on self-reported outcomes (with attendant awareness bias) and other irregularities (i.e., biases).
Wang et al. (2021) present ample evidence of airborne transmission for many respiratory viruses.130 These include influenza virus, respiratory syncytial virus (RSV), human rhinovirus, severe acute respiratory syndrome coronavirus (SARS-CoV), Middle East respiratory syndrome coronavirus (MERS-CoV), SARS-CoV-2 (COVID-19), measles virus, adenovirus, and enterovirus.
COVID-19 RNA fragments have been identified, and infectious COVID-19 virus has been found in airborne aerosols from 0.25 to >4 mm.131 This is consistent with data observed for the influenza virus, where RNA has been identified in both ≤5 µm and >5 µm aerosols respired from infected hosts, with more influenza virus RNA found in the ≤5 µm aerosols.132 The World Health Organization’s chief scientist recently acknowledged that COVID-19 was an airborne virus spread by aerosols.133
These observations highlight the importance of airborne aerosol transmission and infection for respiratory viruses, including COVID-19. Medical-mask RCTs of influenza infection are directly applicable for understanding the benefit of their use to prevent COVID-19 infection. Again, it is not the virus itself but airborne transmission of aerosols or droplets containing viruses that is important for infection.
Where observational data are used in randomized (or even non-randomized) medical intervention studies, a strong statistical component is required to establish informative and interpretable intervention−risk/benefit associations. This is also the case for research claims made from these associations. For a research claim to be considered valid, it must defeat randomness (i.e., a statistical outcome due to chance).
The p-value plots for five studies—Jefferson et al. (2020), Xiao et al. (2020), Nanda et al. (2021), Kim et al. (2022), and Liu et al. (2021)—show results that look random. This is consistent with research claims made by Jefferson et al. (2020), Xiao et al. (2020), Nanda et al. (2021), and Liu et al. (2021), i.e., no significant benefit to medical-mask use (Table 4).
In short, p-value plots were able to reproduce and support their research claims. These reproducible results strengthen the claim that medical masks have an unproven benefit in community settings to prevent respiratory virus infections. This has been reported several years ago134 and more recently.135
The finding of randomness in the p-value plot (Figure 7a) is opposite to the research claim of Kim et al. (2022) (benefit to medical-mask use). This implies that their claim is irreproducible. We did not evaluate the reproducibility of claims by Aggarwal et al. (2020) (no benefit to medical-mask use) and Ollila et al. (2022) (benefit to medical-mask use) because of insufficient data for p-value plots. We judge the latter meta-analysis to be unreliable due to its overreliance on self-reported outcomes (with awareness bias) and discrepancies (i.e., biases).
For an intervention to be useful and practical to a population, any benefit of the intervention must be of sufficient magnitude to observe a difference in an outcome between the intervention group and a control group at the population level. Consider Germany and Sweden during the COVID-19 pandemic. Germany had a mask mandate for its population, whereas Sweden did not. Comparing mask-wearing compliance and COVID-19 outcome data among the populations of these two countries represents evidence for a natural experiment on the mask-benefit question.
Survey data on mask-wearing compliance during the pandemic was captured in many countries by the University of Maryland (UMD) Social Data Science Center, which collaborated with Facebook.136 One of the survey questions asked Facebook users if they wore a mask most or all the time in the previous five days. Figure 8 shows Facebook-user-reported monthly average mask compliance (%) during the second COVID-19 wave—October 2020 through June 2021—in Germany and Sweden. Figure 8 shows that mask compliance in Germany was never less than 80%, whereas mask compliance in Sweden was never more than 21%.
Figure 8. Facebook-user-reported monthly average mask compliance (%) during the second COVID-19 wave in Germany and Sweden.137
What role might masks have played during the second wave? Consider Figure 9, depicting a severe pandemic outcome measure—daily new COVID-19 deaths per million population in Germany and Sweden. Figure 9 was originally derived by Miller (2022)138 and is reproduced here using data from the World Health Organization COVID-19 dashboard.139
Figure 9. Daily new COVID-19 deaths per million population during the second wave in Germany and Sweden.140
Both countries had considered and implemented a variety of pandemic policies, including masking policies, by the second wave of COVID-19 infection (fall 2020). Germany, by April 2020,141 and Sweden, by March 2020,142 had public policies on masks prior to the second wave. Additional information on the practices and evolution of Germany’s and Sweden's COVID-19 pandemic prevention measures is available elsewhere.143
Public-health risk factors for morbidity and mortality are multi-factorial. Numerous features may be at play in the risk factor−health outcome chain across a population. These can include access to health care, health status, lifestyle, quality of life, standard of living, etc. Germany and Sweden are members of the European Union with similar national-health policies and similar laws and standards for health products and services.144 Both should have had similar health-care capacities to respond to the COVID-19 pandemic.
Also, in 2020 both Germany and Sweden ranked closely in the top 10 countries of the world on the United Nations Human Development Index (HDI)—Germany 6th, Sweden 7th.145 The United Nations HDI tracks measures of life expectancy at birth (health status measure), years of schooling (knowledge measure), and gross national income per capita (standard of living measure).
At the population level, a first impression of Figure 8 and Figure 9 is that mask use had little or no benefit in preventing COVID-19 deaths during the second wave. Despite similar health-care capacities, similar United Nations HDI measures, and obvious differences in mask-wearing compliance for these countries (Figure 8), WHO-reported daily COVID-19 deaths per million population are not much different (Figure 9).
These figures provide strong evidence that those arguing for the community use of masks to reduce COVID-19 deaths have not made their case. In lay terms, masks did not work.
Technical Studies: Conclusions
Our technical studies provide substantial evidence that two notable nonpharmaceutical public health interventions—lockdowns and masks—had no proven benefit to public health outcomes. This is consistent with known economic and public health harms of these policies as reported by others.146
We do not believe that our results should be interpreted simply as a suggestion for reform of the existing system of epidemiological modeling—although that modeling system certainly should be reformed. Our technical studies suggest a far greater frailty (failure) in the system of epidemiological analysis and policy recommendations.
That system, generally, grossly overestimated the potential effect of COVID-19147 and, particularly, overestimated the potential benefit of health measures such as lockdowns and masks.148 The epidemiological modeling community failed to alter its policy recommendations even as case fatality ratio and infection fatality ratio numbers changed substantially. These errors are so great as to cast doubt on the entire system.
Epidemiological modeling of the potential effect of COVID-19 was an unreliable exercise—it models a circle of mirrors that inform researchers of their formulas and their parameters, and not of the real world.149 We believe our technical studies support recommendations for policy change to restructure the entire system of government policy based on epidemiological modeling, and not simply to apply cosmetic reforms to the existing system.
Recommendations
Introduction
The CDC and associated professions now rely heavily on a combination of epidemiology, statistics, and mathematical modeling. They do so to alter all sorts of individual and collective behavior, in the name of public health. This is alarming in itself, because public health agencies have taken it upon themselves to shift, for example, how people eat and whether or not to smoke. Of course, there are public health justifications—but this also allows the state and its servants to determine how citizens should live. Even with this relatively narrow scope, it is an astonishing expansion of state authority over individual lives.
But we should not believe that it will be confined to this scope. Already a remarkably large number of subject matters is being subsumed under “public health,” including secondary (“perimetric”) boycotts of institutions funded by tobacco companies,150 fossil fuel divestment,151 Independence Day fireworks,152 so-called “anti-racism,”153 the anti-Israel Boycott, Divest, and Sanction (BDS) movement,154 and “social policy” generally:
Public health problems, whether new or old, are essentially social in character and can only be solved in terms of social policy. The task of public health workers is to convince society to undertake the specific social measures, governmental or other, which are required to solve specific health problems, and to participate in the implementation of these policies. Avoidance of the need for developing effective social policies for health in favor of a sole concentration on problems of individual health behavior is not only oversimplification but an evasion of public health responsibility.155
With such a wide remit, it is very much worth considering whether public health techniques will be used to abridge free speech in the name of public health. We know, for example, that Twitter blacklisted dissenters from the government’s COVID-19 policy to reduce the influence of their skepticism.156 Even broader interventions are more than plausible. Outside the realm of epidemiology, for example, machine-learning experts have been exploring how to remove what they call “hate speech”:
The detection of online hate speech should be accompanied with a strong control strategy so that Internet users can be deterred from posting such texts. User warnings and word removal recommendations are often used to implement such control mechanisms. However, merely asking users to remove hate-related keywords is not a strong enough control strategy, as users often come up with alternate ways to post such texts by surpassing the detection mechanisms. Moreover, the other words in a text that are semantically related to such keywords (such as names of individuals or group) can still significantly harm the targeted individuals or groups. Therefore, a control strategy that can systematically point out these semantically related words is very important for effectively controlling these instances of hate speech.157
Epidemiology already concerns itself with “surveillance” in the health context. It is reasonable to worry about the conflation of public health modeling and the parallel work by computer scientists to establish a broader surveillance state, to fear the marriage of the epidemiological model with the computer science algorithm. Meme transmission can be modeled; so can “public health” efforts to inhibit the reproduction of memes.
Given the dangers that such epidemiological modeling poses to individual liberty, one category of recommendations should be to limit its application. Another category of critique is to reduce reliance on modeling altogether. Briggs has written on the arbitrary nature of mathematical modeling, and the COVID-19 experience does support his contention. Put another way, Gelman and Loken’s “garden of forking paths” applies peculiarly to the world of modeling public health interventions. Gelman and Loken wrote of the world of statistical analysis that,
When we say an analysis was subject to multiple comparisons or “researcher degrees of freedom,” this does not require that the people who did the analysis were actively trying out different tests in a search for statistical significance. Rather, they can be doing an analysis which at each step is contingent on the data. The researcher degrees of freedom do not feel like degrees of freedom because, conditional on the data, each choice appears to be deterministic. But if we average over all possible data that could have occurred, we need to look at the entire garden of forking paths and recognize how each path can lead to statistical significance in its own way. Averaging over all paths is the fundamental principle underlying p-values and statistical significance and has an analogy in path diagrams developed by Feynman to express the indeterminacy in quantum physics.158
Researcher degrees of freedom apply to mathematical modeling. But modeling public health interventions translates these degrees of freedom from understanding the world to recommending policy; researcher degrees of freedom become intervention degrees of freedom.
We may add to this the critique that modeling by its nature is intended to facilitate state action, and tilts against the recommendation to do nothing.159 Modeling justifies state action; modeling relies on intervention degrees of freedom. So far as individual liberty is concerned, the wiser course is to refrain from modeling altogether.
A third set of recommendations is to improve the way modeling is done, if it is to be done at all. While modelers generally seek to improve their product, these recommendations would follow the line of critique of Ioannidis and his fellow meta-researchers, who have leveled searching critiques of the entire current practice of modeling.
Practically, however, refrain from modeling is not an enforceable recommendation—and while modeling may tilt toward state action, prohibiting or restricting modeling may not be a practical solution. Furthermore, reforms to enhance liberty and reforms to improve modeling both will serve practically to cover much of the territory of refrain from modeling.
We therefore provide two different categories of recommendations related to modeling—one to preserve liberty and one to improve modeling. These categories should serve jointly to impede unreliable modeling that facilitates state action. To the extent that these two categories conflict, we advise that our recommendations to preserve liberty override our recommendations to improve modeling.
Institutional and Legal Responsibility
We make our recommendations to the CDC, but we recognize that our criticism of epidemiological modeling, and the corollary public health interventions, does not rest solely with the CDC. A great many individual scientists conduct modeling. The CDC, the WHO, and a host of other organizations make public health recommendations. A large variety of organizations then undertook policy actions. If the CDC made an explicit recommendation to wear masks, states and localities generally issued lockdown orders.160 The responsibility for COVID-19 public health interventions is widely distributed.
Drabiak has established 1) that the constitution, federal law, and state law require clear and present justifications for the authority to impose lockdowns, masks, and other infringements of liberty; and 2) that local, state, and federal authorities exceeded their statutory authority during the COVID-19 pandemic.161 The justification for such excess would have to be prudential—that there was indeed a pandemic of such virulence and lethality that the law had to be suspended temporarily. This does not appear to have been the case. But who, in this labyrinth of authorities, is to be held accountable? Who should be asked by the public to commit to institutional reforms? This wide distribution of responsibility limits both democratic accountability and the possibility of institutional reform.
We direct our recommendations particularly to the CDC, because 1) it holds the greatest single responsibility in the federal government for communicating accurate information about diseases to policymakers and the public; 2) it played a key role in coordinating and articulating American policy responses to COVID-19; and 3) its role to fund research and develop future guidelines for public health policy gives it the greatest ability to shift the professional incentives of the overlapping fields of epidemiology, statistics, and modeling. We do not hold the CDC exclusively responsible for the mishaps of American COVID-19 policy, but we believe it has the greatest ability, and therefore the greatest responsibility, to enact constructive reforms to preclude a recurrence of such mishaps in the future.
Elected policymakers, however, also ought to take responsibility for public health policy. During the COVID-19 pandemic, for example, Florida Governor Ron DeSantis refused to defer to the supposed consensus of professional expertise. He investigated the data himself, made policy based upon his own informed sense of COVID-19’s nature and of proper public health responses, and provided a superior policy response to those of the professional experts. Elected policymakers should be more confident in their capacity to judge crises that require substantial expert knowledge. They need not take this confidence to unrealistic extremes, but they can and should take personal responsibility for making policy decisions in such crises, and not delegate their powers to professional experts or their models.
This recommendation to elected policymakers noted, we direct the following recommendations to the CDC in particular, and more generally to government, to the modeling profession, and to Americans as a whole.
Modeling Recommendations: Liberty
- Liberty Commission: Congress and the president should jointly convene an expert commission, drawing upon noted defenders of civil liberties such as Greg Lukianoff and Glenn Greenwald, as well as epidemiological experts in different agencies and professions, to delimit the areas of private life which may be subject to public health interventions. This commission also should draft rules articulating the principles it has drafted as detailed guidelines limiting what public health interventions, or research regarding health interventions, any federal government may fund, conduct, or allow.
- Define Scope of Public Health Interventions: We recommend that this commission’s rules explicitly limit the scope of public health interventions to physical health, narrowly and carefully defined, and explicitly define any aspect of concepts such as mental health, environmental health, and social health, which warrant the intervention of public health authorities in matters that properly should be decided freely by individuals or their elected policymakers.
- Define Scope Narrowly: We recommend that this commission’s rules explicitly and narrowly limit how public health interventions may change individual and collective behavior, and that all such public health interventions be required to receive explicit sanction from both houses of Congress. Above all, we recommend that public health interventions should not aim to alter public judgment of a public policy; public judgment should determine public health policy, not vice versa.
Modeling Recommendations: Technical Improvements
As the authors of Protecting the Integrity of Government Science (2022) wrote, “The American public has the right to expect from its government accurate information, data, and evidence and scientifically-informed policies, practices, and communications. This requires scientific integrity—based on rigorous scientific research that is free from politically motivated suppression or distortion.”162 Therefore, we recommend that the CDC, and all other federal agencies involved with epidemiological modeling, draft rules regulating the models it funds, conducts, or allows, to ensure transparency, rigor, and depoliticization. These rules should include:
- Require Pre-registration of Mathematical Modeling Studies. The CDC should formulate rules requiring the pre-registration of mathematical modeling studies, including:
- prospective validation practices;
- pre-specified, agreed-upon rules for judging success and/or the need for recalibration;
- registries f existing past models;
- data, code, and software sharing and reporting transparency; and
- unbiased reporting and complete documentation of past model performance.163
- Require Mathematical Modeling Transparency and Reproducibility. The CDC should formulate rules requiring:
- greater reliance n unbiased data and less reliance on theoretical speculation;
- transparent release f underlying data and models, to allow anyone to analyze model input data, model predictions, and model outcome data;
- division of data set construction from data set analysis;
- modeling the entire predictive distribution, with a particular focus on accurately quantifying uncertainty;
- continuously monitoring the performance of any model against real data and either re-adjusting or discarding models based on accruing evidence;
- avoiding unrealistic assumptions about the benefits of interventions;
- using up-to-date and well-vetted tools and processes that minimize the potential for error through auditing loops in the software and code;
- maintaining an open-minded approach and acknowledging that most forecasting is exploratory, subjective, and non-pre-registered research; and
- articulating efforts to avoid unavoidable selective reporting bias.164
The CDC also should limit and require articulate defenses of all arbitrary “weight of evidence” judgments that inform mathematical models.165
- Reduce Intervention Degrees of Freedom. The CDC should formulate rules to reduce intervention degrees of freedom. These rules will overlap with those for pre-registration, transparency, and reproducibility, but they should be framed explicitly to reduce these degrees of freedom.
- Reconceive of Modeling as Measuring Uncertainty. Gelman has severely criticized the use of the term confidence interval, which gives unwary researchers the mistaken impression that a statistical operation can and should be used to establish sufficient knowledge. He prefers the term uncertainty interval, although Greenland prefers compatibility interval; these changes in nomenclature are intended to reinforce the truth that statistics can and should aim at measuring uncertainty rather than establishing certainty.166 This concept also should be applied to modeling, especially where it depends upon statistical operations. As Briggs puts it, “The goal of probability models is to quantify uncertainty in an observable Y given assumptions or observations X. That and nothing more.”167 The CDC should formulate guidelines that make explicit that modeling is meant to quantify uncertainty, and that models should convey to policymakers a quantification of the uncertainties of action rather than a prescription of certainty to justify action.
- Technical Improvements Commission. The CDC should charter a commission to advise it in how to achieve these goals. This commission should include experts such as William M. Briggs, Andrew Gelman, and John Ioannidis.
Further Commissions
- COVID-19 Commission. The federal government commissioned an investigation after 9/11 to determine the full scale of security policy errors that had led to such a catastrophe. We recommend that the federal government commission a full-scale report on the origins and nature of COVID-19, as well as of public health policy errors committed during the response to COVID-19. Errors to be investigated should include every instance of politicization of COVID-19 public health policy, and censorship of discussion of COVID-19 policy, as well as the role of public and private entities (e.g., social media companies) in forwarding politicization and censorship.168 This commission should be empowered to subpoena data from all relevant government agencies and private entities and to publicize it. It should also present concrete suggestions for reforms to prevent the recurrence of policy errors, politicization, and censorship.
While we would wish that such a commission include articulate defenders of what the government did correctly, it should include large numbers of professional critics of government policy, such as John Ioannidis, Jay Bhattacharya, and Martin Kulldorf. This commission, moreover, should be directed not to require a consensus report, but to welcome divisions of opinion, with majority and minority reports. The public should welcome, and be accustomed to, the idea that experts disagree.
- Computer Science Commission. Public health modeling naturally aligns with the use of computer science algorithms; social media censorship of COVID-19 policy discourse depended on both. Public health modeling is well suited to provide a plausible justification for using computer science algorithms to limit public debate—and, with all its flaws, may provide useful techniques for censorship that abrogates Americans’ First Amendment rights. When public health defines the transmission of ideas as a communicable disease that threatens public health, it has a broad arsenal of tools to inhibit such transmission. The federal government also should establish a commission to provide guidelines for federal funding, conduct, and regulation of the use of computer science algorithms, particularly as they are used by the federal government and by social media companies. This commission, moreover, should provide guidelines to ensure that artificial-intelligence programming is not similarly subverted to inhibit liberty.
Conclusion
The CDC, faced with what it took to be a highly lethal COVID-19 pandemic, believed that failing to act boldly to preserve public health would have risked catastrophic consequences. Yet its perception of COVID-19’s lethality was at great variance with reality. Its overreaction imposed unnecessary and gravely deleterious consequences on America. The CDC vividly demonstrated the downside of applying the precautionary principle to public health169—unless one says that the precautionary principle should be applied to the preservation of liberty and prosperity.
We cannot say that to take such precautions always will be wrong. Indeed, much government policy in all areas of life consists practically of a pendulum swing from an excess in one direction to an excess in another. Yet we believe that COVID-19 policy has shown that applying the precautionary principle is gravely detrimental to public welfare. This lesson should be applied to all areas of public life. If the precautionary principle has failed so badly in one instance, it may in another.
We may put this into the language of Bayesian statistics. We have new evidence about the efficacy of the precautionary principle, and we should use it to update our priors about the general efficacy of that principle.
We do not wish government policy to swing too far toward underreaction, when a truly serious crisis emerges. But we believe that the lesson of COVID-19 is that government must require more rigorous procedures to justify an equivalent level of government intervention. Our administrative procedures should not lead us by default to the constriction of liberty and prosperity.
Our public health system is now on a path toward technocratic tyranny—one which doesn’t even improve public health. Americans must create a new system. Public health should not be the health of the state, but the health of individual liberty.
Appendices
Appendix 1: Multiple Testing and Multiple Modeling (MTMM) and Epidemiology
Multiple Testing and Multiple Modeling (MTMM) controls for experiment-wise error—the probability that at least one individual claim will register a false positive when you conduct multiple statistical tests.170 It is instructive to trace some of the history of MTMM with examples related to epidemiology.
Friedman made a research claim in 1959 that Type A personality was associated with heart attacks.171 Several later studies failed to replicate these results. Expert committees found fault with these later studies, and the claim lives to this day. Yet Friedman’s initial study examined hundreds of distinct analytical questions. It is very likely that the association is nothing more than a multiple-testing false positive.172
In 1974, a Lancet paper noted a correlation between the popular blood-pressure drug reserpine and breast cancer, with a p-value < 0.01.173 Several later studies failed to replicate these results.174 Samuel Shapiro, a co-author of the original Lancet paper, later explained that,
Slone and I came to realize that our initial hypothesis-generating study was sloppily designed and inadequately performed. In addition, we had carried out, quite literally, thousands of comparisons involving hundreds of outcomes and hundreds (if not thousands) of exposures. As a matter of probability theory, ‘statistically significant’ associations were bound to pop up and what we had described as a possibly causal association was really a chance finding.175
Yale epidemiologist Alvan Feinstein provided the first rigorous insight into epidemiology’s multiple-testing (MTMM) problem in two 1988 papers. Feinstein’s first paper counted published studies for and against 56 different research claims and found that there were roughly as many studies supporting each particular claim as there were studies rejecting the claim.176
Feinstein’s second paper argued that the researchers he studied did not begin their research with a defined, single question. Instead, they allowed the data to define the question and then published the results.177 An enormous proportion of epidemiological research conclusions were the result of multiple testing and (in modern nomenclature) HARKing—hypothesizing after the result was known.
Statisticians have long been aware of the pitfalls of multiple testing: practitioners are keenly aware that error probabilities are not maintained when there is multiple testing of the same set of data.178 In the 1970s and 1980s, statisticians produced considerable literature on applied medical work that examined associations of blood types with disease.179
In 1985, Westfall observed that the relevant research produced multiple confidence intervals, and that these intervals could be made just wide enough to provide a proper correction parameter for the body of multiple tests by using resampling techniques that preserved the overall family-wise error rate. This assesses the chance of producing a false-positive result while making multiple statistical tests. In other words, researchers who used resampling techniques now had a practical way to assess the probability that multiple testing had produced false-positive results.180 Simulation could solve the otherwise intractable multiple-testing problem.
Epidemiologists, unfortunately, instead decided as a body to disregard the multiple-testing challenge identified by Feinstein. In 1990, the lead editorial in the very first issue of the new journal Epidemiology explicitly articulated this disregard in its title: “No Adjustments Are Needed for Multiple Comparisons.”181 The discipline, alas, generally has followed this counsel.
A book offering practical solutions to the multiple-testing problem has been available since 1993182 and has been cited more than 3,500 times since;183 but very rarely is it used or cited in major epidemiology journals.184 In 2000, Clyde did recognize that environmental epidemiology needed to account for multiple modeling and proposed a Bayesian model average as a solution.185 The field also has paid limited attention to this alternate solution. Clyde (2000) has only been cited twice in the leading environmental epidemiology journal Environmental Health Perspectives.186
Hayat et al. recently analyzed 216 randomly selected articles from a total of 1,023 published in 2013 by seven influential public health journals (American Journal of Public Health, American Journal of Preventive Medicine, International Journal of Epidemiology, European Journal of Epidemiology, Epidemiology, American Journal of Epidemiology, and Bulletin of the World Health Organization). Only 5.1% of these studies reported making statistical corrections for multiple testing.187 We speculate that the studies that performed these corrections were in the genetic epidemiology subdiscipline. As a whole, epidemiologists have not subjected their research to the severe test of Multiple Testing and Multiple Modeling. Their unwillingness to do so warrants significant skepticism of all the field’s results.
Appendix 2: Statistical Significance
What is Statistical Significance?
The requirement that a research result be statistically significant has long been a convention of epidemiologic research.188 In hundreds of journals, in a wide variety of disciplines, you are much more likely to get published if you claim to have a statistically significant result. To understand the nature of the irreproducibility crisis, we must examine the nature of statistical significance. Researchers try to determine whether the relationships they study differ from what can be explained by chance alone by gathering data and applying hypothesis tests, also called tests of statistical significance.
In practice, the hypothesis that forms the basis of a test of statistical significance is rarely the researcher’s original hypothesis that a relationship between two variables exists. Instead, scientists almost always test the hypothesis that no relationship exists between the relevant variables. Statisticians call this the null hypothesis. As a basis for statistical tests, the null hypothesis is mathematically precise in a way that the original hypothesis typically is not. A test of statistical significance yields a mathematical estimate of how well the data collected by the researcher supports the null hypothesis. This estimate is called a p-value.
It is traditional in the epidemiological disciplines to use confidence intervals instead of p-values from a hypothesis test to demonstrate statistical significance. As both confidence intervals and p-values are constructed from the same data, they are interchangeable, and one can be estimated from the other.189 Our use of p-values in this report implies that they can be (and are) estimated from the confidence intervals used in environmental epidemiology studies.
The Bell Curve and the P-Value: The Mathematical Background All “classical” statistical methods rely on the Central Limit Theorem, proved by Pierre-Simon Laplace in 1810. The theorem states that if a series of random trials is conducted, and if the results of the trials are independent and identically distributed, then the resulting normalized distribution of actual results, when compared to the average, will approach an idealized bell-shaped curve as the number of trials increases without limit. By the early twentieth century, as the industrial landscape came to be dominated by methods of mass production, the theorem found application in methods of industrial quality control. Specifically, the p-test naturally arose in connection with the question, “how likely is it that a manufactured part will depart so much from specifications that it won’t fit well enough to be used in the final assemblage of parts?” The p-test, and similar statistics, became standard components of industrial quality control. It is noteworthy that during the first century or so after the Central Limit Theorem had been proved by Laplace, its application was restricted to actual physical measurements of inanimate objects. While philosophical grounds for questioning the assumption of independent and identically distributed errors existed (i.e., we can never know for certain that two random variables are identically distributed), the assumption seemed plausible enough when discussing measurements of length, or temperature, or barometric pressure. Later in the twentieth century, to make their fields of inquiry appear more “scientific,” social scientists began to apply the Central Limit Theorem to human data, even though nobody can possibly believe that any two human beings—the things now being measured—are truly independent and identical. The entire statistical basis of “observational social science” rests on shaky supports, because it assumes the truth of a theorem that cannot be proved applicable to the observations that social scientists make. |
A p-value estimated from a confidence interval is a number between zero and one, representing a probability based on the assumption that the null hypothesis is actually true.190 A very low p-value means that, if the null hypothesis is true, the researcher’s data are rather extreme—surprising, because a researcher’s formal thesis when conducting a null hypothesis test is that there is no association or difference between two groups. It should be rare for data to be so incompatible with the null hypothesis. But perhaps the null hypothesis is not true, in which case the researcher’s data would not be so surprising. If nothing is wrong with the researcher’s procedures for data collection and analysis, then the smaller the p-value, the less likely it is that the null hypothesis is correct.
In other words: the smaller the p-value, the more reasonable it is to reject the null hypothesis and conclude that the relationship originally hypothesized by the researcher does exist between the variables in question. Conversely, the higher the p-value, and the more typical the researcher’s data would be in a world where the null hypothesis is true, the less reasonable it is to reject the null hypothesis. Thus, the p-value provides a rough measure of the validity of the null hypothesis—and, by extension, of the researcher’s “real hypothesis” as well.191 Or it would, if a statistically significant p-value had not become the gold standard for scientific publication.192
Why Does Statistical Significance Matter?
The government’s central role in science, both in funding scientific research and in using scientific research to justify regulation, further disseminated statistical significance throughout the academic world. Within a generation, statistical significance went from a useful shorthand that agricultural and industrial researchers used to judge whether to continue their current line of work, or switch to something new, to a prerequisite for regulation, government grants, tenure, and every other form of scientific prestige—and also, crucially, the essential prerequisite for professional publication.
Scientists’ incentive to produce positive, original results became an incentive to produce statistically significant results. Groupthink, frequently enforced via peer review and editorial selection, inhibits the publication of results that run counter to disciplinary or political presuppositions.193 Many more scientists use a variety of statistical practices, with more or less culpable carelessness, including:
- improper statistical methodology;
- consciously or unconsciously biased data manipulation that produces desired outcomes;
- choosing between multiple measures of a variable, selecting those that provide statistically significant results, and ignoring those that do not; and
- using illegitimate manipulations of research techniques.194
Still others run statistical analyses until they find a statistically significant result—and publish the one (likely spurious) result. Far too many researchers report their methods unclearly and let the uninformed reader assume they actually followed a rigorous scientific procedure.195 A remarkably large number of researchers admit informally to one or more of these practices—which, collectively, are known as p-hacking.196 Significant evidence suggests that p-hacking is pervasive in an extraordinary number of scientific disciplines.197 HARKing is the most insidious form of p-hacking.
Appendix 3: The Irreproducibility Crisis of Modern Science
The Catastrophic Failure of Scientific Replication
Let us briefly review the methods and procedures of science. The empirical scientist conducts controlled experiments and keeps accurate, unbiased records of all observable conditions at the time the experiment is conducted. If a researcher has discovered a genuinely new or previously unobserved natural phenomenon, other researchers—with access to his notes and some apparatus of their own devising—will be able to reproduce or confirm the discovery. If sufficient corroboration is forthcoming, the scientific community eventually acknowledges that the phenomenon is real and adapts existing theory to accommodate the new observations.
The validation of scientific truth requires replication or reproduction. Replicability (most applicable to the laboratory sciences) most commonly refers to obtaining an experiment’s results in an independent study, by a different investigator with different data, while reproducibility (most applicable to the observational sciences) refers to different investigators using the same data, methods, and/or computer code to reach the same conclusion.198 We may further subdivide reproducibility into methods reproducibility, results reproducibility, and inferential reproducibility.199 Scientific knowledge only accrues as multiple independent investigators replicate and reproduce one another’s work.200
Yet today the scientific process of replication and reproduction has ceased to function properly. A vast proportion of the scientific claims in published literature have not been replicated or reproduced; credible estimates are that a majority of these claims cannot be replicated or reproduced—that they are, in fact, false.201 An extraordinary number of scientific and social-scientific disciplines no longer reliably produce true results—a state of affairs commonly referred to as the irreproducibility crisis (reproducibility crisis, replication crisis). A substantial majority of 1,500 active scientists recently surveyed by Nature called the current situation a crisis; 52% judged the situation a major crisis and another 38% judged it “only” a minor crisis.202 The increasingly degraded ordinary procedures of modern science display the symptoms of catastrophic failure.203
The scientific world’s dysfunctional professional incentives bear much of the blame for this catastrophic failure.
The Scientific World’s Professional Incentives
Scientists generally think of themselves as pure truth-seekers who strive to follow a scientific ethos roughly corresponding to Merton’s norms of universalism, communality, disinterestedness, and organized skepticism.204 Public trust in scientists205 generally derives from a belief that they adhere successfully to those norms. But this self-conception differs markedly from reality.
Knowingly or unknowingly, scientists respond to economic and reputational incentives as they pursue their own self-interest.206 Buchanan and Tullock’s work on public choice theory provides a good general framework. Politicians and civil servants (bureaucrats) act to maximize their self-interest rather than acting as disinterested servants of the public good. 207 This general insight applies specifically to scientists, peer reviewers, and government experts.208 The different participants in the scientific research system all serve their own interests as they follow the system’s incentives.
Well-published university researchers earn tenure, promotion, lateral moves to more prestigious universities, salary increases, grants, professional reputation, and public esteem—above all, from publishing exciting, new, positive results. The same incentives affect journal editors, who receive acclaim for their journal, and personal reputational awards, by publishing exciting new research—even if the research has not been vetted thoroughly.209 Grantors want to fund the same sort of exciting research—and government funders has the added incentive that exciting research with positive results also supports the expansion of their organizational mission.210 American university administrations want to host grant-winning research, from which they profit by receiving “overhead” costs—frequently a majority of overall research grant costs.211
All these incentives reward published research with new, positive claims—but not reproducible research. Researchers, editors, grantors, bureaucrats, university administrations—each has an incentive to seek out the exciting new research that draws money, status, and power, but few or no incentives to double-check their work. Above all, they have little incentive to reproduce the research, to check that the exciting claim holds up—because if it does not, they will lose money, status, and prestige.
Each member of the scientific research system, seeking to serve his own interest, engages in procedures guaranteed to inflate the production of exciting, but false, research claims in peer-reviewed publications. Collectively, the scientific world’s professional incentives do not sufficiently reward reproducible research. We can measure the overall effect of the scientific world’s professional incentives by analyzing publication bias.
Academic Incentives versus Industrial Incentives Far too many academics and bureaucrats, and a distressingly large portion of the public, believe that university science is superior to industrial science. University science is believed to be disinterested; industrial science is believed to be corrupted by the desire to make a profit. University science is believed to be accurate and reliable; industrial science is not.212 Our critique of the scientific world’s professional incentives is, above all, a critique of university science incentives. According to one study, zero out of 52 epidemiological claims in randomized trials could be replicated.213 According to another, only 36 of 100 of the most important psychology studies could be replicated.214 Nutritional research, a tissue of disproven claims such as coffee causes pancreatic cancer, has lost much of its public credibility.215 Academic science, both observational and experimental, possesses astonishingly high error rates—and peer and editorial review of university research no longer provides effective quality control.216 Industrial research is subject to far more effective quality control. Government-imposed Good Laboratory Practice Standards, and their equivalents, apply to a broad range of industrial research—and do not apply to university research.217 Industry, moreover, is subject to the most effective quality control of all—a company’s products must work, or it will go out of business.218 Both the profit incentive and government regulation tend to make industrial science reliable; neither affects academic science. As we will see below, environmental epidemiology regulation is largely based on university research. We should treat it with the same skepticism as we would industrial research. |
Publication Bias: How Published Research Skews Toward False-Positive Results
The scientific world’s incentives to publish exciting research rather than reproducible research drastically affect which research scientists submit for publication. Scientists who try to build their careers on checking old findings or publishing negative results are unlikely to achieve professional success. The result is that scientists simply do not submit negative results for publication. Some negative results go to the file drawer. Others somehow turn into positive results as researchers, consciously or unconsciously, massage their data and their analyses. Neither do they perform or publish many replication studies, since the scientific world’s incentives do not reward those activities either.219
We can measure this effect by anecdote. One co-author recently attended a conference where a young scientist stood up and said that she spent six months trying unsuccessfully to replicate a literature claim. Her mentor said to move on—and that failed replication never entered the scientific literature. Individual papers also recount problems, such as difficulties encountered when correcting errors in peer-reviewed literature.220 We can quantify this skew by measuring publication bias—the skew in published research toward positive results compared with results present in the unpublished literature.221
A body of scientific literature ought to have a large number of negative results, or results with mixed and inconclusive results. When we examine a given body of literature and find an overwhelmingly large number of positive results, especially when we check it against the unpublished literature and find a larger number of negative results, we have evidence that the discipline’s professional literature is skewed to magnify positive effects, or even create them out of whole cloth.222
As far back as 1987, a study of the medical literature on clinical trials showed a publication bias toward positive results: “Of the 178 completed unpublished randomized controlled trials (RCTs)223 with a trend specified, 26 (14%) favored the new therapy compared to 423 of 767 (55%) published reports.”224 Later studies provide further evidence that the phenomenon affects an extraordinarily wide range of fields, including:
- the social sciences generally, where “strong results are 40 percentage points more likely to be published than are null results and 60 percentage points more likely to be written up”;225
- climate science, where “a survey of Science and Nature demonstrates that the likelihood that recent literature is not biased in a positive or negative direction is less than one in 5.2 × 10−16”;226
- psychology, where “the negative correlation between effect size and samples size, and the biased distribution of p values indicate pervasive publication bias in the entire field of psychology”;227
- sociology, where “the hypothesis of no publication bias can be rejected at approximately the 1 in 10 million level”;228
- research on drug education, where “publication bias was identified in relation to a series of drug education reviews which have been very influential on subsequent research, policy and practice”;229 and
- research on “mindfulness-based mental health interventions,” where “108 (87%) of 124 published trials reported ≥1 positive outcome in the abstract, and 109 (88%) concluded that mindfulness-based therapy was effective, 1.6 times greater than the expected number of positive trials based on effect size.”230
Publication bias especially leads to a skew in favor of research that erroneously claims to have discovered a statistically significant relationship in its data.
Appendix 4: P-value Plotting: A Severe Test for Publication Bias, P-hacking, and HARKing
Introduction
We use p-value plotting to test whether a field has been affected by the irreproducibility crisis—by publication bias, p-hacking, and HARKing. In essence, we analyze meta-analyses of research and output their results on a simple plot that displays the distribution of p-value results:
- A literature unaffected by publication bias, p-hacking, or HARKing should display its results as a single line.
- A literature that has been affected by publication bias, p-hacking, or HARKing should display bilinearity—results visible as two, separated lines.
P-value plotting of meta-analyses’ results allows a reader, at a glance, to determine whether there is circumstantial evidence that a body of scientific literature has been affected by the irreproducibility crisis.
We will summarize here the statistical components of p-value plotting. We will begin by outlining a few basic elements of statistical methodology: counting; the definition and nature of p-values; and a p-value plotting method that makes it relatively simple to evaluate a collection of p-values. We will then explain what meta-analyses are, and how they are used to inform government regulation. We will then explain how precisely p-value plotting of meta-analyses works, and what it reveals about the scientific literature it tests.231
Counting
Counting can be used to identify which research papers in literature may suffer from the various biases described above. We should want to know how many “questions” are under consideration in a research paper. In a typical nutritional epidemiology paper, for example, there are usually several health outcomes at issue, such as all-cause deaths, cardiovascular endpoints (e.g., heart attack, stroke), diabetes, and various cancers (e.g., breast, colorectal, gallbladder, and liver). Researchers consider whether a risk factor, such as individual food frequencies, predicts any of these health outcomes—that is to say, whether they are “positively” associated with a particular health outcome. When they study foods, epidemiologists may analyze categories including individual food frequencies, food groups, nutrient indexes, and food-group-specific nutrient indexes.
Each of these risk factors is a predictor; each type of health effect is an outcome. Scientists may further analyze an association between a particular food component and a particular health outcome with reference to categories of analysis such as age and sex. Researchers call these further yes/no categories of analysis covariates; covariates may affect the strength of the association, but they are not the direct objects of study.
An epidemiology paper considers the number of questions equal to the product of the number of predictors (P) times the number of outcomes (O) times 2 to the power of the number of yes/no covariates (C). In other words:
the number of questions = P x O x 2C
This formula approximates the number of statistical tests an epidemiology study performs. The larger the number of statistical tests, the easier it is to find a statistically significant association due solely to chance.
P-values
As we have summarized above, a null hypothesis significance test is a method of statistical inference in which a researcher tests a factor (or predictor) against a hypothesis of no association with an outcome. The researcher uses an appropriate statistical test to attempt to disprove the null hypothesis. The researcher then converts the result to a p-value. The p-value is a value between 0 and 1 and is a numerical measure of significance. The smaller the p-value, the more significant the result. Significance is the technical term for surprise. When we are conducting a null hypothesis significance test, we should expect no relationship between any particular predictor and any particular outcome. Any association, any departure from the null hypothesis (random chance), should and does surprise us.
If the p-value is small—conventionally in many disciplines, less than 0.05—then the researcher may reject the null hypothesis and conclude that the result is surprising and that there is indeed evidence for a significant relationship between a predictor and an outcome. If the p-value is large—conventionally, greater than 0.05—then the researcher should accept the null hypothesis and conclude that there is nothing surprising and that there is no evidence for a significant relationship between a predictor and an outcome.
But strong evidence is not dispositive (absolute) evidence. By definition, where p = 0.05, a null hypothesis that is true will be rejected, by chance, 5% of the time. When this happens, it is called a false positive—false-positive evidence for the research hypothesis (false evidence against the null hypothesis). The size of the experiment does not matter. When researchers compute a single p-value, both large and small studies have a 5% chance of producing a false-positive result.
Such studies, by definition, can also produce false negatives—false-negative evidence against the research hypothesis (false evidence for the null hypothesis). In a world of pure science, false positives and false negatives would have equally negative effects on published research. But all the incentives in our summary of the irreproducibility crisis indicate that scientists vastly overproduce false-positive results. We will focus here, therefore, on false positives—which far outnumber false negatives in the published scientific literature.232
We will focus particularly on how and why conducting a large number of statistical tests produces many false positives by chance alone.
Simulating Random p-values
We can illustrate how a large number of statistical tests produce false positives by chance alone through a simulated experiment. We can use a computer to generate 100 pseudo-random numbers between 0 and 1 that mimic p-values and enter them into a 5 x 20 table. (See Figure 10.) These randomly generated p-values should be evenly distributed, with approximately 5 results between 0 and 0.05, 5 between 0.05 and 0.10, and so on—approximately, because a randomly generated sequence of numbers should not produce a perfectly uniform distribution.
In Figure 10, we have simulated a nutritional epidemiology study using a hypothetical single-cohort data set analyzing associations between five individual food components and 20 health outcomes. Remember, these numbers were picked at random.
Figure 10: 100 Simulated p-values
Outcomes | Food 1 | Food 2 | Food 3 | Food 4 | Food 5 |
O 01 | 0.899 | 0.417 | 0.673 | 0.754 | 0.686 |
O 02 | 0.299 | 0.349 | 0.944 | 0.405 | 0.878 |
O 03 | 0.868 | 0.535 | 0.448 | 0.430 | 0.221 |
O 04 | 0.439 | 0.897 | 0.930 | 0.500 | 0.257 |
O 05 | 0.429 | 0.082 | 0.038 | 0.478 | 0.053 |
O 06 | 0.432 | 0.305 | 0.056 | 0.403 | 0.821 |
O 07 | 0.982 | 0.707 | 0.460 | 0.789 | 0.956 |
O 08 | 0.723 | 0.931 | 0.827 | 0.296 | 0.758 |
O 09 | 0.174 | 0.982 | 0.277 | 0.970 | 0.366 |
O 10 | 0.117 | 0.339 | 0.281 | 0.746 | 0.419 |
O 11 | 0.433 | 0.640 | 0.313 | 0.310 | 0.482 |
O 12 | 0.004 | 0.412 | 0.428 | 0.195 | 0.184 |
O 13 | 0.663 | 0.552 | 0.893 | 0.084 | 0.827 |
O 14 | 0.785 | 0.398 | 0.895 | 0.393 | 0.092 |
O 15 | 0.595 | 0.322 | 0.159 | 0.407 | 0.663 |
O 16 | 0.553 | 0.173 | 0.452 | 0.859 | 0.899 |
O 17 | 0.748 | 0.480 | 0.486 | 0.018 | 0.130 |
O 18 | 0.643 | 0.371 | 0.303 | 0.614 | 0.149 |
O 19 | 0.878 | 0.548 | 0.039 | 0.864 | 0.152 |
O 20 | 0.559 | 0.343 | 0.187 | 0.109 | 0.930 |
Each cell in Figure 10 represents a different statistical test applied to associate a predictor (a food component) with an outcome (a health consequence). The Figure displays results of 100 null hypothesis tests analyzing whether each individual food component is positively associated with 20 different outcomes. Each cell represents one out of 100 null hypothesis statistical tests—one test for each of 20 health outcomes, applied to five individual food components. The number in the cell represents the p-value of each individual statistical test.
This simulation contains four p-values that are less than 0.05: 0.004, 0.038, 0.039 and 0.018. In other words, by sheer chance alone, a researcher could write and publish four professional articles based on the four “significant” results (p-values less than 0.05). Researchers are supposed to take account of these pitfalls (chance outcomes). There are standard procedures that can be used to prevent researchers from simply cherry-picking “significant” results.233 But it is all too easy for a researcher to set aside those standard procedures, p-hack, and just report on and write a paper for each result with a nominally significant p-value.
P-hacking by Asking Multiple Questions
As noted above, a standard form of p-hacking is for a researcher to run statistical analyses until a statistically significant result appears—and publish the one (likely spurious) result. When researchers ask hundreds of questions, and when they are free to use any number of statistical models to analyze associations, it is all too easy to engage in this form of p-hacking. In general, research based on multiple analyses of large, complex data sets is especially susceptible to p-hacking, since a researcher can easily produce a p-value < 0.05 by chance alone.234 Research that relies on combining large numbers of questions and computing multiple models is known as Multiple Testing and Multiple Modeling.235
Confirmation bias compounds the difficulties of observing a chance p-value < 0.05. Confirmation bias, frequently triggered by HARKing that falsely conflates exploratory research with confirmatory research, influences researchers so that they are more likely to publish research that confirms a dominant scientific paradigm, such as the association of an air component with a health outcome, and less likely to publish results that contradict a dominant scientific paradigm.
P-value Plots
Now we put together several concepts that we have introduced. When we conduct a null hypothesis statistical test, we can produce a single p-value that falls anywhere in the interval from 0 to 1, and which is considered “statistically significant” in many disciplines when it is less than 0.05. We also know that researchers often look at many questions and compute many models using the same observational data set, and that this allows them to claim that a small p-value produced by chance substantiates a claim to a significant association.
Consider the following example.236 Researchers claimed that, by eating breakfast cereal, a woman is more likely to have a boy baby.237 The researchers conducted a food frequency questionnaire (FFQ) study that asked pregnant women about their consumption of 131 foods at two different time points, one before conception and one just after the estimated date of conception. The FFQ posed a total of 262 questions. The researchers obtained a result with a p-value less than 0.05 and claimed that they had discovered an association between maternal breakfast-cereal consumption and fetal sex ratios. Their procedure made it highly likely that they had simply discovered a false-positive association.
We cannot prove that any one such result is a false positive, absent a series of replication experiments. But we can detect when a given result is likely to be a false positive, drawn from a larger body of questions that indicate randomness rather than a true positive association.
The way to assess a given result is to make a p-value plot of the larger body of results that includes the individual result, and then plot the reported p-values of each of those results. We then use this p-value plot to examine how uniformly the p-values are spread over the interval 0 to 1. We use the following steps to create the p-value plot:
- Rank-order the p-values from smallest to largest.
- Plot the p-values against the integers: 1, 2, 3, …
When we have created the p-value plot, we interpret it like this:
- A p-value plot that forms approximately a 45-degree line (i.e., slope = 1) provides evidence of randomness—a literature that supports the null hypothesis of no significant association.
- A p-value plot that forms approximately a line with a flat/shallow slope < 1, where most of the p-values are small (less than 0.05), provides evidence for a real effect—a literature that supports a statistically significant association.
- A p-value plot that exhibits bilinearity—that divides into two lines—provides evidence of publication bias, p-hacking, and/or HARKing.238
Why does a plotted 45-degree line of p-value results provide evidence of randomness? When a researcher conducts a series of statistical tests to test a hypothesis, and there is no significant association, then the individual results ought to appear anywhere in the interval 0 to 1. When we rank these p-values and plot them against the integers 1, 2, … , they will produce a 45-degree line that depicts a uniform distribution of results. The differences between the individual results, in other words, differ from one another regularly and produce collectively a uniform distribution of results.
Whenever we plot a body of linked p-value results, and the results plot to a 45-degree line, that is evidence that an individual result is the result of a random distribution of results—that even a putatively significant association is really only a fluke result, a false positive, where the evidence as a whole supports the null hypothesis of no significant association.
We may take this as evidence of randomness whether we apply it to:
- a series of individual studies focused on one question,
- a series of tests that emerge by uncontrolled testing of a set of different predictors and different outcomes, or
- a series of meta-analyses.239
The null hypothesis assumption is that there is no significant association. This presumption of a random outcome, of no significant association, must be positively defeated in a hypothesis test in order to make a claim of a significant, surprising result.240 The corollary is that an individual result of a significant association can only be taken as reliable if any body of results to which it belongs also positively defeats the p-value plot of a 45-degree line that depicts a uniform distribution of results.241
Let us return to the research linking breakfast cereal with increased conception of baby boys. That statistical association was drawn from 262 total questions, each of which produced its own p-value. When we plot the reported p-values of all 262 of those questions, in Figure 11 below, the result is a line of slope 1 (approximately).
Figure 11: P-value Plot, 262 P-values, Drawn from Food Frequency Questionnaire, Questions Concerning Boy Baby Conception242
This line supports the presumption of randomness, as a 45-degree line starting at the origin 0,0 would fit the data very well. The small p-value, less than 0.05, registered for the association between breakfast-cereal consumption and boy-baby conception, represents a false-positive finding.
P-value plotting likewise reveals randomness, no significant association, when applied in Figure 12 to a meta-analysis that combined data from 69 questions drawn from 40 observational studies. The claim being evaluated in the meta-analysis was whether long-term exercise training of elderly persons is positively associated with greater mortality and morbidity (increased accidents and falls and hospitalization due to accidents and falls).
Figure 12: P-value Plot, 69 Questions Drawn From 40 Observational Studies, Meta-analysis of Observational Data Sets Analyzing Association Between Elderly Long-term Exercise Training and Mortality and Morbidity Risk243
Figure 12, as Figure 11, plots the p-values as a sloped line from left to right at approximately 45 degrees, and therefore supports the presumption of randomness. Note that Figure 12 contains four p-values less than 0.05, as well as several p-values close to 1.000. The p-values below p = 0.05 are most likely false positives.
These claims are purely statistical. Researchers can, and will, argue that discipline-specific information supports their particular claim for a statistical association—that “relevant biological knowledge,” for example, supports the claim that there truly is an association between breakfast-cereal consumption and boy-baby conception.244
We recognize the possibility that statisticians and disciplinary specialists talk past each other and refuse to engage with the substance of each other’s arguments. But we urge disciplinary specialists, and the public at large, to consider how extraordinarily unlikely it is for a p-value plot indicating randomness to itself be a false positive. The counter-argument that a particular result truly registers a significant association needs to refute the chances against such a 45-degree line appearing if the individual results were not the consequence of selecting false positives for publication.
Such a counter-argument should also consider that p-value plotting does register true effects. We applied the same method to produce a p-value plot in Figure 13 of studies that examined a smoking-lung cancer association.
Figure 13: P-value Plot, 102 Studies, Association of Smoking and Squamous Cell Carcinoma of the Lungs245
In this case, the p-value plot did not form a roughly 45-degree line, with uniform p-value distribution over the interval. Instead it formed an almost horizontal line, with the vast majority of the results well below p = 0.01. Only 3 out of 102 p-values were above p = 0.05. One outlying p-value was just below 0.40—which reminds us that even where there is a true strong relationship, a few studies may produce false negatives. Our p-value plot provides evidence that the studies associating smoking and lung cancer had discovered a true association.
Bilinear P-value Plots
Our method also registers bilinear results (divides into two lines). In Figure 14, we plotted studies that analyze associations between fine particulate matter and the risk of preterm birth or term low birth weight. A 45-degree line, as in Figures 11 and 12, indicates randomness, no effect, and therefore strongly suggests that researchers have indulged in HARKing if they claim a positive effect. A bilinear shape instead suggests the possibility of publication bias, p-hacking, and/or HARKing—although there remains some possibility of a true effect.
Figure 14: P-value Plot, 23 Studies, Association of Fine Particulate Matter (PM2.5) and the Risk of Preterm Birth or Term Low Birth Weight [246]
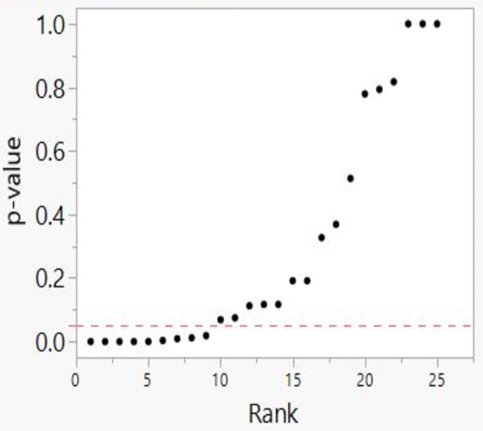
As we shall explain, such a bilinear plot should usually be interpreted as providing evidence that the bias described above has affected a given field, albeit not as strong as the evidence that a 45-degree line provides evidence of no effect. Still, researchers would have good cause to query a claim of an association between fine particulate matter and the risk of preterm birth or term low birth weight, even if a true effect cannot be absolutely ruled out.
Figure 13 demonstrates that our method can detect true associations—it will not come back with a 45-degree line no matter what data you feed into it. When it does detect randomness, as in Figures 11 and 12, the inference is that a particular result is likely to be random, and that the claimed result has failed a statistical test that a true positive body of research passes.
When a p-value plot exhibits bilinearity, as in Figure 14, it provides evidence that there are 1) missing p-values—missing results, which ought to complete the (null) line; and/or 2) p-hacked results, which have driven results down from what they should be to results smaller than the professionally designated level of statistical significance. Bilinearity, in other words, provides evidence that a field has been subject to publication bias—either that negative results have gone into the file drawer or that published results are the result of p-hacking, and/or HARKing.
Our test is useful for assessing the scientific literature precisely because it provides reasonable possibilities for both success and failure.247 We should emphasize that this method is not meant to present an unanswerable disproof of any study or literature to which it is applied. As noted above, the authors of the claim associating maternal breakfast-cereal consumption with altered fetal sex ratios made a counter-argument to our critique, and to the argument for randomness displayed in Figure 11. We urge all scholars and interested citizens to examine these counter-arguments. Scientific discovery proceeds by the scrutiny of such arguments and counter-arguments.248
We claim that our p-value plot method provides a useful test to check claims against the null hypothesis. Any such claims ought as a general rule to survive the test of our method—particularly if they are to be used to influence government policy.
P-value plots are an essential component of the rigorous statistical testing that must now be considered the scientific gold standard. Even meta-analyses exclusively relying on studies of RCTs, which use admirably rigorous study designs,249 can display bilinear p-value plots. P-value plotting provides evidence that while RCT studies may be necessary to produce rigorous science, they are not sufficient unless they have been subjected to equally rigorous statistical testing.
Where government regulatory policy depends on the claim that such positive associations exist, the existence of a bilinear p-value plot provides a very strong argument that a body of literature has not actually proved the existence of an association to the level that justifies government regulation. A bilinear p-value plot provides a good rule of thumb: a government agency has not yet acquired the rigorously tested body of scientific research needed to justify regulation.
P-value plotting isn’t itself a cure-all. The procedure might not be able to tell when an entire literature consists of biased results. P-value plotting cannot detect every form of systematic error. But it is a useful tool, which allows us to detect a strong likelihood that a substantial portion of government regulation has been built on inconsistent science.
We note here that p-value plotting is not the only means available by which to detect publication bias, p-hacking, and HARKing in meta-analyses. Scientists have come up with a broad variety of statistical tests to account for such frailties in base studies as they compute meta-analyses. Unfortunately, publication bias and questionable research procedures in base studies severely degrade the utility of existing means of detection.250 We proffer p-value plotting not as the first means to detect publication bias and p-hacking in meta-analyses, but as a better means than alternatives which have proven ineffective.
Appendix 5: Meta-Analyses: Definition and Use
A meta-analysis is a systematic procedure for statistically combining data from multiple published papers that address a common research question—for example, whether a specific factor is a likely cause or origin of a health outcome such as a stroke or a heart attack. Scientists can conduct meta-analyses relatively easily. Researchers use computer programs to search the published literature; sort quickly through titles, abstracts, and full-texts of papers; and select ca. 10−20 papers from the hundreds to thousands of papers initially identified as candidates for meta-analysis.
The set of papers chosen for a single meta-analysis itself requires careful study so as to select properly comparable and on-topic papers and include all the relevant studies.251 In the well-established cottage industry of meta-analysis studies, a skilled team of 5−15 researchers can turn out one meta-analysis per week.252 Researchers publish approximately 5,000 meta-analysis studies per year.253
Many government agencies now depend upon meta-analyses. The flood of papers on any given topic makes it difficult even for an expert to stay abreast of all the literature, and a meta-analysis provides a convenient way to digest the results of many individual papers. Government agencies also wish to base their policy on a broad spectrum of rigorous, comparable research, rather than just one or a few individual studies. Meta-analyses offer the promise that government agencies are indeed using such research. Meta-analyses also offer what appears to be an impartial protocol that can provide a safeguard against the danger of biased expert judgment.
Yet meta-analyses are not a cure-all. Meta-analyses can themselves be affected by publication bias, and by almost every other form of irreproducibility-crisis research error that affects individual studies.254 For example, when researchers vary meta-analyses’ inclusion and exclusion criteria—the criteria stating which studies to include in a meta-analysis and which to exclude—they can produce wildly varying results.255 In other words, researchers who do not pre-register their inclusion and exclusion criteria can HARK their meta-analyses.
Meta-analyses’ reliability also depends on their base studies’ reliability—and if those have been affected by publication bias or other infirmities (e.g., failure to apply MTMM to control for experiment-wise error), then the meta-analyses they are conducting are no more than Garbage In, Garbage Out (GIGO). Funding bias can affect meta-analyses—and where government agencies are concerned, it is worth emphasizing that government funding can produce substantial funding bias.256
Evaluation
Qualitative study of meta-analyses is a burgeoning field, which should repay further development.257 We will focus here, however, on the quantitative, statistical study of meta-analyses’ validity—an approach made possible by the extraordinary growth in the number of meta-analyses.
When we refer to a research ‘claim’ in our discussion below, we mean that a meta-analysis study makes a claim of a positive statistical association between a factor investigated and an outcome based on combining test statistics from their base studies. As it is a statistical claim being made by the meta-analysis researchers, we can evaluate the reliability of the claim from a statistical point-of-view. We can use p-value plotting to evaluate published meta-analyses, as we did in Figures 11–14, and thereby uncover problems in the way these meta-analyses have been interpreted.
When we plot an approximately 45-degree line, we acquire good evidence for the null hypothesis. When we plot bilinearity, we acquire evidence of publication bias, p-hacking, and/or HARKing—and significant evidence against any claim of a consistent overall positive association between cause and outcome across the studies used in that particular meta-analysis. At the very least, we have acquired evidence that some unidentified covariate complicates the putative relationship.258
We noted above that government agencies rely heavily on meta-analyses to justify regulation. They do not as yet subject these meta-analyses to p-value plotting—and we believe that their failure to do so denies them a very useful tool for assessing the validity of such meta-analyses. P-value plotting that establishes bilinearity does not disprove the meta-analysis. The significant associations could be true; the random results in error. But given the known incentives toward publication bias, p-hacking, and HARKing, bilinearity says we should take meta-analyses’ claims to have detected positive associations with a big grain of salt.
Appendix 6: HARKing: Exploratory Research Disguised as Confirmatory Research
To HARK is to hypothesize after the results are known—to look at the data first and then come up with a hypothesis that provides a statistically significant result.259 Irreproducible research hypotheses produced by HARKing send whole disciplines chasing down rabbit holes, as scientists interpret their follow-up research to conform to a highly tentative piece of exploratory research that was pretending to be confirmatory research.
Scientific advance depends upon scientists maintaining a distinction between exploratory research and confirmatory research, precisely to avoid this mental trap. These two types of research should utilize entirely different procedures. HARKing conflates the two by pretending that a piece of exploratory research has really followed the procedures of confirmatory research.260
Jaeger and Halliday provide useful, brief definitions of exploratory and confirmatory research, and how they differ from one another:
Explicit hypotheses tested with confirmatory research usually do not spring from an intellectual void but instead are often gained through exploratory research. Thus exploratory approaches to research can be used to generate hypotheses that later can be tested with confirmatory approaches. ... The end goal of exploratory research ... is to gain new insights, from which new hypotheses might be developed. ... Confirmatory research proceeds from a series of alternative, a priori hypotheses concerning some topic of interest, followed by the development of a research design (often experimental) to test those hypotheses, the gathering of data, analyses of the data, and ending with the researcher’s inductive inferences. Because most research programs must rely on inductive (rather than deductive) logic ..., none of the alternative hypotheses can be proven to be true; the hypotheses can only be refuted or not refuted. Failing to refute one or more of the alternative hypotheses leads the researcher, then, to gain some measure of confidence in the validity of those hypotheses.261
Exploratory research, in other words, has few predefined hypotheses. Researchers do not at first know precisely what they’re looking for, or even necessarily where to look for it. They “typically generate hypotheses post hoc rather than test a predefined hypothesis.”262 Exploratory studies can easily raise thousands of separate scientific claims,263 and they possess an increased risk of finding false-positive associations.
Confirmatory research “tests predefined hypotheses usually derived from a theory or the results of previous studies that can be used to draw firm and often meaningful conclusions.”264 Confirmatory studies ideally should focus on just one hypothesis, to provide a severe test of its validity. In good confirmatory research, researchers control every significant variable.
When multiple questions are at issue, researchers should use procedures such as Multiple Testing and Multiple Modeling (MTMM) to control for experiment-wise error—the probability that at least one individual claim will register a false positive when you conduct multiple statistical tests.265
Researchers should state the hypothesis clearly, draft the research protocol carefully, and leave as little room for error as possible in execution or interpretation. Properly conducted, confirmatory research is by its nature far less likely to find false positive associations than original research, and conclusions supported by confirmatory research are correspondingly more reliable.
Researchers resort to HARKing—exploratory research that mimics confirmatory research—not only because it can increase their publication rate but also because it can increase their prestige. HARKing scientists can gain the reputation for an overwhelmingly probable research result when all they have really done is set the stage for more follow-on false-positive results or file-drawer negative results.
Another way to define HARKing is that, like p-hacking more generally, it overfits data—it produces a model that conforms to random data.266
HARKing, unfortunately, includes yet wider categories of research. When scientists preregister their research, they specify and publish their research plan in advance. All un-preregistered research can be susceptible to HARKing, as it allows researchers to transform their exploratory research into confirmatory research by looking at their data first and then constructing a hypothesis to fit the data, without informing peer reviewers that this is what they did.267 In general, researchers too frequently fail to make clear distinctions between exploratory and confirmatory research, or to signal transparently to their readers the nature of their own research.268
Appendix 7: Public Health Interventions: Masks (Supporting Information)
Initially, we were interested in showing the number of listings of meta-analysis studies cited in literature related to some aspect of COVID-19. We used the PubMed search engine.269 The search returned 3,256 listings in the National Library of Medicine database. This included 633 listings for 2020, 1,300 listings for 2021, and 1,323 listings thus far for 2022. This is considered an astonishing amount, in that a meta-analysis is a summary of available papers.
7.1 Respiratory virus airborne transmission characteristics
Viruses are one of the smallest known bioaerosols, with a particle diameter ranging from 20 to 300 nm (0.02−0.3 µm).270 The COVID-19 (sars-cov-2) virus has a reported size range of 60−160 nm (0.06−0.16 µm).271 This is similar to the reported size range of influenza respiratory viruses (80–120 nm, 0.08−0.12 µm).272 Rhinovirus—a virus responsible for an estimated 30−35% of all adult colds during cold and flu season (NIH 2009)—is smaller, with a diameter of ~30 nm (0.03 µm).273
Regardless of differing virus sizes, most respiratory viruses are transmitted through secretion fluids during breathing in the form of aerosols (<5 μm) or droplets (>5 μm) rather than isolated viruses.274 RNA fragments from both influenza and COVID-19 viruses have been detected in aerosols ranging from 0.25 to >4 µm.275
When viral-infected human hosts breathe, talk, eat, cough, or sneeze, they emit aerosol particles across a range of sizes,276 and respiratory viruses are in those particles.277 For example, aerosol particles respired from simple breathing are small (size range 0.2 to 0.6 μm), and once emitted can be present in an enclosed setting for several hours.278 Asymptomatic carriers of a virus do not cough and sneeze, and therefore do not expel large respiratory droplets.
Medical masks provide protection against large droplets. However, smaller particulates (aerosols) are less effectively filtered. Aerosol particles between ∼0.1 and 0.5 μm are not easily filtered out of the surrounding air by any physical mechanism, and there continues to be uncertainty about use of conventional medical masks to separate (remove) these small aerosol particles.279
Belkin states that there are a couple of ways in which virus-laden aerosol particles can contribute to the infection of a mask-wearer when these particles are present in the breathing zone of the mask-wearer.280 These include aerosol-particle penetration through a mask during inhalation and the inhalation of air-containing aerosol particles from the side of a mask due to incorrect wear, increased mask resistance, or poor string tension.281
A mask-wearer breathing out moist air increases mask resistance.282 Simple breathing has been shown to release up to 7,200 aerosol particles per liter of exhaled air.283 While this can reduce aerosol penetration through the mask, it worsens the problem of inhaling virus-laden aerosols from the side of the mask.284
7.2 Study selection
The randomized controlled trial (RCT) is recognized as a ‘gold standard’ for assessing the efficacy of an intervention.285 For this evaluation, we were interested in “meta-analysis” or “systematic review” studies of RCTs investigating community medical-mask use for the prevention of viral infection. The focus in this evaluation was on influenza and COVID-19 viruses because of their similar size ranges; keeping in mind that it is not the virus itself but the airborne transmission of aerosols or droplets containing viruses that is important for infection.
Another distinction we make in this evaluation is the nature of the outcome for assessing the potential benefit of mask use. Numerous types of outcome measures have been used in mask−viral infection RCT studies: e.g., medical diagnosis of viral illness, self-reported symptoms of viral illness, and lab-confirmed diagnosis of viral illness.286 We excluded data from studies based on self-reported symptoms of viral illness because of awareness bias.
Awareness bias is the tendency of a study participant to self-report a symptom or effect (e.g., a sickness or disease) because of concerns arising from prior knowledge of an environmental hazard that may cause the symptom.287 Participants in a study, where self-reporting is used to capture outcome measures, tend to overestimate their symptoms because of awareness bias.
The perception of exposure, causal beliefs and concerns, and media coverage have a role in study participants self-reporting symptoms.288 Separating a true biological effect from reporting that is increased through awareness bias is a problem in communities where study participants are aware of their potential exposure.289
Marcon et al. recommended using objective health outcomes to rule out awareness bias in populations potentially exposed to environmental hazards.290 Self-reported symptoms of viral illness cannot be considered objective unless they can be corroborated with other, more credible outcome measures (i.e., laboratory confirmation), as such objectively measured outcomes are not influenced by awareness bias.291
We used two online databases—the Cochrane Central Register of Controlled Trials (CENTRAL) and PubMed—to identify eligible studies. We searched these databases for meta-analyses or systematic reviews of randomized controlled trials investigating medical face-mask use and influenza or COVID-19 (sars-cov-2) infections published from January 1, 2020, to December 7, 2022.292
We identified a potentially eligible systematic review in gray literature during online searches. The CATO Institute (Washington, DC) published this review during the January 1, 2020, to December 7, 2022, period. We did not capture this review by searching the CENTRAL or PubMed databases. It examined RCTs of medical-mask use and viral (including influenza and COVID-19) infections.
We read titles and full abstracts online for each study we identified through the searches. Based upon this, we then downloaded and read electronic copies of eligible meta-analysis or systematic review studies. We used the following criteria to determine the eligibility of studies for the evaluation:
- Base studies were randomized controlled trials (RCTs) or cluster RCTs.
- Meta-analysis or systematic review.
- Compared the efficacy of medical masks with not wearing masks. We excluded studies that did not specify mask type used or present isolated outcomes for individual mask types.
- Included influenza and/or COVID-19 (sars-cov-2) viruses. We excluded studies that did not present isolated outcomes for these viruses.
- Intervention and control groups included community participants. We excluded studies that only involved workers in healthcare settings or that did not present isolated outcomes for community participants.
- Included credible outcome measures, i.e., medical diagnosis of viral illness or lab-confirmed diagnosis of viral illness.
7.3 Search Results – Cochrane Central Register of Controlled Trials (CENTRAL) and PubMed
Cochrane Central Register of Controlled Trials (CENTRAL) search results (performed December 12, 2022)
Eligible studies that met search criteria: #11
1 Universal screening for SARS‐CoV‐2 infection: a rapid review
Meera Viswanathan, Leila Kahwati, Beate Jahn, et al.
https://www.cochranelibrary.com/cdsr/doi/10.1002/14651858.CD013718/full
2 Antibody tests for identification of current and past infection with SARS‐CoV‐2
Tilly Fox, Julia Geppert, Jacqueline Dinnes, et al., Cochrane COVID-19 Diagnostic Test Accuracy Group
https://www.cochranelibrary.com/cdsr/doi/10.1002/14651858.CD013652.pub2/full
3 Rapid, point‐of‐care antigen tests for diagnosis of SARS‐CoV‐2 infection
Jacqueline Dinnes, Pawana Sharma, Sarah Berhane, et al., Cochrane COVID-19 Diagnostic Test Accuracy Group
https://www.cochranelibrary.com/cdsr/doi/10.1002/14651858.CD013705.pub3/full
4 SARS‐CoV‐2‐neutralising monoclonal antibodies for treatment of COVID‐19
Nina Kreuzberger, Caroline Hirsch, Khai Li Chai, et al.
https://www.cochranelibrary.com/cdsr/doi/10.1002/14651858.CD013825.pub2/full
5 Non‐pharmacological measures implemented in the setting of long‐term care facilities to prevent SARS‐CoV‐2 infections and their consequences: a rapid review
Jan M Stratil, Renke L Biallas, Jacob Burns, et al.
https://www.cochranelibrary.com/cdsr/doi/10.1002/14651858.CD015085.pub2/full
6 SARS‐CoV‐2‐neutralising monoclonal antibodies to prevent COVID‐19
Caroline Hirsch, Yun Soo Park, Vanessa Piechotta, et al.
https://www.cochranelibrary.com/cdsr/doi/10.1002/14651858.CD014945.pub2/full
7 Chloroquine or hydroxychloroquine for prevention and treatment of COVID‐19
Bhagteshwar Singh, Hannah Ryan, Tamara Kredo, et al.
https://www.cochranelibrary.com/cdsr/doi/10.1002/14651858.CD013587.pub2/full
8 Remdesivir for the treatment of COVID‐19
Kelly Ansems, Felicitas Grundeis, Karolina Dahms, et al.
https://www.cochranelibrary.com/cdsr/doi/10.1002/14651858.CD014962/full
9 Ivermectin for preventing and treating COVID‐19
Maria Popp, Stefanie Reis, Selina Schießer, et al.
https://www.cochranelibrary.com/cdsr/doi/10.1002/14651858.CD015017.pub3/full
10 Measures implemented in the school setting to contain the COVID‐19 pandemic
Shari Krishnaratne, Hannah Littlecott, Kerstin Sell, et al.
https://www.cochranelibrary.com/cdsr/doi/10.1002/14651858.CD015029/full
11 Physical interventions to interrupt or reduce the spread of respiratory viruses
Tom Jefferson, Chris B Del Mar, Liz Dooley, et al.
https://www.cochranelibrary.com/cdsr/doi/10.1002/14651858.CD006207.pub5/full
12 Colchicine for the treatment of COVID‐19
Agata Mikolajewska, Anna-Lena Fischer, Vanessa Piechotta, et al.
https://www.cochranelibrary.com/cdsr/doi/10.1002/14651858.CD015045/full
13 Routine laboratory testing to determine if a patient has COVID‐19
Inge Stegeman, Eleanor A Ochodo, Fatuma Guleid, et al., Cochrane COVID-19 Diagnostic Test Accuracy Group
https://www.cochranelibrary.com/cdsr/doi/10.1002/14651858.CD013787/full
14 Thoracic imaging tests for the diagnosis of COVID‐19
Sanam Ebrahimzadeh, Nayaar Islam, Haben Dawit, et al., Cochrane COVID-19 Diagnostic Test Accuracy Group
https://www.cochranelibrary.com/cdsr/doi/10.1002/14651858.CD013639.pub5/full
15 Use of antimicrobial mouthwashes (gargling) and nasal sprays by healthcare workers to protect them when treating patients with suspected or confirmed COVID‐19 infection
Martin J Burton, Janet E Clarkson, Beatriz Goulao, et al.
https://www.cochranelibrary.com/cdsr/doi/10.1002/14651858.CD013626.pub2/full
16 Janus kinase inhibitors for the treatment of COVID‐19
Andre Kramer, Carolin Prinz, Falk Fichtner, et al.
https://www.cochranelibrary.com/cdsr/doi/10.1002/14651858.CD015209/full
17 Signs and symptoms to determine if a patient presenting in primary care or hospital outpatient settings has COVID‐19
Thomas Struyf, Jonathan J Deeks, Jacqueline Dinnes, et al., Cochrane COVID-19 Diagnostic Test Accuracy Group
https://www.cochranelibrary.com/cdsr/doi/10.1002/14651858.CD013665.pub3/full
18 Convalescent plasma or hyperimmune immunoglobulin for people with COVID‐19: a living systematic review
Vanessa Piechotta, Claire Iannizzi, Khai Li Chai, et al.
https://www.cochranelibrary.com/cdsr/doi/10.1002/14651858.CD013600.pub4/full
19 Anticoagulants for people hospitalised with COVID‐19
Ronald LG Flumignan, Vinicius T Civile, Jéssica Dantas de Sá Tinôco, et al.
https://www.cochranelibrary.com/cdsr/doi/10.1002/14651858.CD013739.pub2/full
20 Digital contact tracing technologies in epidemics: a rapid review
Andrew Anglemyer, Theresa HM Moore, Lisa Parker, et al.
https://www.cochranelibrary.com/cdsr/doi/10.1002/14651858.CD013699/full
21 International travel‐related control measures to contain the COVID‐19 pandemic: a rapid review
Jacob Burns, Ani Movsisyan, Jan M Stratil, et al.
https://www.cochranelibrary.com/cdsr/doi/10.1002/14651858.CD013717.pub2/full
22 Quarantine alone or in combination with other public health measures to control COVID‐19: a rapid review
Barbara Nussbaumer-Streit, Verena Mayr, Andreea Iulia Dobrescu, et al.
https://www.cochranelibrary.com/cdsr/doi/10.1002/14651858.CD013574.pub2/full
23 Interventions to support the resilience and mental health of frontline health and social care professionals during and after a disease outbreak, epidemic or pandemic: a mixed methods systematic review
Alex Pollock, Pauline Campbell, Joshua Cheyne, et al.
https://www.cochranelibrary.com/cdsr/doi/10.1002/14651858.CD013779/full
24 Personal protective equipment for preventing highly infectious diseases due to exposure to contaminated body fluids in healthcare staff
Jos H Verbeek, Blair Rajamaki, Sharea Ijaz, et al.
https://www.cochranelibrary.com/cdsr/doi/10.1002/14651858.CD011621.pub5/full
25 Antibiotics for the treatment of COVID‐19
Maria Popp, Miriam Stegemann, Manuel Riemer, et al.
https://www.cochranelibrary.com/cdsr/doi/10.1002/14651858.CD015025/full
26 Barriers and facilitators to healthcare workers’ adherence with infection prevention and control (IPC) guidelines for respiratory infectious diseases: a rapid qualitative evidence synthesis
Catherine Houghton, Pauline Meskell, Hannah Delaney, et al.
https://www.cochranelibrary.com/cdsr/doi/10.1002/14651858.CD013582/full
27 Interleukin‐6 blocking agents for treating COVID‐19: a living systematic review
Lina Ghosn, Anna Chaimani, Theodoros Evrenoglou, et al.
https://www.cochranelibrary.com/cdsr/doi/10.1002/14651858.CD013881/full
28 Interventions to reduce contaminated aerosols produced during dental procedures for preventing infectious diseases
Sumanth Kumbargere Nagraj, Prashanti Eachempati, Martha Paisi, et al.
https://www.cochranelibrary.com/cdsr/doi/10.1002/14651858.CD013686.pub2/full
29 Video calls for reducing social isolation and loneliness in older people: a rapid review
Chris Noone, Jenny McSharry, Mike Smalle, et al.
https://www.cochranelibrary.com/cdsr/doi/10.1002/14651858.CD013632/full
30 Hand cleaning with ash for reducing the spread of viral and bacterial infections: a rapid review
Asger Sand Paludan-Müller, Kim Boesen, Irma Klerings, et al.
https://www.cochranelibrary.com/cdsr/doi/10.1002/14651858.CD013597/full
31 Probiotics for preventing acute upper respiratory tract infections
Yunli Zhao, Bi Rong Dong, Qiukui Hao
https://www.cochranelibrary.com/cdsr/doi/10.1002/14651858.CD006895.pub4/full
32 Positioning for acute respiratory distress in hospitalised infants and children
Abhishta P Bhandari, Daniel A Nnate, Lenny Vasanthan, et al.
https://www.cochranelibrary.com/cdsr/doi/10.1002/14651858.CD003645.pub4/full
33 Exercise versus no exercise for the occurrence, severity, and duration of acute respiratory infections
Antonio Jose Grande, Justin Keogh, Valter Silva, et al.
https://www.cochranelibrary.com/cdsr/doi/10.1002/14651858.CD010596.pub3/full
34 Topical antibiotic prophylaxis to reduce respiratory tract infections and mortality in adults receiving mechanical ventilation
Silvia Minozzi, Silvia Pifferi, Luca Brazzi, et al.
https://www.cochranelibrary.com/cdsr/doi/10.1002/14651858.CD000022.pub4/full
35 Humidification of indoor air for preventing or reducing dryness symptoms or upper respiratory infections in educational settings and at the workplace
Katarzyna Byber, Thomas Radtke, Dan Norbäck, et al.
https://www.cochranelibrary.com/cdsr/doi/10.1002/14651858.CD012219.pub2/full
36 Biomarkers as point‐of‐care tests to guide prescription of antibiotics in people with acute respiratory infections in primary care
Siri Aas Smedemark, Rune Aabenhus, Carl Llor, et al.
https://www.cochranelibrary.com/cdsr/doi/10.1002/14651858.CD010130.pub3/full
37 Heliox for croup in children
Irene Moraa, Nancy Sturman, Treasure M McGuire, et al.
https://www.cochranelibrary.com/cdsr/doi/10.1002/14651858.CD006822.pub6/full
38 Antibiotic treatment for Stenotrophomonas maltophilia in people with cystic fibrosis
Reshma Amin, Nikki Jahnke, Valerie Waters
https://www.cochranelibrary.com/cdsr/doi/10.1002/14651858.CD009249.pub5/full
39 Chest physiotherapy for pneumonia in adults
Xiaomei Chen, Jiaojiao Jiang, Renjie Wang, et al.
https://www.cochranelibrary.com/cdsr/doi/10.1002/14651858.CD006338.pub4/full
40 Antibiotic therapy versus no antibiotic therapy for children aged 2 to 59 months with WHO‐defined non‐severe pneumonia and wheeze
Zohra S Lassi, Zahra Ali Padhani, Jai K Das, et al.
https://www.cochranelibrary.com/cdsr/doi/10.1002/14651858.CD009576.pub3/full
41 Continuous positive airway pressure (CPAP) for acute bronchiolitis in children
Kana R Jat, Jeanne M Dsouza, Joseph L Mathew
https://www.cochranelibrary.com/cdsr/doi/10.1002/14651858.CD010473.pub4/full
42 Magnesium sulphate for treating acute bronchiolitis in children up to two years of age
Sudha Chandelia, Dinesh Kumar, Neelima Chadha, et al.
https://www.cochranelibrary.com/cdsr/doi/10.1002/14651858.CD012965.pub2/full
43 Vaccines for measles, mumps, rubella, and varicella in children
Carlo Di Pietrantonj, Alessandro Rivetti, Pasquale Marchione, et al.
https://www.cochranelibrary.com/cdsr/doi/10.1002/14651858.CD004407.pub5/full
44 Pneumococcal conjugate vaccines for preventing acute otitis media in children
Joline LH de Sévaux, Roderick P Venekamp, Vittoria Lutje, et al.
https://www.cochranelibrary.com/cdsr/doi/10.1002/14651858.CD001480.pub6/full
45 Zinc supplementation for the promotion of growth and prevention of infections in infants less than six months of age
Zohra S Lassi, Jaameeta Kurji, Cristieli Sérgio de Oliveira, et al.
https://www.cochranelibrary.com/cdsr/doi/10.1002/14651858.CD010205.pub2/full
46 Parenteral versus enteral fluid therapy for children hospitalised with bronchiolitis
Peter J Gill, Mohammed Rashidul Anwar, Emily Kornelsen, et al.
https://www.cochranelibrary.com/cdsr/doi/10.1002/14651858.CD013552.pub2/full
47 Corticosteroids as standalone or add‐on treatment for sore throat
Simone de Cassan, Matthew J Thompson, Rafael Perera, et al.
https://www.cochranelibrary.com/cdsr/doi/10.1002/14651858.CD008268.pub3/full
48 Antibiotic treatment for nontuberculous mycobacteria lung infection in people with cystic fibrosis
Valerie Waters, Felix Ratjen
https://www.cochranelibrary.com/cdsr/doi/10.1002/14651858.CD010004.pub5/full
49 Antibiotic treatment for Burkholderia cepacia complex in people with cystic fibrosis experiencing a pulmonary exacerbation
Robert Lord, Andrew M Jones, Alex Horsley
https://www.cochranelibrary.com/cdsr/doi/10.1002/14651858.CD009529.pub4/full
50 Vitamin C supplementation for prevention and treatment of pneumonia
Zahra Ali Padhani, Zorays Moazzam, Alina Ashraf, et al.
https://www.cochranelibrary.com/cdsr/doi/10.1002/14651858.CD013134.pub3/full
51 Antibiotics for treatment of sore throat in children and adults
Anneliese Spinks, Paul P Glasziou, Chris B Del Mar
https://www.cochranelibrary.com/cdsr/doi/10.1002/14651858.CD000023.pub5/full
52 Xpert Ultra versus Xpert MTB/RIF for pulmonary tuberculosis and rifampicin resistance in adults with presumptive pulmonary tuberculosis
Jerry S Zifodya, Jonah S Kreniske, Ian Schiller, et al.
https://www.cochranelibrary.com/cdsr/doi/10.1002/14651858.CD009593.pub5/full
53 Xpert MTB/RIF Ultra and Xpert MTB/RIF assays for extrapulmonary tuberculosis and rifampicin resistance in adults
Mikashmi Kohli, Ian Schiller, Nandini Dendukuri, et al.
https://www.cochranelibrary.com/cdsr/doi/10.1002/14651858.CD012768.pub3/full
54 Rapid diagnostic tests for plague
Sophie Jullien, Harsha A Dissanayake, Marty Chaplin
https://www.cochranelibrary.com/cdsr/doi/10.1002/14651858.CD013459.pub2/full
55 Xpert MTB/RIF Ultra assay for tuberculosis disease and rifampicin resistance in children
Alexander W Kay, Tara Ness, Sabine E Verkuijl, et al.
https://www.cochranelibrary.com/cdsr/doi/10.1002/14651858.CD013359.pub3/full
56 Interleukin‐1 blocking agents for treating COVID‐19
Mauricia Davidson, Sonia Menon, Anna Chaimani, et al.
https://www.cochranelibrary.com/cdsr/doi/10.1002/14651858.CD015308/full
57 COVID‐19 and its cardiovascular effects: a systematic review of prevalence studies
Pierpaolo Pellicori, Gemina Doolub, Chih Mun Wong, et al.
https://www.cochranelibrary.com/cdsr/doi/10.1002/14651858.CD013879/full
58 Interventions for the treatment of persistent post‐COVID‐19 olfactory dysfunction
Lisa O'Byrne, Katie E Webster, Samuel MacKeith, et al.
https://www.cochranelibrary.com/cdsr/doi/10.1002/14651858.CD013876.pub3/full
59 Interventions for the prevention of persistent post‐COVID‐19 olfactory dysfunction
Katie E Webster, Lisa O'Byrne, Samuel MacKeith, et al.
https://www.cochranelibrary.com/cdsr/doi/10.1002/14651858.CD013877.pub3/full
60 Systemic corticosteroids for the treatment of COVID‐19: Equity‐related analyses and update on evidence
Carina Wagner, Mirko Griesel, Agata Mikolajewska, et al.
https://www.cochranelibrary.com/cdsr/doi/10.1002/14651858.CD014963.pub2/full
61 Healthcare workers’ perceptions and experiences of communicating with people over 50 years of age about vaccination: a qualitative evidence synthesis
Claire Glenton, Benedicte Carlsen, Simon Lewin, et al.
https://www.cochranelibrary.com/cdsr/doi/10.1002/14651858.CD013706.pub2/full
PubMed search results (performed December 12, 2022)
Eligible studies that met search criteria: #’s 7, 13, 16, 18, 26, 52
1 Bundgaard H, Bundgaard JS, Raaschou-Pedersen DET, et al. Effectiveness of Adding a Mask Recommendation to Other Public Health Measures to Prevent SARS-CoV-2 Infection in Danish Mask Wearers: A Randomized Controlled Trial. Ann Intern Med. 2021 Mar;174(3):335–343. https://doi.org/10.7326/M20-6817
2 Chu DK, Akl EA, Duda S, Solo K, et al., COVID-19 Systematic Urgent Review Group Effort (SURGE) study authors. Physical distancing, face masks, and eye protection to prevent person-to-person transmission of SARS-CoV-2 and COVID-19: a systematic review and meta-analysis. The Lancet. 2020 Jun 27;395(10242):1973–1987. https://doi.org/10.1016/S0140-6736(20)31142-9
3 Li Y, Liang M, Gao L, et al. Face masks to prevent transmission of COVID-19: A systematic review and meta-analysis. Am J Infect Control. 2021 Jul;49(7):900–906. https://doi.org/10.1016/j.ajic.2020.12.007
4 Liang M, Gao L, Cheng C, et al. Efficacy of face mask in preventing respiratory virus transmission: A systematic review and meta-analysis. Travel Med Infect Dis. 2020 Jul-Aug;36:101751. https://doi.org/10.1016/j.tmaid.2020.101751
5 Hemmer CJ, Hufert F, Siewert S, et al. Protection From COVID-19: The Efficacy of Face Masks. Dtsch Arztebl Int. 2021 Feb 5;118(5):59–65. https://doi.org/10.3238/arztebl.m2021.0119
6 Candevīr A, Üngör C, Çīzmecī Şenel F, et al. How efficient are facial masks against COVID-19? Evaluating the mask use of various communities one year into the pandemic. Turk J Med Sci. 2021 Dec 17;51(SI-1):3238–3245. https://doi.org/10.3906/sag-2106-190
7 Tran TQ, Mostafa EM, Tawfik GM, et al. Efficacy of face masks against respiratory infectious diseases: a systematic review and network analysis of randomized-controlled trials. J Breath Res. 2021 Sep 13;15(4). https://doi.org/10.1088/1752-7163/ac1ea5
8 Bartoszko JJ, Farooqi MAM, Alhazzani W, et al. Medical masks vs N95 respirators for preventing COVID-19 in healthcare workers: A systematic review and meta-analysis of randomized trials. Influenza Other Respir Viruses. 2020 Jul;14(4):365–373. https://doi.org/10.1111/irv.12745
9 MacIntyre CR, Chughtai AA. A rapid systematic review of the efficacy of face masks and respirators against coronaviruses and other respiratory transmissible viruses for the community, healthcare workers and sick patients. Int J Nurs Stud. 2020 Aug;108:103629. https://doi.org/10.1016/j.ijnurstu.2020.103629
10 Bundgaard H, Bundgaard JS, Raaschou-Pedersen DET, et al. Face masks for the prevention of COVID-19 - Rationale and design of the randomised controlled trial DANMASK-19. Dan Med J. 2020 Aug 18;67(9):A05200363. https://pubmed.ncbi.nlm.nih.gov/32829745/.
11 Long Y, Hu T, Liu L, et al. Effectiveness of N95 respirators versus surgical masks against influenza: A systematic review and meta-analysis. J Evid Based Med. 2020 May;13(2):93–101. https://doi.org/10.1111/jebm.12381
12 Chou R, Dana T, Jungbauer R, et al. Masks for Prevention of Respiratory Virus Infections, Including SARS-CoV-2, in Health Care and Community Settings: A Living Rapid Review. Ann Intern Med. 2020 Oct 6;173(7):542–555. https://doi.org/10.7326/M20-3213
13 Nanda A, Hung I, Kwong A, et al. Efficacy of surgical masks or cloth masks in the prevention of viral transmission: Systematic review, meta-analysis, and proposal for future trial. J Evid Based Med. 2021 May;14(2):97–111. https://doi.org/10.1111/jebm.12424
14 Abboah-Offei M, Salifu Y, Adewale B, et al. A rapid review of the use of face mask in preventing the spread of COVID-19. Int J Nurs Stud Adv. 2021 Nov;3:100013. https://doi.org/10.1016/j.ijnsa.2020.100013
15 Coclite D, Napoletano A, Gianola S, et al. Face Mask Use in the Community for Reducing the Spread of COVID-19: A Systematic Review. Front Med (Lausanne). 2021 Jan 12;7:594269. https://doi.org/10.3389/fmed.2020.594269
16 Kim MS, Seong D, Li H, Chung SK, et al. Comparative effectiveness of N95, surgical or medical, and non-medical facemasks in protection against respiratory virus infection: A systematic review and network meta-analysis. Rev Med Virol. 2022 Sep;32(5):e2336. https://doi.org/10.1002/rmv.2336
17 Loeb M, Bartholomew A, Hashmi M, et al. Medical Masks Versus N95 Respirators for Preventing COVID-19 Among Health Care Workers: A Randomized Trial. Ann Intern Med. 2022 Dec;175(12):1629–1638. https://doi.org/10.7326/M22-1966
18 Xiao J, Shiu EYC, Gao H, et al. Nonpharmaceutical Measures for Pandemic Influenza in Nonhealthcare Settings—Personal Protective and Environmental Measures. Emerg Infect Dis. 2020 May;26(5):967–975. https://doi.org/10.3201/eid2605.190994
19 Daoud AK, Hall JK, Petrick H, et al. The Potential for Cloth Masks to Protect Health Care Clinicians From SARS-CoV-2: A Rapid Review. Ann Fam Med. 2021 Jan-Feb;19(1):55–62. https://doi.org/10.1370/afm.2640
20 Muller SM. Masks, mechanisms and Covid-19: the limitations of randomized trials in pandemic policymaking. Hist Philos Life Sci. 2021 Mar 25;43(2):43. https://doi.org/10.1007/s40656-021-00403-9
21 Li Y, Wei Z, Zhang J, et al. Wearing masks to reduce the spread of respiratory viruses: a systematic evidence mapping. Ann Transl Med. 2021 May;9(9):811. https://doi.org/10.21037/atm-20-6745
22 Pearce N, Vandenbroucke JP. Arguments about face masks and Covid-19 reflect broader methodologic debates within medical science. Eur J Epidemiol. 2021 Feb;36(2):143–147. https://doi.org/10.1007/s10654-021-00735-7
23 Fortaleza CR, De Souza LDR, Rúgolo JM, et al. COVID-19: What we talk about when we talk about masks. Rev Soc Bras Med Trop. 2020 Nov 6;53:e20200527. https://doi.org/10.1590/0037-8682-0527-2020
24 Laine C, Goodman SN, Guallar E. The Role of Masks in Mitigating the SARS-CoV-2 Pandemic: Another Piece of the Puzzle. Ann Intern Med. 2021 Mar;174(3):419–420. https://doi.org/10.7326/M20-7448
25 Brainard J, Jones NR, Lake IR, et al. Community use of face masks and similar barriers to prevent respiratory illness such as COVID-19: a rapid scoping review. Euro Surveill. 2020 Dec;25(49):2000725. https://doi.org/10.2807/1560-7917.ES.2020.25.49.2000725
26 Aggarwal N, Dwarakanathan V, Gautam N, et al. Facemasks for prevention of viral respiratory infections in community settings: A systematic review and meta-analysis. Indian J Public Health. 2020 Jun;64(Supplement):S192-S200. https://pubmed.ncbi.nlm.nih.gov/32496254/.
27 Baier M, Knobloch MJ, Osman F, et al. Effectiveness of Mask-Wearing on Respiratory Illness Transmission in Community Settings: A Rapid Review. Disaster Med Public Health Prep. 2022 Mar 7:1–8. https://doi.org/10.1017/dmp.2021.369
28 Rowan NJ, Moral RA. Disposable face masks and reusable face coverings as non-pharmaceutical interventions (NPIs) to prevent transmission of SARS-CoV-2 variants that cause coronavirus disease (COVID-19): Role of new sustainable NPI design innovations and predictive mathematical modelling. Sci Total Environ. 2021 Jun 10;772:145530. https://doi.org/10.1016/j.scitotenv.2021.145530
29 Hirt J, Janiaud P, Hemkens LG. Randomized trials on non-pharmaceutical interventions for COVID-19: a scoping review. BMJ Evid Based Med. 2022 Dec;27(6):334–344. https://doi.org/10.1136/bmjebm-2021-111825
30 Chen Y, Wang Y, Quan N, et al. Associations Between Wearing Masks and Respiratory Viral Infections: A Meta-Analysis and Systematic Review. Front Public Health. 2022 Apr 27;10:874693. https://doi.org/10.3389/fpubh.2022.874693
31 Lehnert B, Herold J, Blaurock M, et al. Reliability of the Acoustic Voice Quality Index AVQI and the Acoustic Breathiness Index (ABI) when wearing CoViD-19 protective masks. Eur Arch Otorhinolaryngol. 2022 Sep;279(9):4617–4621. https://doi.org/10.1007/s00405-022-07417-4
32 Dugré N, Ton J, Perry D, et al. Masks for prevention of viral respiratory infections among health care workers and the public: PEER umbrella systematic review. Can Fam Physician. 2020 Jul;66(7):509-517. https://pubmed.ncbi.nlm.nih.gov/32675098/.
33 Wang H, Chen MB, Cui WY, et al. The efficacy of masks for influenza-like illness in the community: A protocol for systematic review and meta-analysis. Medicine (Baltimore). 2020 Jun 5;99(23):e20525. https://doi.org/10.1097/MD.0000000000020525
34 Yang HJ, Yoon H, Kang SY, et al. Respiratory Protection Effect of Ear-loop-type KF94 Masks according to the Wearing Method in COVID-19 Pandemic: a Randomized, Open-label Study. J Korean Med Sci. 2021 Jul 19;36(28):e209. https://doi.org/10.3346/jkms.2021.36.e209
35 Egan M, Acharya A, Sounderajah V, et al. Evaluating the effect of infographics on public recall, sentiment and willingness to use face masks during the COVID-19 pandemic: A randomised internet-based questionnaire study. BMC Public Health. 2021 Feb 17;21(1):367. https://doi.org/10.1186/s12889-021-10356-0
36 Karam C, Zeeni C, Yazbeck-Karam V, et al. Respiratory Adverse Events After LMA® Mask Removal in Children: A Randomized Trial Comparing Propofol to Sevoflurane. Anesth Analg. 2023 Jan 1;136(1):25–33. https://doi.org/10.1213/ANE.0000000000005945
37 Jackson AR, Hull JH, Hopker JG, et al. The impact of a heat and moisture exchange mask on respiratory symptoms and airway response to exercise in asthma. ERJ Open Res. 2020 Jun 22;6(2):00271-2019. https://doi.org/10.1183/23120541.00271-2019
38 Turkia M. The History of Methylprednisolone, Ascorbic Acid, Thiamine, and Heparin Protocol and I-MASK+ Ivermectin Protocol for COVID-19. Cureus. 2020 Dec 31;12(12):e12403. https://doi.org/10.7759/cureus.12403
39 Spang RP, Pieper K. The tiny effects of respiratory masks on physiological, subjective, and behavioral measures under mental load in a randomized controlled trial. Sci Rep. 2021 Oct 1;11(1):19601. https://doi.org/10.1038/s41598-021-99100-7
40 Brian MS, Carmichael RD, Berube FR, et al. The effects of a respiratory training mask on steady-state oxygen consumption at rest and during exercise. Physiol Int. 2022 May 16. https://doi.org/10.1556/2060.2022.00176
41 Tian L, Liu Y, Wei X, et al. A systematic review and meta-analysis of different mask ventilation schemes on management of general anesthesia in patients with respiratory failure. Ann Palliat Med. 2021 Nov;10(11):11587-11597. https://doi.org/10.21037/apm-21-2709
42 Regmi K, Lwin CM. Impact of non-pharmaceutical interventions for reducing transmission of COVID-19: a systematic review and meta-analysis protocol. BMJ Open. 2020 Oct 22;10(10):e041383. https://doi.org/10.1136/bmjopen-2020-041383
43 Li J, Qiu Y, Zhang Y, et al. Protective efficient comparisons among all kinds of respirators and masks for health-care workers against respiratory viruses: A PRISMA-compliant network meta-analysis. Medicine (Baltimore). 2021 Aug 27;100(34):e27026. https://doi.org/10.1097/MD.0000000000027026
44 Cirit Ekiz B, Köksal N, Tuna T, et al. Comparison of full-face and oronasal mask effectiveness in hypercapnic respiratory failure patients with non-invasive mechanical ventilation. Tuberk Toraks. 2022 Jun;70(2):157–165. English. https://doi.org/10.5578/tt.20229806
45 Thota B, Samantaray A, Vengamma B, et al. A randomised controlled trial of high-flow nasal oxygen versus non-rebreathing oxygen face mask therapy in acute hypoxaemic respiratory failure. Indian J Anaesth. 2022 Sep;66(9):644–650. https://doi.org/10.4103/ija.ija_507_22
46 Duong-Quy S, Ngo-Minh X, Tang-Le-Quynh T, et al. The use of exhaled nitric oxide and peak expiratory flow to demonstrate improved breathability and antimicrobial properties of novel face mask made with sustainable filter paper and Folium Plectranthii amboinicii oil: additional option for mask shortage during COVID-19 pandemic. Multidiscip Respir Med. 2020 Jun 1;15(1):664. https://doi.org/10.4081/mrm.2020.664
47 Toprak E, Bulut AN. The effect of mask use on maternal oxygen saturation in term pregnancies during the COVID-19 process. J Perinat Med. 2020 Nov 26;49(2):148–152. https://doi.org/10.1515/jpm-2020-0422
48 Ünal E, Özdemir A. The Effect of Correct Handwashing and Mask Wearing Training on Cardiac Patients' COVID-19 Fear and Anxiety. J Community Health Nurs. 2022 Apr-Jun;39(2):71–89. https://doi.org/10.1080/07370016.2022.2058201
49 Coelho SG, Segovia A, Anthony SJ, et al. Return to school and mask-wearing in class during the COVID-19 pandemic: Student perspectives from a school simulation study. Paediatr Child Health. 2022 May 5;27(Suppl 1):S15–S21. https://doi.org/10.1093/pch/pxab102
50 Felinska EA, Chen ZW, Fuchs TE, et al. Surgical Performance Is Not Negatively Impacted by Wearing a Commercial Full-Face Mask with Ad Hoc 3D-Printed Filter Connection as a Substitute for Personal Protective Equipment during the COVID-19 Pandemic: A Randomized Controlled Cross-Over Trial. J Clin Med. 2021 Feb 2;10(3):550. https://doi.org/10.3390/jcm10030550
51 Graham F. Daily briefing: Masks work against COVID, finds a huge randomized trial. Nature. 2021 Sep 2. https://doi.org/10.1038/d41586-021-02415-8
52 Ollila HM, Partinen M, Koskela J, et al. Face masks to prevent transmission of respiratory infections: Systematic review and meta-analysis of randomized controlled trials on face mask use. PLOS One. 2022 Dec 1;17(12):e0271517. https://doi.org/10.1371/journal.pone.0271517
53 Poncin W, Schalkwijk A, Vander Straeten C, et al. Impact of surgical mask on performance and cardiorespiratory responses to submaximal exercise in COVID-19 patients near hospital discharge: A randomized crossover trial. Clin Rehabil. 2022 Aug;36(8):1032–1041. https://doi.org/10.1177/02692155221097214
54 Paiva DN, Wagner LE, Dos Santos Marinho SE, et al. Effectiveness of an adapted diving mask (Owner mask) for non-invasive ventilation in the COVID-19 pandemic scenario: study protocol for a randomized clinical trial. Trials. 2022 Mar 18;23(1):218. https://doi.org/10.1186/s13063-022-06133-y
55 Benítez-Robaina S, Ramos-Macias Á, Borkoski-Barreiro S, et al. COVID-19 era: Hearing handicaps behind face mask use in hearing aid users. J Int Adv Otol. 2022 Nov;18(6):465–470. https://doi.org/10.5152/iao.2022.21578
56 Feng L, Zhang Q, Ruth N, et al. Compromised skin barrier induced by prolonged face mask usage during the COVID-19 pandemic and its remedy with proper moisturization. Skin Res Technol. 2022 Nov 25. https://doi.org/10.1111/srt.13214
57 Lin Q, Cai Y, Yu C, et al. Effects of Wearing Face Masks on Exercise Capacity and Ventilatory Anaerobic Threshold in Healthy Subjects During the COVID-19 Epidemic. Med Sci Monit. 2022 May 30;28:e936069. https://doi.org/10.12659/MSM.936069
58 Mohd Kamil MK, Yuen Yoong KP, Noor Azhar AM, et al. Non-rebreather mask and low-flow nasal cannula vs high-flow nasal cannula in severe COVID-19 pneumonia in the emergency department. Am J Emerg Med. 2023 Jan;63:86–93. https://doi.org/10.1016/j.ajem.2022.10.029
59 Nazir N, Saxena A. The effectiveness of high-flow nasal cannula and standard non-rebreathing mask for oxygen therapy in moderate category COVID-19 pneumonia: Randomised controlled trial. Afr J Thorac Crit Care Med. 2022 May 5;28(1):10.7196/AJTCCM.2022.v28i1.206. https://doi.org/10.7196/AJTCCM.2022.v28i1.206
60 Chou R. Comparative Effectiveness of Mask Type in Preventing SARS-CoV-2 in Health Care Workers: Uncertainty Persists. Ann Intern Med. 2022 Dec;175(12):1763–1764. https://doi.org/10.7326/M22-3219
61 Heidinger A, Falb T, Werkl P, et al. The Impact of Tape Sealing Face Masks on Visual Field Scores in the Era of COVID-19: A Randomized Cross-over Study. J Glaucoma. 2021 Oct 1;30(10):878–881. https://doi.org/10.1097/IJG.0000000000001922
62 Wang MX, Gwee SXW, Chua PEY, et al. Effectiveness of Surgical Face Masks in Reducing Acute Respiratory Infections in Non-Healthcare Settings: A Systematic Review and Meta-Analysis. Front Med (Lausanne). 2020 Sep 25;7:564280. https://doi.org/10.3389/fmed.2020.564280
63 Dost B, Kömürcü Ö, Bilgin S, Dökmeci H, et al. Investigating the Effects of Protective Face Masks on the Respiratory Parameters of Children in the Postanesthesia Care Unit During the COVID-19 Pandemic. J Perianesth Nurs. 2022 Feb;37(1):94–99. https://doi.org/10.1016/j.jopan.2021.02.004
64 Bánfai B, Musch J, Betlehem J, et al. How effective are chest compressions when wearing mask? A randomised simulation study among first-year health care students during the COVID-19 pandemic. BMC Emerg Med. 2022 May 8;22(1):82. https://doi.org/10.1186/s12873-022-00636-2
65 Boyle KG, Napoleone G, Ramsook AH, et al. Effects of the Elevation Training Mask® 2.0 on dyspnea and respiratory muscle mechanics, electromyography, and fatigue during exhaustive cycling in healthy humans. J Sci Med Sport. 2022 Feb;25(2):167–172. https://doi.org/10.1016/j.jsams.2021.08.022
66 Femi-Abodunde A, Olinger K, Burke LMB, et al. Radiology Dictation Errors with COVID-19 Protective Equipment: Does Wearing a Surgical Mask Increase the Dictation Error Rate? J Digit Imaging. 2021 Oct;34(5):1294–1301. https://doi.org/10.1007/s10278-021-00502-w
67 Schultheis WG, Sharpe JE, Zhang Q, et al. Effect of Taping Face Masks on Quantitative Particle Counts Near the Eye: Implications for Intravitreal Injections in the COVID-19 Era. Am J Ophthalmol. 2021 May;225:166–171. https://doi.org/10.1016/j.ajo.2021.01.021
68 Science M, Caldeira-Kulbakas M, Parekh RS, et al., Back-to-School COVID-19 School Study Group. Effect of Wearing a Face Mask on Hand-to-Face Contact by Children in a Simulated School Environment: The Back-to-School COVID-19 Simulation Randomized Clinical Trial. JAMA Pediatr. 2022 Dec 1;176(12):1169–1175. https://doi.org/10.1001/jamapediatrics.2022.3833
69 Abbasi S, Siddiqui KM, Qamar-ul-Hoda M. Adverse Respiratory Events After Removal of Laryngeal Mask Airway in Deep Anesthesia Versus Awake State in Children: A Randomized Trial. Cureus. 2022 Apr 19;14(4):e24296. https://doi.org/10.7759/cureus.24296
70 Saxena A, Nazir N, Pandey R, et al. Comparison of Effect of Non-invasive Ventilation Delivered by Helmet vs Face Mask in Patients with COVID-19 Infection: A Randomized Control Study. Indian J Crit Care Med. 2022 Mar;26(3):282–287. https://doi.org/10.5005/jp-journals-10071-24155
71 Al Ali RA, Gautam B, Miller MR, et al. Laryngeal Mask Airway for Surfactant Administration Versus Standard Treatment Methods in Preterm Neonates with Respiratory Distress Syndrome: A Systematic Review and Meta-analysis. Am J Perinatol. 2022 Oct;39(13):1433–1440. https://doi.org/10.1055/s-0041-1722953
72 Iezadi S, Azami-Aghdash S, Ghiasi A, et al. Effectiveness of the non-pharmaceutical public health interventions against COVID-19; a protocol of a systematic review and realist review. PLOS One. 2020 Sep 29;15(9):e0239554. https://doi.org/10.1371/journal.pone.0239554 [Update in: PLOS One. 2021 Nov 23;16(11):e0260371]
73 Pin-On P, Leurcharusmee P, Tanasungnuchit S, et al. Desflurane is not inferior to sevoflurane in the occurrence of adverse respiratory events during laryngeal mask airway anesthesia: a non-inferiority randomized double-blinded controlled study. Minerva Anestesiol. 2020 Jun;86(6):608–616. https://doi.org/10.23736/S0375-9393.20.14202-0
7.4 Descriptive information about eligible study characteristics
Cochrane review literature
Jefferson et al. (2020)293 – Jefferson et al. ran computer searches in 6 databases:
- Cochrane Central Register of Controlled Trials (CENTRAL) (2020, Issue 3)
- PubMed (2010 to April 1, 2020)
- The biomedical research database Embase (2010 to April 1, 2020)
- CINAHL (Cumulative Index to Nursing and Allied Health Literature) (2010 to April 1, 2020)
- US National Institutes of Health Ongoing Trials Register ClinicalTrials.gov (January 2010 to March 16, 2020)
- World Health Organization International Clinical Trials Registry Platform (January 2010 to March 16, 2020)
Jefferson et al. identified and further analyzed 15 community (i.e., non-healthcare worker) RCTs—base studies—comparing medical masks to no masks using the generalized inverse-variance random-effects model. The viral illness outcomes they reported were: numbers of acute respiratory infections, influenza-like illness (ILI), laboratory-confirmed influenza (LCI), or other viral pathogens. The specific focus of this evaluation was on data for numbers of ILI and LCI. This included nine ILI and six LCI outcomes (Analysis 1.1, p. 143). All these data met the eligibility criteria.
Table 7.4.1 shows results for the 15 RCT base studies, including primary outcome measures (risk ratio and 95% confidence intervals) and p-values that were estimated. Their research claim, i.e., cause−effect scientific claim, was (Authors’ conclusions, p. 3): “pooled results of randomised trials did not show a clear reduction in respiratory viral infection with the use of medical/surgical masks during seasonal influenza.”
Table 7.4.1. Outcome measures (risk ratio and 95% confidence intervals) and p-values for 15 randomized controlled trials (base studies) included in Jefferson et al. meta-analysis
Outcome measure | 1st Author Year | Risk ratio (95% CI) | p-value |
Influenza-like illness (ILI) | Aiello 2012 | 1.10 (0.88 − 1.38) | 0.43304 |
" | Barasheed 2014 | 0.58 (0.32 − 1.04) | 0.02222 |
" | Canini 2010 | 1.03 (0.52 − 2.00) | 0.93667 |
" | Cowling 2008 | 0.88 (0.34 − 2.27) | 0.80744 |
" | Jacobs 2009 | 0.88 (0.02 − 31.84) | 0.98821 |
" | MacIntyre 2009 | 1.11 (0.64 − 1.91) | 0.73421 |
" | MacIntyre 2015 | 0.26 (0.03 − 2.51) | 0.24213 |
" | MacIntyre 2016 | 0.32 (0.03 − 3.11) | 0.38679 |
" | Suess 2012 | 0.61 (0.20 − 1.87) | 0.35996 |
Lab-confirmed influenza (LCI) | Aiello 2012 | 0.92 (0.59 − 1.42) | 0.70556 |
" | Cowling 2008 | 1.16 (0.31 − 4.34) | 0.87632 |
" | MacIntyre 2009 | 2.51 (0.74 − 8.50) | 0.44559 |
" | MacIntyre 2015 | 0.83 (0.45 − 1.56) | 0.54827 |
" | MacIntyre 2016 (1) | 0.97 (0.06 − 15.51) | 0.99393 |
" | Suess 2012 | 0.39 (0.13 − 1.19) | 0.02408 |
Medical research literature
Aggarwal et al. (2020)294 – Aggarwal et al. ran computer searches on April 25, 2020, in two databases: PubMed and Embase. They identified 902 records from their searches. They undertook reviews of 83 full-text articles, of which 74 were excluded by their criteria. The remaining nine studies (cluster-RCTs) were used by Aggarwal et al. for their meta-analysis. Five of these studies compared medical-mask and no-mask use by community participants. Their meta-analysis used the random effects model. Viral illness outcomes they reported were ILI, self-reported ILI, and LCI.
Aggarwal et al.’s results for the 5 cluster-RCT base studies are shown in Table 7.4.2. These include outcome measures (effect sizes and 95% confidence intervals) and p-values that were estimated. Two of the five outcome measures failed to meet the eligibility criteria, as they were based on self-reported ILI (with attendant awareness bias) (Table 7.4.2). Consequently, we did not use Aggarwal et al.’s results for p-value plotting. The research claim, taken from their abstract, was: “data pooled from randomized controlled trials do not reveal a reduction in occurrence of ILI with use of facemask alone in community settings.”
Xiao et al. (2020)295 – Xiao et al. investigated multiple nonpharmaceutical measures (hand hygiene, masks) for pandemic influenza in nonhealthcare (community) settings. For the ‘mask’ component of their investigation, they ran computer searches in four databases (CENTRAL, PubMed, Embase, and Medline) to identify ‘randomized controlled trial in community setting’ studies that were available from 1946 through July 28, 2018. They identified and screened the titles of 1,100 articles, from which 856 were excluded.
Table 7.4.2. Outcome measures (risk ratio and 95% confidence intervals) and p-values for 5 randomized controlled trials (base studies) included in Aggarwal et al. meta-analysis
Outcome measure | 1st Author Year | Effect size (95% CI) | p-value |
Self-reported ILI | Aiello 2010a | −0.33 (−0.64 − −0.02) | 0.0369 |
Lab-confirmed influenza (LCI) | Aiello 2012 | −0.16 (−0.63 − 0.31) | 0.5046 |
" | Cowling 2008 | 0.69 (−0.56 − 1.95) | 0.2812 |
Lab-confirmed viral infection (influenza) | MacIntyre 2009 | 0.25 (−0.43 − 0.94) | 0.4744 |
Self-reported ILI | Suess 2012 | −0.49 (−1.85 − 0.86) | 0.4785 |
Xiao et al. reviewed full abstracts of the remaining 244 articles and undertook reviews of 98 full-text articles. From this list, they identified 10 RCT articles, of which they included seven RCTs as base studies in a meta-analysis of medical mask versus no mask using the fixed effects model. The viral illness outcome they reported was LCI.
Xiao et al.’s results for the seven RCT base studies are shown in Table 7.4.3. These include outcome measure (risk ratio and 95% confidence intervals) and p-values that were estimated. The research claim, taken from their abstract, was: “Although mechanistic studies support the potential effect of hand hygiene or face masks, evidence from 14 randomized controlled trials of these measures did not support a substantial effect on transmission of laboratory-confirmed influenza.”
Table 7.4.3. Outcome measures (risk ratio and 95% confidence intervals) and p-values for 7 randomized controlled trials (base studies) included in Xiao et al. meta-analysis
Outcome measure | 1st Author Year | Risk ratio (95% CI) | p-value |
Lab-confirmed influenza (LCI) | Aiello 2010a | 2.34 (0.56 − 9.72) | 0.5663 |
" | Aiello 2012 | 0.71 (0.34 − 1.48) | 0.3187 |
" | Baeasheed 2014 | 7.43 (0.33 − 169.47) | 0.8815 |
" | Cowling 2008 | 1.12 (0.37 − 3.35) | 0.8746 |
" | Macintyre 2009 | 3.19 (0.13 − 77.36) | 0.9115 |
" | Macintyre 2016 | 0.33 (0.01 − 7.96) | 0.7411 |
" | Suess 2012 | 0.38 (0.38 − 0.89) | 0.0009 |
Nanda et al. (2021)296 – Nanda et al. evaluated RCTs of cloth and medical face-mask use (± hand hygiene) for preventing respiratory virus transmission in the community setting. They ran computer searches in three databases (CENTRAL, PubMed, Embase). They identified and screened the titles of 1,499 articles, from which 1,126 were excluded. They reviewed full texts of 373 articles. From this list, they included 11 RCT articles as base studies in their meta-analysis. The viral illness outcome they reported was laboratory-confirmed virus.
Nanda et al.’s results for the seven RCT base studies are shown in Table 7.4.4. These include outcome measure (risk ratio and 95% confidence intervals) and p-values that were estimated. The research claim, taken from their abstract, was: “There is limited available preclinical and clinical evidence for face mask benefit in sars-cov-2. RCT evidence for other respiratory viral illnesses shows no significant benefit of masks in limiting transmission.”
Table 7.4.4. Outcome measures (risk ratio and 95% confidence intervals) and p-values for 7 randomized controlled trials (base studies) included in Nanda et al. meta-analysis
Outcome measure | 1st Author Year | Risk ratio (95% CI) | p-value |
Lab-confirmed virus (influenza) | Aiello 2010a | 0.99 (0.98 − 1.01) | 0.192629 |
" | Aiello 2012 | 1.01 (0.99 − 1.04) | 0.436782 |
" | Baeasheed 2014 | 0.92 (0.81 − 1.05) | 0.209509 |
" | Cowling 2008 | 0.99 (0.92 −1.07) | 0.806279 |
" | Macintyre 2009 | 0.97 (0.91 − 1.03) | 0.340394 |
" | Macintyre 2016 | 1.01 (1.00 − 1.02) | 0.048497 |
" | Suess 2012 | 1.19 (1.03 − 1.37) | 0.016719 |
Tran et al. (2021)297 – Tran et al. registered a protocol for their study in PROSPERO on May 7, 2020. They performed a systematic review and network meta-analysis of RCTs to assess the efficacy of face masks in preventing respiratory infections in community settings. They ran computer searches in nine databases: CENTRAL, PubMed, Embase, Web of Science (ISI), Scopus, Google Scholar, ASSIA, Clinicaltrials.gov, and System for Information on Grey Literature in Europe (SIGLE).
They identified and screened the titles and abstracts of 13,988 articles, from which 13,876 were excluded. They reviewed full texts of 112 articles, and they added 1 article from gray literature. From this list, they selected 16 RCT articles for their overall analysis and included eight RCT articles as base studies in their mask versus no mask meta-analysis.
Tran et al. used the fixed effects model in their meta-analysis. The viral illness outcome they reported was ILI. Seven of the eight RCT base studies used in their meta-analysis were the exact same as those used by Xiao et al. (2020) and Nanda et al. (2021). Tran et al.’s results for the eight RCT base studies are shown in Table 7.4.5. This includes outcome measure (risk ratio and 95% confidence intervals) and p-values that were estimated.
Table 7.4.5. Outcome measures (risk ratio and 95% confidence intervals) and p-values for 8 randomized controlled trials (base studies) included in Tran et al. meta-analysis
Outcome measure | 1st Author Year | Risk ratio (95% CI) | p-value |
Influenza-like illness (ILI) | Aiello 2010a | 0.78 (0.64 − 0.96) | 0.007 |
" | Aiello 2012 | 0.85 (0.58 − 1.24) | 0.373 |
" | Barasheed 2014 | 0.58 (0.33 − 1.01) | 0.0155 |
" | Canini 2010 | 1.02 (0.61 − 1.71) | 0.9432 |
" | Cowling 2008 | 2.05 (0.69 − 6.04) | 0.4417 |
" | MacIntyre 2009 | 1.31 (0.72 − 2.40) | 0.4695 |
" | MacIntyre 2016 | 0.33 (0.03 − 3.11) | 0.0116 |
" | Suess 2012 | 0.51 (0.21 − 1.25) | 0.0648 |
The research claim, taken from their abstract, was: “Given the body of evidence through a systematic review and meta-analyses, our findings supported the protective benefits of MFMs [medical face masks] in reducing respiratory transmissions, and the universal mask-wearing should be applied—especially during the COVID-19 pandemic.”
Kim et al. (2022)298 – Kim et al. initially registered a protocol for their study in PROSPERO on October 28, 2020, and changed the protocol on November 20, 2020. They performed a network meta-analysis of RCTs to assess the efficacy of face masks in preventing respiratory infections in community settings. They ran computer searches in PubMed, Google Scholar and medRxiv databases for studies published up to February 5, 2021.
Kim et al. identified and screened the titles of 5,946 articles, from which 5,761 were excluded. They reviewed full texts of 185 articles. From this list, they selected 35 articles for their overall analysis, which included RCTs, prospective cohort studies, retrospective cohort studies, case–control studies, and cross‐sectional studies.
Kim et al. focused on RCTs for their mask versus no mask meta-analysis. Kim et al. used the random effects model in their meta-analysis. The viral illness outcomes they reported were LCI for influenza (6 base studies) and LCI for COVID-19 (1 base study).
Table 7.4.6 shows Kim et al.’s results for the seven RCT base studies. These include outcome measure (risk ratio and 95% confidence intervals) and p-values that were estimated. The research claim, taken from their abstract, was: “Evidence supporting the use of medical or surgical masks against influenza or coronavirus infections (SARS, MERS and COVID‐19) was weak.”
Table 7.4.6. Outcome measures (odds ratio and 95% confidence intervals) and p-values for 7 randomized controlled trials (base studies) included in Kim et al. meta-analysis
Outcome measure | 1st Author Year | Odds ratio (95% CI) | p-value |
LCI | Aiello 2012 | 0.7 (0.33 − 1.5) | 0.3148 |
" | Alfelali 2020 | 1.16 (0.55 − 2.48) | 0.7452 |
Lab-confirmed COVID-19 | Bundgaard 2020 | 0.82 (0.55 − 1.23) | 0.2294 |
LCI | Cowling 2008 | 1.16 (0.31 − 4.34) | 0.8763 |
" | MacIntyre 2011 | 0.52 (0.13 − 2.09) | 0.3371 |
" | MacIntyre 2009 | 4.96 (0.26 − 92.99) | 0.8671 |
" | Suess 2012 | 0.32 (0.12 − 0.84) | 0.0002 |
Ollila et al. (2022)299 – Ollila et al. initially registered a protocol for their study in PROSPERO on November 16, 2020, and changed the protocol on May 12, 2022, and September 22, 2022; it was published on December 1, 2022. They performed a systematic review and meta-analysis of RCTs to assess the efficacy of face masks in preventing respiratory infections in community settings.
They ran computer searches in CENTRAL, PubMed, Embase, and the Web of Science databases for studies published between 1981 and February 9, 2022. We note that well into their study (initially registered November 16, 2020), they first changed the research protocol 16 months later (May 12, 2022), and then again four months later.
They identified and screened 1,836 articles, from which 1,785 were excluded. They reviewed full texts of 49 articles. From this list, they selected 18 RCT articles for their analysis; eight of these were specific to community settings, and 10 were specific to non-community settings. Here, we were interested in the eight results for community settings.
Ollila et al.’s results for the eight RCT base studies are shown in Table 7.4.7. This just includes outcome measures (odds ratio and 95% confidence intervals), not p-values. The research claim, taken from their abstract, was: “Our findings support the use of face masks particularly in a community setting and for adults.”
We did not estimate p-values for the base study statistics used by Ollila et al. Six of the eight outcome measures failed to meet the eligibility criteria. Specifically, five of these measures were based on self-reported symptoms (with attendant awareness bias), and the origin of one measure Ollila et al. used for another base study could not be confirmed (Table 7.4.7). We determined this by accessing and reading each of the eight base studies used by Ollila et al.
From reading the base studies and recognizing that Ollila et al. changed their protocol twice—well into the study before it was published—we are concerned about the reliability of this meta-analysis. Nowhere in the meta-analysis do they state which outcome measures they used.
These practices—changing the research protocol multiple times and failing to indicate specific outcome measures in their paper—imply selective analysis and reporting. Researchers have flexibility to use different methods in a study. Unfortunately, they then have further flexibility to only report those methods that yield favorable results and ignore those that yield unfavorable results.300
This preferential reporting involves the selective tendency to highlight statistically significant findings and to avoid highlighting nonsignificant findings in research.301 This can be problematic because the significant findings could, in the future, turn out to be false positives.
Table 7.4.7. Outcome measures (odds ratio and 95% confidence intervals) and p-values for 8 randomized controlled trials (base studies) included in Ollila et al. meta-analysis
Outcome measure | 1st Author Year | Odds ratio (95% CI) | p-value |
Self-reported viral symptoms* | Barasheed 2014 | 0.393 (0.161 − 0.959) | |
Self-reported viral symptoms* | Aiello 2010a | 0.709 (0.552 − 0.910) | |
Unknown⁰ | Aiello 2012 | 0.725 (0.497 − 1.058) | |
Lab-reported COVID-19 infection | Bundgaard 2020 | 0.815 (0.542 − 1.226) | |
Self-reported ILI symptoms | Aelami 2015 | 0.874 (0.644 − 1.187) | |
Self-reported COVID-19 symptoms*+ | Abaluck 2021 | 0.908 (0.829 − 0.995) | |
Self-reported ARI symptoms | Abdin 2005 | 0.970 (0.733 − 1.284) | |
Clinical confirmed respiratory infection | Alfelali 2020 | 1.089 (0.828 − 1.277) |
* Laboratory-confirmed measures did not show a difference between mask and control groups.
⁰ Unable to establish what statistics were used from review of base study article.
+ The Chikina et al. (2022) re-analysis states that all of the outcomes in the study are based on self-reported symptoms.
Note: Ollila et al. do not state anywhere in their study which outcome measures they used.
Also, the test statistics Ollila et al. used for three of the base studies for self-reported symptoms showing a benefit of mask use in Table 7.4.7—Barasheed et al. (2014), Aiello et al. (2010a), and Abaluck et al. (2021)—are opposite to other published data of lab-confirmed statistics for the same studies.302
Specifically, Ollila et al. reported a significant difference between mask and control group outcomes in their meta-analysis for these three base studies, whereas published data exist for laboratory-confirmed infections which show no difference between the mask and control groups.
For the Barasheed et al. (2014) base study, data reported for lab-confirmed infections in their published paper showed no difference.303 For Aiello et al. (2010a), another Aiello publication at the same time304 reported that polymerase chain reaction (PCR)-confirmed infections for the same study showed no difference between mask and control groups.
For the Abaluck et al. (2021) base study, Chikina et al. (2022)305 independently reviewed this study and identified numerous biases unreported by Abaluck et al. that obscure inferences of causality. The Chikina et al. review identified a difference of just 20 lab-confirmed COVID-19 cases between the mask and no mask groups in a study population of over 300,000 individuals (i.e., 1,106 COVID-19 symptomatic seropositives in the mask group versus 1,086 in the no mask group).
The independent reviewers stated that: “it would not be reasonable to conclude from this trial that there is a direct causal link between mask wearing and the number of residents in villages and households, any causal claims based on effects of similar size in this trial should be considered with caution.”306
A final observation is the “main” result reported by Abaluck et al.’s (2021) study (Results, p. 1): “Adjusting for baseline covariates, the intervention [masking] reduced symptomatic seroprevalence by 9.5% (adjusted prevalence ratio = 0.91 [0.82, 1.00].” This result is not significant (p-value=0.062).307
As the self-reported statistics used by Ollila et al. for the Abaluck et al. (2021) base study were opposite to more-reliable, lab-confirmed statistics, and given other biases identified by Chikina et al., the Ollila et al. study itself and their claim, “support the use of face masks particularly in a community setting and for adults,” is judged unreliable.
Gray literature
Liu et al. (2021)308 – The Liu et al. systematic review involved examining available clinical evidence of the effect of face-mask use in community settings on respiratory infection rates, including by COVID-19. This review differed from the other meta-analyses we evaluated in that it did not specify its methodologies for identifying RCT base studies. However, the authors did present and discuss the results of RCTs that they identified.
As a result of their different methodology, we attempted to obtain original copies of the base studies to confirm the results reported by Liu et al. They reported outcome measures as p-values for 16 RCT base papers. We only obtained 14 of the 16 base papers. Lui et al.’s results for the 14 base papers are presented in Table 7.4.8.
Regarding their results, we were specifically interested in data for the clinical diagnosis of ILI, as well as LCI or other laboratory-confirmed viral pathogens. We identified multiple test statistics in the 14 base papers. We converted these statistics to p-values and presented them in Table 7.4.8 (p-values used for plotting are highlighted, bolded, and italicized).
The research claim, taken from their abstract, was: “Of sixteen quantitative meta-analyses, eight were equivocal or critical as to whether evidence supports a public recommendation of masks, and the remaining eight supported a public mask intervention on limited evidence primarily on the basis of the precautionary principle.”
Table 7.4.8. Outcome measures (p-values) for 16 randomized controlled trials (base studies) included in Liu et al. systematic review
Base study |
Intervention |
Control group |
Outcomes |
P-value |
Comments |
Aiello et al. (2010a) [U. Mich. Dorms] |
medical mask (MM) |
no MM |
influenza-like illness (ILI); lab (PCR)-confirmed influenza infection |
0.25 |
for ILI; [note: PCR results stated as non-significant, no data provided to estimate p-value, ILI data not used for p-value plot] |
Aiello et al. (2012) [U. Mich. dorms] |
MM |
no MM |
ILI; lab (RT-PCR)-confirmed influenza infection |
0.52 0.42 0.72 0.69 |
for ILI before adjustments for covariates; for ILI after adjustments for covariates; for RT-PCR before adjustments for covariates; for RT-PCR after adjustments for covariates |
Abdin et al. (2005) [Hajj pilgrims] |
MM |
no MM |
acute respiratory infection |
0.84 |
for acute respiratory infection; (OR 0.97, 95% CI 0.73−1.28); p-value estimated; [note: not used for p-value plot, as base study unavailable to review] |
Barasheed et al. (2014) [Hajj pilgrims] |
MM |
no MM |
ILI; lab (swab testing)-confirmed virus infection |
0.04 0.90 0.90 |
for ILI; for lab-confirmed Influenza A virus infection; for lab-confirmed Influenza B virus infection |
Alfelali et al. (2020) [Hajj Pilgrims] |
MM |
no MM |
respiratory virus infections (RVIs); lab-confirmed RVIs |
0.18 0.40 0.26 0.06 |
for RVIs (intention-to-treat analysis); for lab-confirmed RVIs (intention-to-treat analysis); for RVIs (per-protocol analysis); for lab-confirmed RVIs (per-protocol analysis) [note: not used for p-value plot, as viruses included rhinovirus, influenza viruses, parainfluenza viruses] |
Canini et al. (2010) [households in France] |
MM |
no MM |
ILI – positive rapid Influenza A test |
1.00 |
for difference in ILI between groups |
Macintyre et al. (2009) [households in Australia] |
MM |
no MM |
ILI; lab-confirmed total virus infections (VIs) |
0.50 0.46 0.32 |
for ILI (by house); for ILI (by individual); for lab-confirmed total VIs; [note: not used for p-value plot, as viruses included Influenza A and B, respiratory syncytial virus, adenovirus, parainfluenza viruses (PIV) types 1–3, coronaviruses, human metapneumovirus, enteroviruses, rhinoviruses] |
Macintyre et al. (2016) [households in China] |
MM |
no MM |
ILI; clinical respiratory illness (CRI), lab-confirmed viral illnesses |
0.34 0.44 0.98 |
for ILI; for clinical respiratory illness (CRI); for lab-confirmed viral illnesses; [p-values estimated] |
Simmerman et al. (2011) [households in Thailand] |
MM + hand washing |
No intervention (MM or hand washing) |
lab-confirmed influenza (by RT-PCR or serology) |
0.525 |
for lab-confirmed influenza |
Note: p-values italicized & bolded used for p-value plot.
Table 7.4.8. Outcome measures (p-values) for 16 randomized controlled trials (base studies) included in Liu et al. systematic review (con’t)
Base study |
Intervention |
Control |
Outcomes |
P-value |
Comments |
Cowling et al. (2008) [households in Hong Kong] |
MM |
no MM |
lab-confirmed influenza; clinical influenza definition 1; clinical influenza definition 2; clinical influenza definition 3 |
0.99 1.00 0.97 0.52 |
for lab-confirmed influenza; for clinical influenza definition 1; for clinical influenza definition 2; for clinical influenza definition 3 |
Cowling et al. (2009) [households in Hong Kong] |
MM + hand hygiene |
No intervention (MM or hand hygiene) |
Influenza A + B virus infection confirmed by RT-PCR; clinical diagnosis after 7 days (2 definitions) |
0.48 0.37 0.26 |
for lab (RT-PCR)-confirmed influenza (OR 0.77, 95% CI 0.38–1.55); for clinical influenza definition 1 (OR 1.25, 95% CI 0.79–1.98); for clinical influenza definition 2 (OR 1.68, 95% CI 0.68–4.15); [p-values estimated] |
Suess et al. (2012) [households in Germany] |
MM |
no MM |
lab-confirmed (RT-PCR) for influenza; clinical ILI |
0.10 0.30 |
for lab-confirmed (RT-PCR); for clinical ILI |
Larson et al. (2010) [Hispanic households in New York City] |
MM + hand sanitizer + education |
Education only (i.e., no MM + hand sanitizer) |
Influenza (A or B) confirmatory testing by culture or RT-PCR; ILI (CDC definition); viral upper respiratory infections |
0.893 0.61 0.194 |
for influenza (RT-PCR lab-confirmed); for ILI; for viral upper respiratory infections |
Jacobs et al. (2009) [hospital workers in Japan] |
MM |
no MM |
presence of a cold based on a previously validated measure of self-reported symptoms |
0.81 |
for presence of a cold; 32 health care workers completed the study; 8 symptoms recorded daily [note: not used for p-value plot, as base study unavailable to review] |
Bundgaard et al. (2021) [adult community members in Denmark] |
MM |
no MM |
SARS-CoV-2 infection at 1 month by: IgM antibody testing; IgG antibody testing; RT-PCR, or healthcare-diagnosed |
0.35 0.58 0.80 – 0.23 |
for SARS-CoV-2 infection: main trial measurement end point (OR 0.82, 95% CI 0.54–1.23); +ve IgM antibody test result (OR 0.87, 95% CI 0.54–1.41); +ve IgG antibody test result (OR 1.07, 95% CI 0.66–1.75); RT-PCR positivity (n/a); healthcare-diagnosed (OR 0.52, 95% CI 0.18–1.53); [p-values estimated] |
Abaluck et al. (2021) [cluster randomized communities in Bangladesh] |
MM |
no MM |
reduction in symptomatic SARS-CoV-2 seroprevalence |
0.066 0.062 |
reduced symptomatic SARS-CoV-2 seroprevalence, 2 results are given: n=200 blood samples, symptomatic seroprevalence adjusted prevalence ratio = 0.89 [0.78, 1.00]; n=10,790 blood samples, symptomatic seroprevalence adjusted prevalence ratio = 0.91 [0.82, 1.00]; [p-values estimated; note: results for non-medical, cloth masks excluded] |
Note: p-values italicized & bolded used for p-value plot.
References
Abaluck, J., Kwong, L. H., Styczynski, A., et al. 2022. Impact of community masking on COVID-19: A cluster-randomized trial in Bangladesh. Science 375, 6577: eabi9069. https://www.science.org/doi/10.1126/science.abi9069.
Adam, D. 2020. Special report: The simulations driving the world’s response to COVID-19. Nature, April 2, 2020. https://www.nature.com/articles/d41586-020-01003-6.
Adiga, A., Dubhashi, D., Lewis, B. et al. 2020. Mathematical Models for COVID-19 Pandemic: A Comparative Analysis. Journal of the Indian Institute of Science 100, 4: 793–807. https://doi.org/10.1007/s41745-020-00200-6.
Aggarwal, N., Dwarakanathan, V., Gautam, N., and Ray, A. 2020. Facemasks for prevention of viral respiratory infections in community settings: A systematic review and meta-analysis. Indian Journal of Public Health 64, Supplement: S192–S200. https://pubmed.ncbi.nlm.nih.gov/32496254/.
Aiello, A. E., Murray, G. F., Perez, V., et al. 2010a. Mask use, hand hygiene, and seasonal influenza-like illness among young adults: A randomized intervention trial. Journal of Infectious Diseases 201, 4: 491–498. https://doi.org/10.1086/650396.
Aiello, A. E., Coulborn, R. M., Perez, V. et al. 2010b. A randomized intervention trial of mask use and hand hygiene to reduce seasonal influenza-like illness and influenza infections among young adults in a university setting. International Journal of Infectious Diseases 14 (Supplement 1): e320. https://doi.org/10.1016/j.ijid.2010.02.2201
AIER (American Institute for Economic Research). 2020. Cost of Lockdowns: A Preliminary Report. AIER, Great Barrington, MA. https://www.aier.org/article/cost-of-us-lockdowns-a-preliminary-report/.
Alderson, P. 2004. Absence of evidence is not evidence of absence. British Medical Journal 328, 7438: 476. https://doi.org/10.1136/bmj.328.7438.476.
Allen, D. W. 2022. Covid-19 Lockdown Cost/Benefits: A Critical Assessment of the Literature. International Journal of the Economics of Business 29, 1: 1–32. https://doi.org/10.1080/13571516.2021.1976051.
Allison, D. B., Brown, A. W., George, B. J., Kaiser, K. A. 2016. Reproducibility: A tragedy of errors. Nature 530, 7588: 27–29. https://doi.org/10.1038/530027a.
Al-Marzouki, S., Evans, S., Marshall, T., and Roberts, I. 2005. Are these data real? Statistical methods for the detection of data fabrication in clinical trials. British Medical Journal 331: 267. https://doi.org/10.1136/bmj.331.7511.267.
Altman, D. G., and Bland, J. M. 1995. Statistics notes: Absence of evidence is not evidence of absence. British Medical Journal 311, 7003: 485. https://doi.org/10.1136/bmj.311.7003.485.
Altman, D. G., and Bland, J. M. 2011a. How to obtain the confidence interval from a P value. British Medical Journal 343, d2090. https://doi.org/10.1136/bmj.d2090.
Altman, D. G., and Bland, J. M. 2011b. How to obtain the P value from a confidence interval. British Medical Journal 343, d2304. https://doi.org/10.1136/bmj.d2304.
Anderson, M. S., Ronning, E. A., De Vries, R., Martinson, B. C. 2010. Extending the Mertonian Norms: Scientists’ Subscription to Norms of Research. The Journal of Higher Education 81, 3: 366–393. https://dx.doi.org/10.1353%2Fjhe.0.0095.
Anderson, J. H. 2021. Do Masks Work? A review of the evidence. City Journal, August 11, 2021. https://www.city-journal.org/do-masks-work-a-review-of-the-evidence.
Archer, E. 2020. The Intellectual and Moral Decline in Academic Research. The James G. Martin Center for Academic Renewal, January 29, 2020. https://www.jamesgmartin.center/2020/01/the-intellectual-and-moral-decline-in-academic-research/.
Axe, D., Briggs, W. M., Richards, J. W. 2020. The Price of Panic: How the Tyranny of Experts Turned a Pandemic into a Catastrophe. Washington, DC: Regnery Publishing.
Axfors, C., and Ioannidis, J. P. A. 2022. Infection fatality rate of COVID-19 in community-dwelling elderly populations. European Journal of Epidemiology 37, 3: 235–249. https://doi.org/10.1007/s10654-022-00853-w.
Baker, M. 2016. 1,500 scientists lift the lid on reproducibility. Nature 533, 7604: 452–454. http://www.nature.com/news/1-500-scientists-lift-the-lid-on-reproducibility-1.19970.
Bałazy, A., Toivola, M., Adhikari, A., et al. 2006. Do N95 respirators provide 95% protection level against airborne viruses, and how adequate are surgical masks? American Journal of Infection Control 34, 2: 51−57. https://doi.org/10.1016/j.ajic.2005.08.018.
Ballin, M., Ioannidis, J. P., Bergman, J., Kivipelto, M., Nordström, A., and Nordström, P. 2022. Time-varying risk of death after SARS-CoV-2 infection in Swedish long-term care facility residents: a matched cohort study. BMJ Open 12, 11: e066258. https://doi.org/10.1136/bmjopen-2022-066258.
Bandholm, T., Christensen, R., Thorborg, K., Treweek, S., Henriksen, M. 2017. Preparing for what the reporting checklists will not tell you: the PREPARE Trial guide for planning clinical research to avoid research waste. British Journal of Sports Medicine 51, 20: 1494–1501. https://doi.org/10.1136/bjsports-2017-097527.
Barasheed. O., Rashid, H., Alfelali, M., et al.; Hajj Research Team. 2014. Viral respiratory infections among Hajj pilgrims in 2013. Virologica Sinica 29, 6: 364−371. https://doi.org/10.1007/s12250-014-3507-x.
Bar-On, Y. M., Flamholz, A., Phillips, R., and Milo, R. 2020. SARS-CoV-2 (COVID-19) by the numbers. eLife 9: e57309. https://doi.org/10.7554/eLife.57309.
Begley, C. G., and Ellis, L. M. 2012. Drug development: Raise standards for preclinical cancer research. Nature 483: 531-33. https://doi.org/10.1038/483531a.
Belkin, N. L. 1996. A century after their introduction, are surgical masks necessary? American Operating Room Nurses Journal 64, 4: 602−607. http://doi.org/10.1016/s0001-2092(06)63628-4.
Bendavid, E., Oh, C., Bhattacharya, J., and Ioannidis, J. P. A. 2021. Assessing mandatory stay-at-home and business closure effects on the spread of COVID-19. European Journal of Clinical Investigation 51, 4: e13484. https://doi.org/10.1111/eci.13484.
Benjamin, D. J., Berger, J. O., Johannesson, M., et al. 2018. Redefine statistical significance. Nature Human Behaviour 2, 1: 6−10. https://doi.org/10.1038/s41562-017-0189-z.
Benjamini, Y., and Hochberg, Y. 1995. Controlling the False Discovery Rate: A Practical and Powerful Approach to Multiple Testing. Journal of the Royal Statistical Society, Series B 57, 1: 289−300. https://doi.org/10.1111/j.2517-6161.1995.tb02031.x.
Berger, J. O., and Sellke, T. 1987. Testing a Point Null Hypothesis: The Irreconcilability of P Values and Evidence. Journal of the American Statistical Association 33, 82, 397: 112–122. https://doi.org/10.1080/01621459.1987.10478397.
Bertozzi, A. L., Franco, E., Mohler, G., Short, M. B., and Sledge, D. 2020. The challenges of modeling and forecasting the spread of COVID-19. Proceedings of the National Academy of Sciences of the United States of America 117, 29: 16732–16738. https://doi.org/10.1073/pnas.2006520117.
Bidel, S., Hu, G., Jousilahti, P., Pukkala, E., Hakulinen, T., Tuomilehto, J. 2013. Coffee consumption and risk of gastric and pancreatic cancer—A prospective cohort study. International Journal of Cancer 132, 7: 1651–1659. https://doi.org/10.1002/ijc.27773.
Biggerstaff, M., Slayton, R. B., Johansson, M. A., and Butler, J. C. 2022. Improving Pandemic Response: Employing Mathematical Modeling to Confront Coronavirus Disease 2019. Clinical Infectious Diseases 74, 5: 913–917. https://academic.oup.com/cid/article/74/5/913/6338173.
Blanding, M. 2021. Symposium encourages ‘anti-racism’ focus for public health. Harvard T. H. Chan School of Public Health, September 29, 2021. https://www.hsph.harvard.edu/news/features/symposium-encourages-anti-racism-focus-for-public-health/.
BNC (Palestinian BDS National Committee). 2021. EqualHealth Campaign Against Racism Issue Statement of Solidarity and Endorse BDS. BDS, May 17, 2021. https://bdsmovement.net/EqualHealth-Campaign-Against-Racism-Issue-Statement-of-Solidarity-Endorse-BDS.
Boccia, S., Ricciardi, W., and Ioannidis, J. P. A. 2020. What Other Countries Can Learn From Italy During the COVID-19 Pandemic. JAMA Internal Medicine 180, 7: 927–928. https://doi.org/10.1001/jamainternmed.2020.1447.
Boos, D. D., and Stefanski, L. A. 2011. P-Value Precision and Reproducibility. The American Statistician 65, 4: 213−221. https://doi.org/10.1198/tas.2011.10129.
Bordewijk, E. M., Wang, R., Askie, L. M., Gurrin, L. C., Thornton, J. G., van Wely, M., Li, W., and Mol, B. W. 2020. Data integrity of 35 randomised controlled trials in women’ health. The European Journal of Obstetrics & Gynecology and Reproductive Biology 249: 72‒83. https://doi.org/10.1016/j.ejogrb.2020.04.016.
Borlée, F., Yzermans, C. J., Oostwegel, F. S. M., et al. 2019. Attitude toward livestock farming does not influence the earlier observed association between proximity to goat farms and self-reported pneumonia. Environmental Epidemiology 3, 2: e041. http://doi.org/10.1097/EE9.0000000000000041.
Boyd, C. 2020. Neil Ferguson whose grim warnings prompted Boris Johnson to order TOTAL LOCKDOWN admits Sweden may have suppressed Covid-19 to the same level but WITHOUT draconian measures. Daily Mail, June 2, 2020. https://www.dailymail.co.uk/news/article-8379769/Professor-Lockdown-Neil-Ferguson-admits-greatest-respect-Sweden.html.
Brauer, F. 2017. Mathematical epidemiology: Past, present, and future. Infectious Disease Modelling 2, 2: 113–127. https://www.ncbi.nlm.nih.gov/pmc/articles/PMC6001967/.
Briggs, W. M. 2016. Uncertainty: The Soul of Modeling, Probability, & Statistics. New York, NY: Springer.
Briggs, W. M. 2017. The Substitute for p-values. Journal of the American Statistical Association 112: 897–898. https://doi.org/10.1080/01621459.2017.1311264.
Briggs, W. M. 2018. Uncertainty: The Soul of Models, Probability & Statistics. Chapter Abstracts. William M. Briggs, March 14, 2018. https://www.wmbriggs.com/post/18724/.
Briggs, W. M. 2019. Everything Wrong with P-Values Under One Roof. In: Beyond Traditional Probabilistic Methods in Economics, ECONVN 2019, Studies in Computational Intelligence, Volume 809, eds. Kreinovich V., Thach N., Trung N., Van Thanh D. Cham, Switzerland: Springer. https://doi.org/10.1007/978-3-030-04200-4_2.
Briggs, W. M. 2020. Should We Have Trusted Expert Epidemiological Models? William M. Briggs, April 15, 2020. https://www.wmbriggs.com/post/30383.
Bruns, S. B., and Ioannidis, J. P. A. 2016. p-Curve and p-Hacking in Observational Research. PLOS One 11, 2: e0149144. https://doi.org/10.1371/journal.pone.0149144.
Buchanan, J. M., and Tullock, G. 2004. The Calculus of Consent: Logical Foundations of Constitutional Democracy. Indianapolis: Liberty Fund, Inc.
Bundgaard, H., Bundgaard, J. S., Raaschou-Pedersen, D. E. T., et al. 2021. Effectiveness of Adding a Mask Recommendation to Other Public Health Measures to Prevent SARS-CoV-2 Infection in Danish Mask Wearers: A Randomized Controlled Trial. Annals of Internal Medicine 174, 3: 335‒343. http://doi.org/10.7326/M20-6817.
California. 2020. Executive Order N-33-20. Executive Department, State of California. https://www.gov.ca.gov/wp-content/uploads/2020/03/3.19.20-attested-EO-N-33-20-COVID-19-HEALTH-ORDER.pdf.
Canini, L., Andréoletti, L., Ferrari, P., et al. 2010. Surgical Mask to Prevent Influenza Transmission in Households: A Cluster Randomized Trial. PLOS One 5, 11: e13998. http://doi.org/10.1371/journal.pone.0013998.
Carp, J. 2012. The secret lives of experiments: Methods reporting in the fMRI literature. NeuroImage 63, 1: 289−300. http://dx.doi.org/10.1016/j.neuroimage.2012.07.004.
Carter, E. C., Schönbrodt, F. D., Gervais, W. M., Hilgard, J. 2019. Correcting for Bias in Psychology: A Comparison of Meta-Analytic Methods. Advances in Methods and Practices in Psychological Science 2, 2: 115‒144. https://doi.org/10.1177/2515245919847196.
Case, R. B., Heller, S. S., Case, N. B., Moss, A. J. 1985. Type A Behavior and Survival after Acute Myocardial Infarction. New England Journal of Medicine 312, 12: 737–41. https://doi.org/10.1056/NEJM198503213121201.
CDC (Centers for Disease Control and Prevention). 2022. CDC Museum COVID-19 Timeline, August 16, 2022. https://www.cdc.gov/museum/timeline/covid19.html
Cecil, J. S., and Griffin, E. 1985. The Role of Legal Policies in Data Sharing. In Sharing Research Data, eds. Fienberg, S.E., Martin, M. E., Straf, M. L. Washington, DC: National Academy Press. 148–198. https://www.nap.edu/read/2033/chapter/15.
Chamberlain, S. 2021. Fauci emails show his flip-flopping on wearing masks to fight COVID. New York Post, June 3, 2021. https://nypost.com/2021/06/03/fauci-emails-show-his-flip-flopping-on-wearing-masks-to-fight-covid/.
Chambers, C. 2017. The Seven Deadly Sins of Psychology: A Manifesto for Reforming the Culture of Scientific Practice. Princeton, NJ: Princeton University Press.
Chappell, Bill. 2020. WHO Sets 6 Conditions For Ending A Coronavirus Lockdown. NPR, April 15, 2020. https://www.npr.org/sections/goatsandsoda/2020/04/15/834021103/who-sets-6-conditions-for-ending-a-coronavirus-lockdown.
Charlton, B. G. 1996. The uses and abuses of meta-analysis. Family Practice 13, 4: 397–401 https://doi.org/10.1093/fampra/13.4.397.
Chawla, D. S. 2020. Russian journals retract more than 800 papers after ‘bombshell’ in- vestigation. Science, January 8, 2020. https://www.sciencemag.org/news/2020/01/russian-journals-retract-more-800-papers-after-bombshell-investigation.
Chen, D-G., and Peace, K. E. 2013. Applied Meta-Analysis with R. 2013. Boca Raton, FL: Chapman & Hall.
Chikina, M., Pegden, W., and Recht, B. 2022. Re-analysis on the statistical sampling biases of a mask promotion trial in Bangladesh: a statistical replication. Trials 23: 786. https://doi.org/10.1186/s13063-022-06704-z.
Chin, V., Samia, N. I., Marchant, R., Rosen, O., Ioannidis, J. P. A., Tanner, M. A., and Cripps, S. 2020. A case study in model failure? COVID-19 daily deaths and ICU bed utilisation predictions in New York state. European Journal of Epidemiology 35, 8: 733–742. https://doi.org/10.1007/s10654-020-00669-6.
Chin, V., Ioannidis, J. P. A., Tanner, M. A., Cripps, S. 2021. Effect estimates of COVID-19 non-pharmaceutical interventions are non-robust and highly model-dependent. Journal of Clinical Epidemiology 136: 96–132. https://doi.org/10.1016/j.jclinepi.2021.03.014.
Claeson, M., and Hanson, S. 2021a. COVID-19 and the Swedish enigma. The Lancet 397, 10271: 259−261. http://doi.org/10.1016/S0140-6736(20)32750-1.
Claeson, M., and Hanson, S. 2021b. The Swedish COVID-19 strategy revisited. The Lancet 397, 10285: 1619. http://doi.org/10.1016/S0140-6736(21)00885-0.
Cleophas, T. J., and Zwinderman, A. H. 2015. Modern Meta-Analysis: Review and Update of Methodologies. New York, NY: Springer.
Clase, C. M., Fu, E. L., Joseph, M., et al. 2020. Cloth Masks May Prevent Transmission of COVID-19: An Evidence-Based, Risk-Based Approach. Annals of Internal Medicine 173, 6: 489–491. https://doi.org/10.7326/M20-2567.
Clyde, M. 2000. Model uncertainty and health effect studies for particulate matter. Environmetrics 11, 6: 745–763. https://doi.org/10.1002/1099-095X(200011/12)11:6<745::AID-ENV431>3.0.CO;2-N.
Cohen, J. 1994. The earth is round (p < .05). American Psychologist 49, 12: 997–1003. https://doi.org/10.1037/0003-066X.49.12.997.
Colbourn T. 2020. COVID-19: extending or relaxing distancing control measures. The Lancet Public Health 5, 5: e236–e237. https://doi.org/10.1016/S2468-2667(20)30072-4.
Coleman. L. 2019. How to Tackle the Unfolding Research Crisis. Quillette, December 14, 2019. https://quillette.com/2019/12/14/how-to-tackle-the-unfolding-research-crisis/.
Collins, G. S., and Wilkinson, J. 2021. Statistical issues in the development of COVID-19 prediction models. Journal of Medical Virology 93, 2: 624–625. https://doi.org/10.1002/jmv.26390.
Contopoulos-Ioannidis, D. G., Karvouni, A., Kouri, I., and Ioannidis, J. P. A. 2009. Reporting and interpretation of SF-36 outcomes in randomised trials: systematic review. British Medical Journal 338: a3006. https://doi.org/10.1136/bmj.a3006.
Cooper, R. 2019. Divestment in Fossil Fuels: A Preventive Public Health Strategy. Psychiatric Times 36, 4, April 12, 2019. https://www.psychiatrictimes.com/view/divestment-fossil-fuels-preventive-public-health-strategy.
Cordes, C. 1998. Overhead Rates for Federal Research Are as High as Ever, Survey Finds. The Chronicle of Higher Education, January 23, 1998. https://www.chronicle.com/article/Overhead-Rates-for-Federal/99293.
Coronado-Montoya, S., Levis, A. W., Kwakkenbos, L., Steele, R. J., Turner, E. H., Thombs, B. D. 2016. Reporting of Positive Results in Randomized Controlled Trials of Mindfulness-Based Mental Health Interventions. PLOS One 11, 4. https://doi.org/10.1371/journal.pone.0153220.
Couzin, J., and Unger, K. 2006. Cleaning Up the Paper Trail. Science 312, 5770: 38–43. https://doi.org/10.1126/science.312.5770.38.
Cox, L. A., Jr, and Popken, D. A. 2020. Should air pollution health effects assumptions be tested? Fine particulate matter and COVID-19 mortality as an example. Global Epidemiology 2: 100033. https://doi.org/10.1016/j.gloepi.2020.100033.
Crandall, C. S., and Sherman, J. W. 2016. On the scientific superiority of conceptual replications for scientific progress. Journal of Experimental Social Psychology 66: 93–99. http://dx.doi.org/10.1016/j.jesp.2015.10.002.
Curb, J. D., Hardy, R. J., Labarthe, D. R., Borhani, N. O., and Taylor, J. O. 1982. Reserpine and breast cancer in the Hypertension Detection and Follow-Up Program. Hypertension 4, 2: 307–311. https://doi.org/10.1161/01.hyp.4.2.307.
Dayaratna, K. 2020. Failures of an Influential COVID-19 Model Used to Justify Lockdowns. Heritage Foundation, May 18, 2020. https://www.heritage.org/public-health/commentary/failures-influential-covid-19-model-used-justify-lockdowns.
DerSimonian, R., and Laird, N. 1986. Meta-analysis in clinical trials. Controlled Clinical Trials 7, 3: 177–188. https://doi.org/10.1016/0197-2456(86)90046-2.
De Souto Barreto, P., Rolland, Y., Vellas, B., and Maltais, M. 2019. Association of Long-term Exercise Training With Risk of Falls, Fractures, Hospitalizations, and Mortality in Older Adults: A Systematic Review and Meta-analysis. JAMA Internal Medicine 179, 3: 394–405. https://doi.org/10.1001/jamainternmed.2018.5406.
De Vrieze, J. 2018. Meta-analyses were supposed to end scientific debates. Often, they only cause more controversy. Science, September 18, 2018. https://www.sciencemag.org/news/2018/09/meta-analyses-were-supposed-end-scientific-debates-often-they-only-cause-more.
Dickersin, K., Chan, S., Chalmers, T. C., Sacks, H. S., and Smith, H., Jr. 1987. Publication bias and clinical trials. Controlled Clinical Trials 8, 4: 343–353. https://doi.org/10.1016/0197-2456(87)90155-3.
Diener, E., and Biswas-Diener, R. 2018. The Replication Crisis in Psychology. In Introduction to Psychology, eds. R. Biswas-Diener & E. Diener. Champaign, IL: DEF Publishers. https://nobaproject.com/modules/the-replication-crisis-in-psychology. In https://nobaproject.com/textbooks/introduction-to-psychology-the-full-noba-collection.
Drabiak, K. 2021. The Intersection of Epidemiology and Legal Authority: Covid-19 Stay at Home Orders. Rutgers Journal of Law and Public Policy 18, 2: 1–69. https://rutgerspolicyjournal.org/sites/jlpp/files/Drabiak_Spring%2021.pdf.
Dreher, R. 2020. Masks As Condensed Symbols. The American Conservative, May 15, 2020. https://www.theamericanconservative.com/masks-as-condensed-symbols-coronavirus/.
Drummond, H. 2022. The Face Mask Cult. UK: CantusHead Books. https://hectordrummond.com/the-face-mask-cult/.
Editorial Board (Wall Street Journal). 2021. How Fauci and Collins Shut Down Covid Debate. They worked with the media to trash the Great Barrington Declaration. Wall Street Journal, December 21, 2021. https://www.wsj.com/articles/fauci-collins-emails-great-barrington-declaration-covid-pandemic-lockdown-11640129116?page=1.
Edwards, M. A., and Roy, S. 2017. Academic Research in the 21st Century: Maintaining Scientific Integrity in a Climate of Perverse Incentives and Hypercompetition. Environmental Engineering Science 34, 1: 51–61. https://dx.doi.org/10.1089%2Fees.2016.0223.
Ellenberg, J. 2014. How Not to Be Wrong: The Power of Mathematical Thinking. New York, NY: Penguin Press.
Engber, D. 2017. Daryl Bem Proved ESP Is Real. Which means science is broken. Slate, June 7, 2017. https://slate.com/health-and-science/2017/06/daryl-bem-proved-esp-is-real-showed-science-is-broken.html.
EPA (Environmental Protection Agency). N.D. Good Laboratory Practices Standards Compliance Monitoring Program. Compliance. United States Environmental Protection Agency. Accessed August 14, 2020. https://www.epa.gov/compliance/good-laboratory-practices-standards-compliance-monitoring-program.
Erikssen, J., Thaulow, E., Stormorken, H., Brendemoen, O., and Hellem, A. 1980. AB0 Blood Groups and Coronary Heart Disease (CHD). Thrombosis and Haemostasis 43, 2: 137–140. https://doi.org/10.1055/s-0038-1650035.
European Union. 2023. Health: Supporting Public Health in Europe, 2023. EU, Brussels, Belgium. https://european-union.europa.eu/priorities-and-actions/actions-topic/health_en.
Ewers, M., Ioannidis, J. P. A., and Plesnila, N. 2021. Access to data from clinical trials in the COVID-19 crisis: open, flexible, and time-sensitive. Journal of Clinical Epidemiology 130: 143–146. https://doi.org/10.1016/j.jclinepi.2020.10.008.
Fanelli, D. 2009. How Many Scientists Fabricate and Falsify Research? A Systematic Review and Meta-Analysis of Survey Data. PLOS One 4, 5: e5738. https://doi.org/10.1371/journal.pone.0005738.
Feinstein, A. R. 1988. Scientific Standards in Epidemiologic Studies of the Menace of Daily Life. Science 242, 4883: 1257–1263. https://doi.org/10.1126/science.3057627.
Fennelly, K. P. 2020. Particle sizes of infectious aerosols: implications for infection control. The Lancet Respiratory Medicine 8, 9: 914–924. https://doi.org/10.1016/S2213-2600(20)30323-4.
Ferguson, N. M., Cummings, D. A. T., Fraser, C., Cajka, J. C., Cooley, P. C., and Burke, D. S. 2006. Strategies for mitigating an influenza pandemic. Nature 442, 7101: 448–452. https://www.nature.com/articles/nature04795.
Ferguson, N. 2020. Reply to Giesecke. YouTube, April 25, 2020. https://www.youtube.com/watch?v=6cYjjEB3Ev8.
Fischer, A. J., and Ghelardi, G. 2016. The Precautionary Principle, Evidence-Based Medicine, and Decision Theory in Public Health Evaluation. Frontiers in Public Health 4: 107. https://doi.org/10.3389/fpubh.2016.00107.
Fisher, R. A. 1925. Statistical Methods for Research Workers. Edinburgh, UK: Oliver and Boyd. https://www.scribd.com/document/58873576/Fisher-R-a-1925-Statistical-Methods-for-Research-Workers.
Fisher, R. A. 1935. The Logic of Inductive Inference. Journal of the Royal Statistical Society 98, 1: 39–82. https://www.jstor.org/stable/pdf/2342435.pdf?seq=1.
Fisher, R. A. 1950. Statistical Methods for Research Workers, 11th ed., pp 99−101. Edinburgh, UK: Oliver and Boyd.
Florida. 2020. State Of Florida Office Of The Governor Executive Order Number 20-244 (Phase 3; Right to Work; Business Certainty; Suspension of Fines.) https://www.flgov.com/wp-content/uploads/orders/2020/EO_20-244.pdf.
Franco, A., Malhotra, N., and Simonovits, G. 2014. Publication bias in the social sciences: Unlocking the file drawer. Science 345, 6203: 1502–1505. https://doi.org/10.1126/science.1255484.
Freese, J., and Peterson, D. 2018. The Emergence of Statistical Objectivity: Changing Ideas of Epistemic Vice and Virtue in Science. Sociological Theory 36, 3: 289–313. https://doi.org/10.1177/0735275118794987.
Friedman, M., and Rosenman, R. H. 1959. Association of specific overt behavior pattern with blood and cardiovascular findings: blood cholesterol level, blood clotting time, incidence of arcus senilis, and clinical coronary artery disease. Journal of the American Medical Association 169, 12: 1286–1296. http://dx.doi.org/10.1001/jama.1959.03000290012005.
Furukawa, N. W., Brooks, J. T., and Sobel, J. 2020. Evidence Supporting Transmission of Severe Acute Respiratory Syndrome Coronavirus 2 While Presymptomatic or Asymptomatic. Emerging Infectious Diseases 26: 7. https://doi.org/10.3201/eid2607.201595.
GAO (U.S. Government Accountability Office). 2020. Disease modeling: How Math Can Help In A Pandemic. U.S. Government Accountability Office, June 9, 2020. https://www.gao.gov/blog/disease-modeling-how-math-can-help-pandemic.
Garrison, R. J., Havlik, R. J., Harris, R. B., Feinleib, M., Kannel, W. B., and Padgett, S. J. 1976. ABO blood group and cardiovascular disease: the Framingham study. Atherosclerosis 25, 2–3: 311–318. https://doi.org/10.1016/0021-9150(76)90036-8.
Gelman, A., and Loken, E. 2013. The garden of forking paths: Why multiple comparisons can be a problem, even when there is no “fishing expedition” or “p-hacking” and the research hypothesis. Miscellaneous Psychology Papers 140: 1272–1280. http://www.stat.columbia.edu/~gelman/research/unpublished/p_hacking.pdf.
Gelman, A., and Loken, E. 2014. The Statistical Crisis in Science. American Scientist 102, 6: 460–465. https://www.americanscientist.org/article/the-statistical-crisis-in-science.
Gelman, A., and Greenland, S. 2019. Are confidence intervals better termed “uncertainty intervals”? BMJ 366: I5381. https://pubmed.ncbi.nlm.nih.gov/31506269/.
Gerber, A. S., and Malhotra, N. 2008. Publication Bias in Empirical Sociological Research: Do Arbitrary Significance Levels Distort Published Results? Sociological Methods and Research 37, 1: 3–30. http://journals.sagepub.com/doi/abs/10.1177/0049124108318973.
Giesecke, J. 2020. Why lockdowns are the wrong policy: Swedish expert Prof. Johan Giesecke. YouTube, April 17, 2020. https://www.youtube.com/watch?v=bfN2JWifLCY.
Glaeser, E. L. 2006. Researcher incentives and empirical methods. NBER Technical Working Papers 0329, National Bureau of Economic Research, Inc. https://www.nber.org/papers/t0329.pdf.
Glass, G. V. 1976. Primary, Secondary, and Meta-Analysis of research. Educational Researcher 5, 10: 3–8. https://doi.org/10.3102/0013189X005010003.
Gobry, P.-E. 2016. Big Science is broken. The Week, April 18, 2016. https://theweek.com/articles/618141/big-science-broken.
Goh, Y., Tan, B. Y. Q., Bhartendu, C., Ong, J. J. Y., and Sharma, V. K. 2020. The face mask: How a real protection becomes a psychological symbol during Covid-19? Brain, Behavior, and Immunity 88: 1–5. https://doi.org/10.1016/j.bbi.2020.05.060.
Goldstein, B. D. 2001. The Precautionary Principle Also Applies to Public Health Actions. American Journal of Public Health 91, 9: 1358–1361. https://doi.org/10.2105/ajph.91.9.1358.
Goodman, S. N., Fanelli, D., and Ioannidis, J. P. A. 2016. What does research reproducibility mean? Science Translational Medicine 8, 341: 341ps12. https://doi.org/10.1126/scitranslmed.aaf5027.
Gostin, L. O., Friedman, E. A., and Wetter, S. A. 2020. Responding to Covid-19: How to Navigate a Public Health Emergency Legally and Ethically. Hastings Center Report 50, 2: 8−12. http://doi.org/10.1002/hast.1090.
Grossman, J., and Mackenzie, F. J. 2005. The Randomized Controlled Trial: gold standard, or merely standard? Perspectives in Biology and Medicine 48, 4: 516–534. https://doi.org/10.1353/pbm.2005.0092.
GS (Google Scholar). 2020a. https://scholar.google.com/scholar_lookup?hl=en-US&-publication_year=1993&author=+Westfall+PHauthor=+Young+SS&title=Resampling-based+multiple+testing%3A+examples+and+methods+for+p-value+adjustment, October 8, 2020.
GS (Google Scholar). 2020b. https://scholar.google.com/scholar?hl=en&as_sdt=5%2C33&sciodt=0%2C33&cites=2910987059377145085&scipsc=1&q=%22environmental+health+perspectives%22&btnG=, October 8, 2020.
Halsey, L. G., Curran-Everett, D., Vowler, S. L., and Drummond, G. B. 2015. The fickle P value generates irreproducible results. Nature Methods 12, 3: 179–185. https://doi.org/10.1038/nmeth.3288.
Han, Z. Y., Weng, W. G., and Huang, Q. Y. 2013. Characterizations of particle size distribution of the droplets exhaled by sneeze. Journal of the Royal Society Interface 10, 88: 20130560. http://dx.doi.org/10.1098/rsif.2013.0560.
Hardie, J. 2016. Why Face Masks Don’t Work: A Revealing Review. Oral Health, October 18, 2016. https://web.archive.org/web/20200509053953/https:/www.oralhealthgroup.com/features/face-masks-dont-work-revealing-review/.
Harris, R. 2017. Rigor Mortis: How Sloppy Science Creates Worthless Cures, Crushes Hope, and Wastes Billions. New York, NY: Basic Books.
Hart, J. 2022. The Twitter Blacklisting of Jay Bhattacharya. The Wall Street Journal, December 9, 2022. https://www.wsj.com/articles/the-twitter-blacklisting-of-jay-bhattacharya-medical-expert-covid-lockdown-stanford-doctor-shadow-banned-censorship-11670621083.
Hartgerink, C. H. J. 2017. “Reanalyzing Head et al. (2015): investigating the robustness of widespread p-hacking. PeerJ 5: e3068. https://doi.org/10.7717/peerj.3068.
Hayat, M. J., Powell, A., Johnson, T., and Cadwell, B. L. 2017. Statistical methods used in the public health literature and implications for training of public health professionals. PLOS One 12, 6: e0179032. https://doi.org/10.1371/journal.pone.0179032.
Head, M. L., Holman L., Lanfear, R., Kahn, A. T., and Jennions, M. D. 2015. The Extent and Consequences of P-Hacking in Science. PLOS Biology 13, 3: e1002106. https://doi.org/10.1371/journal.pbio.1002106.
Heinonen, O. P., Shapiro, S., Tuominen, L., and Turunen, M. I. 1974. Reserpine use in relation to breast cancer. The Lancet (London, England) 304, 7882: 675–677. https://doi.org/10.1016/s0140-6736(74)93259-0.
Hennen, A. 2019. The Credibility Issue in Nutrition Science Is a Sign for All of Higher Ed. The James G. Martin Center for Academic Renewal, November 27, 2019. https://www.jamesgmartin.center/2019/11/the-credibility-issue-in-nutrition-science-is-a-sign-for-all-of-higher-ed/.
Herby, J., Jonung, L., and Hanke, S. H. 2022. A literature review and meta-analysis of the effects of lockdowns on COVID-19 mortality. SAE./No. 210/May 2022. John Hopkins Institute for Applied Economics, Global Health, and the Study of Business Enterprise. https://sites.krieger.jhu.edu/iae/files/2022/06/A-Systematic-Review-and-Meta-Analysis-of-the-Effects-of-Lockdowns-of-COVID-19-Mortality-II.pdf.
Herold, E. 2018. Researchers Behaving Badly: Known Frauds Are “the Tip of the Iceberg.” Leapsmag. October 19, 2018. https://leapsmag.com/researchers-behaving-badly-why-scientific-misconduct-may-be-on-the-rise/.
Honein, M. A., Christie, A., Rose, D. A., et al. 2020. Summary of Guidance for Public Health Strategies to Address High Levels of Community Transmission of SARS-CoV-2 and Related Deaths, December 2020. MMWR Morb Mortal Wkly Rep. 2020 Dec 11; 69(49):1860–1867. https://pubmed.ncbi.nlm.nih.gov/33301434/.
Howick, J., Koletsi, D., Ioannidis, J. P. A., et al. 2022. Most healthcare interventions tested in Cochrane Reviews are not effective according to high quality evidence: a systematic review and meta-analysis. Journal of Clinical Epidemiology 148: 160–169. https://doi.org/10.1016/j.jclinepi.2022.04.017.
Hubbard, R. 2015. Corrupt Research: The Case for Reconceptualizing Empirical Management and Social Science. London, UK: Sage Publications.
Hung, H. M. J., O’Neill, R. T., Bauer, P., et al. 1997. The Behavior of the P-Value When the Alternative Hypothesis Is True. Biometrics 53, 1: 11–22. https://doi.org/10.2307/2533093.
Husch Blackwell. 2022. Assisting businesses with COVID-19 orders and helping them effectively continue operations. https://www.huschblackwell.com/state-by-state-covid-19-guidance.
IHME (IHME COVID-19 Forecasting Team). 2021. Modeling COVID-19 scenarios for the United States. Nature Medicine 27: 94–105. https://doi.org/10.1038/s41591-020-1132-9.
Inglesby, T. V., Nuzzo, J. B., O’Toole, T., and Henderson, D. A. 2006. Disease Mitigation Measures in the Control of Pandemic Influenza. Biosecurity and Bioterrorism: Biodefense, Strategy, Practice, and Science 4, 4: 366–375. https://doi.org/10.1089/bsp.2006.4.366.
Ioannidis, J. P. A. 2005. Why Most Published Research Findings Are False. PLOS Medicine 2, 8: e124. https://doi.org/10.1371/journal.pmed.0020124.
Ioannidis, J. P. A. 2008. Why Most Discovered True Associations Are Inflated. Epidemiology 19, 5: 640–648. https://doi.org/10.1097/EDE.0b013e31818131e7.
Ioannidis, J. P. A., Tarone, R. E., and McLaughlin, J. K. 2011. The False-positive to False-negative Ratio in Epidemiologic Studies. Epidemiology 22: 450–456. http://doi.org/10.1097/EDE.0b013e31821b506e.
Ioannidis, J. P. A. 2016. The Mass Production of Redundant, Misleading, and Conflicted Systematic Reviews and Meta-analyses. The Milbank Quarterly 94, 3: 485–514. https://doi.org/10.1111/1468-0009.12210.
Ioannidis, J. P. A. 2018. Meta-research: Why research on research matters. PLOS Biology 16, 3: e2005468. https://journals.plos.org/plosbiology/article?id=10.1371/journal.pbio.2005468.
Ioannidis, J. P. A. 2020a. Coronavirus disease 2019: The harms of exaggerated information and non-evidence-based measures. European Journal of Clinical Investigation 50, 4: e13222. Advance online publication. https://doi.org/10.1111/eci.13223.
Ioannidis, J. P. A. 2020b. A fiasco in the making? As the coronavirus pandemic takes hold, we are making decisions without reliable data. Stat, March 17, 2020. https://www.statnews.com/2020/03/17/a-fiasco-in-the-making-as-the-coronavirus-pandemic-takes-hold-we-are-making-decisions-without-reliable-data/.
Ioannidis J. P. A. 2020c. Global perspective of COVID-19 epidemiology for a full-cycle pandemic. European Journal of Clinical Investigation 50, 12: e13423. https://doi.org/10.1111/eci.13423.
Ioannidis, J. P. A., Axfors, C., and Contopoulos-Ioannidis, D. G. 2020d. Population-level COVID-19 mortality risk for non-elderly individuals overall and for non-elderly individuals without underlying diseases in pandemic epicenters. Environmental Research 188: 109890. https://doi.org/10.1016/j.envres.2020.109890.
Ioannidis, J. P. A. 2021a. Benefit of COVID-19 vaccination accounting for potential risk compensation. NPJ Vaccines 6, 1: 99. https://doi.org/10.1038/s41541-021-00362-z.
Ioannidis, J. P. A. 2021b. Infection fatality rate of COVID-19 inferred from seroprevalence data. Bulletin of the World Health Organization 99, 1: 19–33F. https://doi.org/10.2471/BLT.20.265892.
Ioannidis, J. P. A. 2021c. Over- and under-estimation of COVID-19 deaths. European Journal of Epidemiology 36, 6: 581–588. https://doi.org/10.1007/s10654-021-00787-9.
Ioannidis, J. P. A., Tezel, A., and Jagsi, R. 2021d. Overall and COVID-19-specific citation impact of highly visible COVID-19 media experts: bibliometric analysis. BMJ Open 11, 10: e052856. https://doi.org/10.1136/bmjopen-2021-052856.
Ioannidis, J. P. A. 2021e. Precision shielding for COVID-19: metrics of assessment and feasibility of deployment. BMJ Global Health 6, 1: e004614. https://doi.org/10.1136/bmjgh-2020-004614.
Ioannidis, J. P. A., Salholz-Hillel, M., Boyack, K. W., and Baas, J. 2021f. The rapid, massive growth of COVID-19 authors in the scientific literature. Royal Society Open Science 8, 9: 210389. https://doi.org/10.1098/rsos.210389.
Ioannidis, J. P. A. 2021g. Reconciling estimates of global spread and infection fatality rates of COVID-19: An overview of systematic evaluations. European Journal of Clinical Investigation 51, 5: e13554. https://doi.org/10.1111/eci.13554.
Ioannidis, J. P. 2022a. Citation impact and social media visibility of Great Barrington and John Snow signatories for COVID-19 strategy. BMJ Open 12, 2: e052891. https://doi.org/10.1136/bmjopen-2021-052891.
Ioannidis, J. P. A. 2022b. The end of the COVID-19 pandemic. European Journal of Clinical Investigation 52, 6: e13782. https://doi.org/10.1111/eci.13782.
Ioannidis, J. P. A. 2022c. Estimating conditional vaccine effectiveness. European Journal of Epidemiology 37, 9: 885–890. https://doi.org/10.1007/s10654-022-00911-3.
Ioannidis, J. P. A., Cripps, S., and Tanner, M. A. 2022d. Forecasting for COVID-19 has failed. International Journal of Forecasting 38, 2: 423–438. https://www.ncbi.nlm.nih.gov/pmc/articles/PMC7447267/.
Ioannidis, J. P. A. 2022e. High-cited favorable studies for COVID-19 treatments ineffective in large trials. Journal of Clinical Epidemiology 148: 1–9. https://doi.org/10.1016/j.jclinepi.2022.04.001.
Ioannidis, J. P. A., Bendavid, E., Salholz-Hillel, M., Boyack, K. W., and Baas, J. 2022f. Massive covidization of research citations and the citation elite. Proceedings of the National Academy of Sciences of the United States of America 119, 28: e2204074119. https://doi.org/10.1073/pnas.2204074119.
Ioannidis, J. P. A. 2022g. Pre-registration of mathematical models. Mathematical Biosciences 345: 108782. https://doi.org/10.1016/j.mbs.2022.108782.
IQA (Information Quality Act). 2001. Public Law 106—554, Sec. 515.
Jaeger, R. G., and Halliday, T. R. 1998. On Confirmatory versus Exploratory Research. Herpetologica 54, Supplement: S64–S66. https://www.jstor.org/stable/3893289?seq=1.
James, J. J. 2020. Lockdown or Lockup. Disaster Medicine and Public Health Preparedness 14, 6: e6−e7. https://doi.org/10.1017/dmp.2020.127.
Janiaud, P., Hemkens, L. G., and Ioannidis, J. P. A. 2021. Challenges and Lessons Learned From COVID-19 Trials: Should We Be Doing Clinical Trials Differently? Canadian Journal of Cardiology 37, 9: 1353–1364. https://doi.org/10.1016/j.cjca.2021.05.009.
Jefferson, T., Del Mar, C. B., Dooley, L., et al. 2020. Physical interventions to interrupt or reduce the spread of respiratory viruses. Cochrane Database of Systematic Reviews 11, 11, CD006207. http://doi.org/10.1002/14651858.CD006207.pub5.
Jenson, H. B. 2020. How did “flatten the curve” become “flatten the economy?” A perspective from the United States of America. Asian Journal of Psychiatry 51: 102165. http://doi.org/10.1016/j.ajp.2020.102165.
John, L. K., Loewenstein, G., and Prelec, D. 2012. Measuring the Prevalence of Questionable Research Practices With Incentives for Truth Telling. Psychological Science 23, 5: 524–532. https://doi.org/10.1177/0956797611430953.
Johnson, V. E. 2013. Revised standards for statistical evidence. Proceedings of the National Academy of Sciences of the United States of America 110, 48: 19313−19317. https://doi.org/10.1073/pnas.1313476110.
Joseph, A. 2020. Lancet, New England Journal retract Covid-19 studies, including one that raised safety concerns about malaria drugs. Statnews, June 4, 2020. https://www.statnews.com/2020/06/04/lancet-retracts-major-covid-19-paper-that-raised-safety-concerns-about-malaria-drugs/.
Kaiser, J. 2017. NIH plan to reduce overhead payments draws fire. Science, June 2, 2017. https://www.sciencemag.org/news/2017/06/nih-plan-reduce-overhead-payments-draws-fire.
Karadimas, P. 2022. COVID-19, Public Policy, and Public Choice Theory. The Independent Review 27, 2: 273−302. https://www.independent.org/publications/tir/article.asp?id=1751.
Kavvoura, F. K., Liberopoulos, G., and Ioannidis, J. P. A. 2007. Selection in Reported Epidemiological Risks: An Empirical Assessment. PLOS Medicine 4, 3: e79. http://doi.org/10.1371/journal.pmed.0040079.
Keller, V. 2015. Knowledge and the Public Interest, 1575–1725. Cambridge, MA: Cambridge University Press.
Kim, S. Y., and Kim, Y. 2018. The Ethos of Science and Its Correlates: An Empirical Analysis of Scientists’ Endorsement of Mertonian Norms. Science, Technology, and Society 23, 1: 1–24. https://doi.org/10.1177/0971721817744438.
Kim, M. S., Seong, D., Li, H., et al. 2022. Comparative effectiveness of N95, surgical or medical, and non-medical facemasks in protection against respiratory virus infection: A systematic review and network meta-analysis. Reviews in Medical Virology 32, 5: e2336. https://doi.org/10.1002/rmv.2336.
Kindzierski, W., Young, S., Meyer, T., et al. 2021. Evaluation of a Meta-Analysis of Ambient Air Quality as a Risk Factor for Asthma Exacerbation. Journal of Respiration 1: 173−196. https://doi.org/10.3390/jor1030017.
Klein, D., Stern, C., and Karlson, N. 2020. The underpinnings of Sweden’s permissive COVID regime. VoxEU, April 20, 2020. https://cepr.org/voxeu/columns/underpinnings-swedens-permissive-covid-regime.
Kretzschmar, M., and Wallinga, J. 2009. Mathematical Models in Infectious Disease Epidemiology. In Krämer, A., Kretzschmar, M., and Krickeberg, K., eds., Modern Infectious Disease Epidemiology. Statistics for Biology and Health. New York, NY: Springer. https://doi.org/10.1007/978-0-387-93835-6_12.
Kühberger, A., Fritz, A., and Scherndl, T. 2014. Publication Bias in Psychology: A Diagnosis Based on the Correlation between Effect Size and Sample Size. PLOS One 9, 9: e105825. https://doi.org/10.1371/journal.pone.0105825.
Kuhn, E. 2016. Science And Deference: The “Best Available Science” Mandate is A Fiction in the Ninth Circuit. Harvard Environmental Law Review, November 7, 2016. https://harvardelr.com/2016/11/07/elrs-science-and-deference-the-best-available-science-mandate-is-a-fiction-in-the-ninth-circuit/.
Kulldorff, M., Gupta, S., Bhattacharya, J. 2020. Great Barrington Declaration. https://gbdeclaration.org/.
Kupferschmidt, K. 2022. WHO’s departing chief scientist regrets errors in debate over whether SARS-CoV-2 spreads through air. Science, November 23, 2022. https://doi.org/10.1126/science.adf9731.
Labarthe, D. R., and O’Fallon, W. M. 1980. Reserpine and Breast Cancer. A Community-Based Longitudinal Study of 2,000 Hypertensive Women. Journal of the American Medical Association 243, 22: 2304–2310. https://jamanetwork.com/journals/jama/article-abstract/370217.
Lander, E. T. 2022. Protecting the Integrity of Government Science. White House Office of Science and Technology Policy (OSTP). https://www.epa.gov/scientific-integrity/protecting-integrity-government-science.
Lavezzo, E., Franchin, E., Ciavarella, C., et al. 2020. Suppression of a SARS-CoV-2 outbreak in the Italian municipality of Vo’. Nature 584: 425–429. https://doi.org/10.1038/s41586-020-2488-1.
Lee, P. N., Forey, B. A., and Coombs, K. J. 2012. Systematic review with meta-analysis of the epidemiological evidence in the 1900s relating smoking to lung cancer. BMC Cancer 12: 385. https://doi.org/10.1186/1471-2407-12-385.
Lee, J. J., Price, J. C., Jackson, W. M., Whittington, R. A., and Ioannidis, J. P. A. 2022. COVID-19: A Catalyst for Transforming Randomized Trials. Journal of Neurosurgical Anesthesiology 34, 1: 107–112. https://doi.org/10.1097/ANA.0000000000000804.
Levine, J. 2020. YouTube censors epidemiologist Knut Wittkowski for opposing lockdown. New York Post, May 16, 2020. https://nypost.com/2020/05/16/youtube-censors-epidemiologist-knut-wittkowski-for-opposing-lockdown/.
Levitt, M., Zonta, F., and Ioannidis, J. P. A. 2022a. Comparison of pandemic excess mortality in 2020–2021 across different empirical calculations. Environmental Research 213: 113754. https://doi.org/10.1016/j.envres.2022.113754.
Levitt, M., Zonta, F., and Ioannidis, J. P. A. 2022b. Excess death estimates from multiverse analysis in 2009-2021. medRxiv, 2022.09.21.22280219. https://doi.org/10.1101/2022.09.21.22280219.
Li, X., Huang, S., Jiao, A., et al. 2017. Association between ambient fine particulate matter and preterm birth or term low birth weight: An updated systematic review and meta-analysis. Environmental Pollution 227: 596–605. https://doi.org/10.1016/j.envpol.2017.03.055.
Lilienfeld, S. O. 2017. Psychology’s Replication Crisis and the Grant Culture: Righting the Ship. Perspectives on Psychological Science 12, 4: 660–664. https://doi.org/10.1177/1745691616687745.
Liu, I. T., Prasad, V, and Darrow, J. J. 2021. Evidence for Community Cloth Face Masking to Limit the Spread of SARS-CoV-2: A Critical Review. CATO Working Paper No. 64. November 8, 2021. The CATO Institute, Washington, DC. https://www.cato.org/sites/cato.org/files/2021-11/working-paper-64.pdf.
Lorenc, T., Felix, L., Petticrew, M., et al. 2016. Meta-analysis, complexity, and heterogeneity: a qualitative interview study of researchers’ methodological values and practices. Systematic Reviews 5, 1: 192. https://doi.org/10.1186/s13643-016-0366-6.
MacMahon, B., Yen, S., Trichopoulos, D., Warren, K., and Nardi, G. 1981. Coffee and Cancer of the Pancreas. New England Journal of Medicine 304: 630–633. https://doi.org/10.1056/nejm198103123041102.
Magness, P. W. 2020. Correction request for Nature-Medicine IHME paper, published on October 23. American Institute for Economic Research, October 24, 2020. https://www.aier.org/wp-content/uploads/2020/10/Magness-Nature-Medicine-letter.pdf.
Magness, P. W. 2021a. The Failure of Imperial College Modeling Is Far Worse than We Knew. American Institute for Economic Research, April 22, 2021. https://www.aier.org/article/the-failure-of-imperial-college-modeling-is-far-worse-than-we-knew/.
Magness, P. 2021b. The Failures of Pandemic Central Planning. October 1, 2021. http://doi.org/10.2139/ssrn.3934452.
Mandavilli, A. 2020. The Price for Not Wearing Masks: Perhaps 130,000 Lives. New York Times, October 23, 2020. https://www.nytimes.com/2020/10/23/health/covid-deaths.html.
Manuel, T. 2019. Why the Way We Use Statistical Significance Has Created a Crisis in Science. Science: The Wire, March 31, 2019. https://science.thewire.in/the-sciences/why-the-way-we-use-statistical-significance-has-created-a-crisis-in-science/.
Marcon, A., Nguyen, G., Rava, M., et al. 2015. A score for measuring health risk perception in environmental surveys. Science of The Total Environment 527–528: 270–278. https://doi.org/10.1016/j.scitotenv.2015.04.110.
Martino, J. P. 2017. Science Funding: Politics and Porkbarrel. New York, NY: Routledge.
Mathews, F., Johnson, P. J., and Neil, A. 2008. You are what your mother eats: evidence for maternal preconception diet influencing foetal sex in humans. Proceedings of the Royal Society B: Biological Sciences 275, 1643: 1661–1668. https://dx.doi.org/10.1098%2Frspb.2008.0105.
Martuzzi M. 2007. The precautionary principle: in action for public health. Occupational and Environmental Medicine 64, 9: 569–570. https://doi.org/10.1136/oem.2006.030601.
Mathews, F., Johnson, P. J., and Neil, A. 2009. Reply to Comment by Young et al. Proceedings of the Royal Society B: Biological Sciences 276, 1660: 1213–1214. https://doi.org/10.1098/rspb.2008.1781.
Mayes, L. C., Horwitz, R. I., and Feinstein, A. R. 1988. A Collection of 56 Topics with Contradictory Results in Case-Control Research. International Journal of Epidemiology 17, 3: 680–685. https://doi.org/10.1093/ije/17.3.680.
Mayo, D. G. 2018. Statistical Inference as Severe Testing: How to Get Beyond the Statistics Wars. Cambridge: Cambridge University Press.
McCambridge, J. 2007. A case study of publication bias in an influential series of reviews of drug education. Drug and Alcohol Review 26, 5: 463–468. https://doi.org/10.1080/09595230701494366.
McCormack, J., Vandermeer, B., and Allan, G. M. 2013. How confidence intervals become confusion intervals. BMC Medical Research Methodology 13: 134. https://doi.org/10.1186/1471-2288-13-134.
Melnick, E. R., and Ioannidis, J. P. A. 2020. Should governments continue lockdown to slow the spread of covid-19? BMJ (Clinical Research Ed.) 369: m1924. https://doi.org/10.1136/bmj.m1924.
Members, W.-C. J. M. 2020. Report of the WHO-China Joint Mission on Coronavirus Disease 2019 (COVID-19). World Health Organization (WHO). https://www.who.int/docs/default-source/coronaviruse/who-china-joint-mission-on-covid-19-final-report.pdf.
Menter, T., Haslbauer, J. D., Nienhold, R., et al. 2020. Postmortem examination of COVID-19 patients reveals diffuse alveolar damage with severe capillary congestion and variegated findings in lungs and other organs suggesting vascular dysfunction. Histopathology 77, 2: 198−209. https://doi.org/10.1111/his.14134.
Merton, R. K. 1973. The Sociology of Science: Theoretical and Empirical Investigations. Chicago, IL: The University of Chicago Press.
Meyerowitz, E. A., Richterman, A., Gandhi, R. T., and Sax, P. E. 2021. Transmission of SARS-CoV-2: A Review of Viral, Host, and Environmental Factors. Annals of Internal Medicine 174, 1: 69–79. https://doi.org/10.7326/M20-5008.
Michaels, P. J. 2008. Evidence for “Publication Bias” concerning Global Warming in Science and Nature. Energy & Environment 19, 2: 287−301. http://journals.sagepub.com/doi/abs/10.1260/095830508783900735?journalCode=eaea.
Michaud, D. S., Feder, K., and Voicescu, S. A., et al. 2018. Clarifications on the Design and Interpretation of Conclusions from Health Canada’s Study on Wind Turbine Noise and Health. Acoustics Australia 46: 99−110. https://doi.org/10.1007/s40857-017-0125-4.
Miller, I. 2022. Unmasked: The Global Failure of COVID Mask Mandates. Brentwood, TN: Post Hill Press.
Moffatt S., Mulloli T. P., Bhopal R., et al. 2000a. An Exploration of Awareness Bias in Two Environmental Epidemiology Studies. Epidemiology 11, 2: 199−208. https://doi.org/10.1097/00001648-200003000-00020.
Moffatt, S., and Bhopal, R. 2000b. Study on environmental hazards is flawed. British Medical Journal 320, 7244: 1274. https://doi.org/10.1136/bmj.320.7244.1274.
Montgomery, D. C., and Runger, G. C. 2003. Applied Statistics and Probability for Engineers. New York, NY: John Wiley & Sons.
Moolgavkar, S. H., McClellan, R. O., Dewanji, A., Turim, J., Luebeck, E. G., Edwards, M. 2013. Time-Series Analyses of Air Pollution and Mortality in the United States: A Subsampling Approach. Environmental Health Perspectives 121, 1: 73–78. https://doi.org/10.1289/ehp.1104507.
Mosher, S. W. 2022. Government censorship should scare us just as much as COVID once did. New York Post, September 17. 2022. https://nypost.com/2022/09/17/government-censorship-should-scare-us-as-much-as-covid-did/.
Mosley, V. M., and Wyckoff, R. W. G. 1946. Electron Micrography of the Virus of Influenza. Nature 157: 263. https://doi.org/10.1038/157263a0.
Mousavi, A., Yuan, Y., Masri, S., Barta, G., and Wu, J. 2021. Impact of 4th of July Fireworks on Spatiotemporal PM2.5 Concentrations in California Based on the PurpleAir Sensor Network: Implications for Policy and Environmental Justice. International Journal of Environmental Research and Public Health 18, 11: 5735. https://doi.org/10.3390/ijerph18115735.
Mouzo, J. 2022. Masks in schools are not associated with lower coronavirus transmission, says new study. El País, March 10, 2022. https://english.elpais.com/society/2022-03-11/masks-in-schools-are-not-associated-with-lower-coronavirus-transmission-says-new-study.html.
Nanda, A., Hung, I., Kwong, A., et al. 2021. Efficacy of surgical masks or cloth masks in the prevention of viral transmission: Systematic review, meta-analysis, and proposal for future trial. Journal of Evidence-Based Medicine 14, 2: 97–111. https://doi.org/10.1111/jebm.12424.
NASEM (National Academies of Sciences, Engineering, and Medicine). 1991. Environmental Epidemiology, Volume 1: Public Health and Hazardous Wastes. Washington, DC: The National Academies Press. https://doi.org/10.17226/1802.
NASEM (National Academies of Sciences, Engineering, and Medicine). 2016. Statistical Challenges in Assessing and Fostering the Reproducibility of Scientific Results: Summary of a Workshop. Washington, DC: The National Academies Press. https://www.nap.edu/read/21915/.
NASEM (National Academies of Science, Engineering, and Medicine). 2019. Reproducibility and Replicability in Science. Washington, DC: The National Academies Press. https://www.nap.edu/read/25303/.
Nelson, L. D., Simmons, J., and Simonsohn, U. 2018. Psychology’s Renaissance. Annual Review of Psychology 69: 511−534. https://doi.org/10.1146/annurev-psych-122216-011836.
Nelson, A. 2022. Doctors slam COVID government censorship exposed in ‘Twitter Files’: ‘On the road to totalitarianism.’ Fox News, December 27, 2022. https://www.foxnews.com/media/doctors-slam-covid-government-censorship-exposed-twitter-files-road-totalitarianism.
NIH (National Institutes of Health). 2009. Understanding a Common Cold Virus. National Institutes of Health, Bethesda, MD. https://www.nih.gov/news-events/nih-research-matters/understanding-common-cold-virus.
NIH (National Institutes of Health). 2017. Influenza virus biology. NIH Influenza Virus Resource help center, National Library of Medicine, Bethesda, MD. https://www.ncbi.nlm.nih.gov/genome/viruses/variation/help/flu-help-center/influenza-virus-biology/.
Nilsen, E. B., Bowler, D. E., and Linnell, J. D. C. 2020. Exploratory and confirmatory research in the open science era. Journal of Applied Ecology 57, 4: 842−847. https://doi.org/10.1111/1365-2664.13571.
Nissen, S. B., Magidson, T., Gross, K., et al. 2016. Publication bias and the canonization of false facts. eLife 5: e21451. https://doi.org/10.7554/elife.21451.
Nixon, K., Jindal, S., Parker, F., et al. 2022. An evaluation of prospective COVID-19 modelling studies in the USA: from data to science translation. The Lancet. Digital health 4, 10: e738–e747. https://doi.org/10.1016/S2589-7500(22)00148-0.
Normile, D. 2021. ‘Zero COVID’ is getting harder—but China is sticking with it. Science, November 17, 2021. https://www.science.org/content/article/zero-covid-getting-harder-china-sticking-it.
Nosek, B. A., and Errington, T. M. 2020. What is replication? PLOS Biology 18, 3: e3000691. https://doi.org/10.1371/journal.pbio.3000691.
O’Conner, D. S., Green, S., and Higgins, J. P. T. 2008. Cochrane Handbook for Systematic Reviews of Interventions. Chichester, UK: Wiley-Blackwell.
OECD (Organisation for Economic Co-operation and Development). 2020. OECD Policy Responses to Coronavirus (COVID-19). Flattening the COVID-19 peak: Containment and mitigation policies. Organisation for Economic Co-operation and Development (OECD). https://www.oecd.org/coronavirus/policy-responses/flattening-the-covid-19-peak-containment-and-mitigation-policies-e96a4226/.
Offen, N., Smith, E. A., and Malone, R. E. 2005. The perimetric boycott: a tool for tobacco control advocacy. Tobacco Control 14, 4: 272–277. https://doi.org/10.1136/tc.2005.011247.
Ogden, T. 2011. Lawyers Beware! The Scientific Process, Peer Review, and the Use of Papers in Evidence. The Annals of Occupational Hygiene 55, 7: 689–691. https://doi.org/10.1093/annhyg/mer056.
Ollila, H. M., Laine, L., Koskela, J., et al. 2020. Systematic review and meta-analysis to examine the use of face mask intervention in mitigating the risk of spread of respiratory infections and if the effect of face mask use differs in different exposure settings and age groups. PROSPERO 2020 CRD42020205523. https://www.crd.york.ac.uk/prospero/display_record.php?ID=CRD42020205523.
Ollila, H. M., Partinen, M., Koskela, J., et al. 2022. Face masks to prevent transmission of respiratory infections: Systematic review and meta-analysis of randomized controlled trials on face mask use. PLOS One 17, 12: e0271517. https://doi.org/10.1371/journal.pone.0271517.
Olson, C. M., Rennie, D., Cook, D., Dickersin, K., Flanagin, A., Hogan, J. W., Zhu, Q., Reiling, J., Pace, B. 2002. Publication Bias in Editorial Decision Making. Journal of the American Medical Association 287, 21: 2825–2828. https://doi.org/10.1001/jama.287.21.2825.
Open Science Collaboration [Brian Nosek, et al.]. 2015. Estimating the reproducibility of psychological science. Science 349, 6251: aac4716. https://doi.org/10.1126/science.aac4716.
Oreskes, N., and Conway, E. M. 2010. Merchants of Doubt: How a Handful of Scientists Obscured the Truth on Issues from Tobacco Smoke to Global Warming. New York, NY: Bloomsbury Press.
Pachetti, M., Marini, B., Giudici, F., et al. 2020. Impact of lockdown on Covid-19 case fatality rate and viral mutations spread in 7 countries in Europe and North America. Journal of Translational Medicine 18, 1: 338. https://doi.org/10.1186/s12967-020-02501-x.
Palpacuer, C., Hammas, K., Duprez, R., Laviolle, B., Ioannidis, J. P. A., Naudet, F. 2019. Vibration of effects from diverse inclusion/exclusion criteria and analytical choices: 9216 different ways to perform an indirect comparison meta-analysis. BMC Medicine 17, 174. https://doi.org/10.1186/s12916-019-1409-3.
Patel, A., and Jernigan, D. B. 2020. 2019-nCoV CDC Response Team. Initial Public Health Response and Interim Clinical Guidance for the 2019 Novel Coronavirus Outbreak — United States, December 31, 2019–February 4, 2020. Morbidity and Mortality Weekly Report (MMWR) 69, 5: 140–146. https://doi.org/10.15585/mmwr.mm6905e1.
Paterlini, M. 2020. ‘Closing borders is ridiculous’: the epidemiologist behind Sweden's controversial coronavirus strategy. Nature 580, 7805: 574. https://www.nature.com/articles/d41586-020-01098-x.
Peace, K. E., Yin, J. J., Rochani, H., Pandeya, S., and Young, S. S. 2018. A Serious Flaw in Nutrition Epidemiology: A Meta-Analysis Study. International Journal of Biostatistics 14, 2: 14(2):/j/ijb.2018.14.issue-2/ijb-2018-0079/ijb-2018-0079.xml. https://doi.org/10.1515/ijb-2018-0079.
Pellizzari, E., Lohr, K., Blatecky, A., and Creel, D. 2017. Reproducibility: A Primer on Semantics and Implications for Research. Research Triangle Park, NC: RTI Press. https://www.rti.org/sites/default/files/resources/18127052_Reproducibility_Primer.pdf.
Pezzullo, A. M., Ioannidis, J. P. A., and Boccia, S. 2022. Quality, integrity and utility of COVID-19 science: opportunities for public health researchers. European Journal of Public Health, ckac183. Advance online publication. https://doi.org/10.1093/eurpub/ckac183.
Pezzullo, A. M., Axfors, C., Contopoulos-Ioannidis, D. G., Apostolatos, A., and Ioannidis, J. P. A. 2023. Age-stratified infection fatality rate of COVID-19 in the non-elderly population. Environmental Research 216, Pt. 3: 114655. https://doi.org/10.1016/j.envres.2022.114655.
Pilz, S., and Ioannidis, J. P. A. 2022a. Does natural and hybrid immunity obviate the need for frequent vaccine boosters against SARS-CoV-2 in the endemic phase? European Journal of Clinical Investigation 52, 2: e13906. Advance online publication. https://doi.org/10.1111/eci.13906.
Pilz, S., Theiler-Schwetz, V., Trummer, C., Krause, R., and Ioannidis, J. P. A. 2022b. SARS-CoV-2 reinfections: Overview of efficacy and duration of natural and hybrid immunity. Environmental Research 209: 112911. https://doi.org/10.1016/j.envres.2022.112911.
Piquero, A. R., Jennings, W. G., Jemison, E., et al. 2021. Domestic violence during the COVID-19 pandemic - Evidence from a systematic review and meta-analysis. Journal of Criminal Justice 74: 101806. https://doi.org/10.1016/j.jcrimjus.2021.101806.
Popper, K. 1963. Conjectures and Refutations: The Growth of Scientific Knowledge. London: Routledge.
Prather, K. A., Wang, C. C., and Schooley, R. T. 2020. Reducing transmission of SARS-CoV-2. Science 368, 6498: 1422−1424. https://doi.org/10.1126/science.abc6197.
Prati, G., and Mancini, A. D. 2021. The psychological impact of COVID-19 pandemic lockdowns: a review and meta-analysis of longitudinal studies and natural experiments. Psychological Medicine 51: 201–211. https://doi.org/10.1017/S0033291721000015.
Prem, K., Liu, Y., Russell, T. W., et al., Centre for the Mathematical Modelling of Infectious Diseases COVID-19 Working Group. 2020. The effect of control strategies to reduce social mixing on outcomes of the COVID-19 epidemic in Wuhan, China: a modelling study. The Lancet. Public Health 5, 5: e261–e270. https://doi.org/10.1016/S2468-2667(20)30073-6.
Rabinowitz, P. M., Slizovskiy, I. B., Lamers, V., et al. 2015. Proximity to Natural Gas Wells and Reported Health Status: Results of a Household Survey in Washington County, Pennsylvania. Environmental Health Perspectives 123, 1: 21–26. https://doi.org/10.1289/ehp.1307732.
Randall, D., and Welser, C. 2018. The Irreproducibility Crisis of Modern Science: Causes, Consequences, and the Road to Reform. New York, NY: National Association of Scholars. https://www.nas.org/reports/the-irreproducibility-crisis-of-modern-science.
Redman, B. K. 2013. Research Misconduct Policy in Biomedicine: Beyond the Bad-Apple Approach. Cambridge, MA: The MIT Press.
Ridley, M., and Davis, D. 2020. Is the chilling truth that the decision to impose lockdown was based on crude mathematical guesswork? Rational Optimist, http://www.rationaloptimist.com/blog/lockdown-and-mathematical-guesswork/.
Ritchie, S. 2020. Science Fictions: How Fraud, Bias, Negligence, and Hype Undermine the Search for Truth. New York, NY: Henry Holt and Company.
Roberts, S., and Martin, M. A. 2010. Bootstrap-after-Bootstrap Model Averaging for Reducing Model Uncertainty in Model Selection for Air Pollution Mortality Studies. Environmental Health Perspectives 118, 1: 131–136. https://doi.org/10.1289/ehp.0901007.
Roche, G. C. 1994. The Fall of the Ivory Tower: Government Funding, Corruption, and the Bankrupting of American Higher Education. Washington, DC: Regnery.
Rothman, K. J. 1990. No Adjustments Are Needed for Multiple Comparisons. Epidemiology 1, 1: 43–46. https://www.jstor.org/stable/pdf/20065622.pdf?seq=1.
Rothstein, H. R., Sutton, A. J., and Borenstein, M. 2005. Publication Bias in Meta-analysis. In Publication Bias in Meta-Analysis: Prevention, Assessment and Adjustments, eds. Rothstein, H. R., Sutton, A. J., Borenstein, M. Chichester, UK: Wiley. 1–7. https://www.meta-analysis.com/downloads/Publication-Bias-Preface.pdf.
Sachs, J. D., Karim, S. S. A., Aknin, L., et al. 2022. The Lancet Commission on lessons for the future from the COVID-19 pandemic. The Lancet 400, 10359: 1224−1280. https://doi.org/10.1016/S0140-6736(22)01585-9.
Sample, I. 2019. Scientists top list of most trusted professions in US. The Guardian, August 2, 2019. https://www.theguardian.com/science/2019/aug/02/scientists-top-list-most-trusted-professions-us.
Sarewitz, D. 2012. Beware the creeping cracks of bias. Nature 485: 149. https://doi.org/10.1038/485149a.
Schachtman, N. 2011. Misplaced Reliance On Peer Review to Separate Valid Science From Nonsense. Tortini, August 14, 2011. http://schachtmanlaw.com/misplaced-reliance-on-peer-review-to-separate-valid-science-from-nonsense/.
Schlette, S., Henke, K.-D., and Klenk, T. 2020. Germany’s Response to the Coronavirus Pandemic – The August update. https://www.cambridge.org/core/blog/2020/04/08/germanys-response-to-the-coronavirus-pandemic/.
Scheuch, G. 2020. Breathing Is Enough: For the Spread of Influenza Virus and SARS-CoV-2 by Breathing Only. Journal of Aerosol Medicine and Pulmonary Drug Delivery 33, 4: 230−234. https://doi.org/10.1089/jamp.2020.1616.
Schippers, M. C., Ioannidis, J. P. A., and Joffe, A. R. 2022. Aggressive measures, rising inequalities, and mass formation during the COVID-19 crisis: An overview and proposed way forward. Frontiers in Public Health 10: 950965. https://doi.org/10.3389/fpubh.2022.950965.
Schönweitz, F. B., Eichinger, J., Kuiper, J. M., et al. 2022. The social meanings of artefacts: Face masks in the COVID-19 pandemic. Frontiers in Public Health 10: 829904. https://doi.org/10.3389/fpubh.2022.829904.
Schroter, S., Black, N., Evans, S., Godlee, F., Osorio, L., Smith, R. 2008. What errors do peer reviewers detect, and does training improve their ability to detect them? Journal of the Royal Society of Medicine 101, 10: 507–14. https://doi.org/10.1258/jrsm.2008.080062.
Schwarzkopf, S. 2014. The Pipedream of Preregistration. The Devil’s Neuroscientist, November 28, 2014. https://devilsneuroscientist.wordpress.com/2014/11/28/the-pipedream-of-preregistration/.
Schweder, T., and Spjøtvoll, E. 1982. Plots of P-values to evaluate many tests simultaneously. Biometrika 69, 3: 493–502. https://doi.org/10.1093/biomet/69.3.493.
Sedgwick, P. 2014. Understanding why “absence of evidence is not evidence of absence.” British Medical Journal 349: g4751. https://doi.org/10.1136/bmj.g4751.
Seifert, J., Meissner, C., Birkenstock, A., et al. 2021. Peripandemic psychiatric emergencies: impact of the COVID-19 pandemic on patients according to diagnostic subgroup. European Archives of Psychiatry and Clinical Neuroscience 271, 2: 259–270. https://doi.org/10.1007/s00406-020-01228-6.
Seong, D., Shin, J., and Kim, M. 2020. Comparative efficacy of N95, surgical, medical, and non-medical facemasks in respiratory virus transmission prevention: a living systematic review and network meta-analysis. PROSPERO 2020 CRD42020214729. https://www.crd.york.ac.uk/prospero/display_record.php?ID=CRD42020214729.
Shahbaz, A., and Funk, A. 2020. Information Isolation: Censoring the COVID-19 Outbreak. Freedom House. https://freedomhouse.org/report/report-sub-page/2020/information-isolation-censoring-covid-19-outbreak.
Shapin, S. 1994. A Social History of Truth: Civility and Science in Seventeenth-Century England. Chicago, IL: The University of Chicago Press.
Shapiro, S. 2004. Looking to the 21st century: have we learned from our mistakes, or are we doomed to compound them? Pharmacoepidemiology and Drug Safety 13, 4: 257–265. https://doi.org/10.1002/pds.903.
Shekelle, R. B., Hulley, S. B., Neaton, J. D., et al. 1985a. The MRFIT behavior pattern study: II. Type A behavior and incidence of coronary heart disease. American Journal of Epidemiology 122, 4: 559–570. https://doi.org/10.1093/oxfordjournals.aje.a114135.
Shekelle, R. B., Gale, M., and Norusis, M. 1985b. Type A score (Jenkins activity Survey) and risk of recurrent coronary heart disease in the aspirin myocardial infarction study. The American Journal of Cardiology 56, 4: 222–225. https://doi.org/10.1016/0002-9149(85)90838-0.
Shusterman, D. 1992. Critical Review: The Health Significance of Environmental Odor Pollution. Archives of Environmental & Occupational Health 47: 76–87. https://doi.org/10.1080/00039896.1992.9935948.
Simonsohn, U., Nelson, L. D., and Simmons, J. P. 2014. p-Curve and Effect Size: Correcting for Publication Bias Using Only Significant Results. Perspectives on Psychological Science 9, 6: 666–681. https://doi.org/10.1177/1745691614553988.
Smith, R. 2010. Classical peer review: an empty gun. Breast Cancer Research 12: S13. https://doi.org/10.1186/bcr2742.
Smith-Sivertsen, T., Tchachtchine, V., and Lund, E. 2000. Self-Reported Airway Symptoms in a Population Exposed to Heavy Industrial Pollution: What Is the Role of Public Awareness? Epidemiology 11, 6: 739–740. https://doi.org/10.1097/00001648-200011000-00027.
Stanley, W. M. 1944. The size of the influenza virus. Journal of Experimental Medicine 79, 3: 267–283. https://doi.org/10.1084/jem.79.3.267.
Stott, E. J., and Killington, R. A. 1972. Rhinoviruses. Annual Review of Microbiology 26, 1: 503−524. https://doi.org/10.1146/annurev.mi.26.100172.002443.
Streiner, D. L. 2018. Statistics Commentary Series, Commentary No. 27: P-Hacking. Journal of Clinical Psychopharmacology 38, 4: 286−288. https://doi.org/10.1097/JCP.0000000000000901.
Stroup, D. F., Berlin, J. A., Morton, S. C., et al. 2000. Meta-analysis of Observational Studies in Epidemiology: A Proposal for Reporting. Journal of the American Medical Association 283, 15: 2008–2012. https://doi.org/10.1001/jama.283.15.2008.
Taleb, N. N. 2018. Skin in the Game: Hidden Asymmetries in Daily Life. New York, NY: Penguin.
Tanner, S. 2015. Evidence of False Positives in Research Clearinghouses and Influential Journals: An Application of P-Curve to Policy Research. https://gspp.berkeley.edu/assets/uploads/research/pdf/Tanner_p-curve_paper_v2.0.pdf.
Tellier, R. 2006. Review of Aerosol Transmission of Influenza A Virus. Emerging Infectious Diseases 12, 11: 1657–1662. https://www.ncbi.nlm.nih.gov/pmc/articles/PMC3372341/.
Tellier, R. 2009. Aerosol transmission of influenza A virus: a review of new studies. Journal of the Royal Society Interface 6: S783–S790. https://doi.org/10.1098/rsif.2009.0302.focus.
Terris, M. 2011. A Social Policy for Health. American Journal of Public Health 101, 2: 250–252. https://doi.org/10.2105/ajph.101.2.250.
Thornton, A., and Lee, P. 2000. Publication bias in meta-analysis: its causes and consequences. Journal of Clinical Epidemiology 53, 2: 207–216. https://doi.org/10.1016/S0895-4356(99)00161-4.
Trafimow, D., Amrhein, V., Areshenkoff, C. N., Barrera-Causil, C. J., et al. 2018. Manipulating the Alpha Level Cannot Cure Significance Testing. Frontiers in Psychology 9: 699. https://doi.org/10.3389/fpsyg.2018.00699.
Tran, T. Q., Mostafa, E. M., Ravikulan, R., et al. 2020. Efficacy of facemasks against airborne infectious diseases: a systematic review and network meta-analysis of randomized-controlled trials. PROSPERO 2020 CRD42020178516. https://www.crd.york.ac.uk/prospero/display_record.php?ID=CRD42020178516.
Tran, T. Q., Mostafa, E. M., Tawfik, G. M., et al. 2021. Efficacy of face masks against respiratory infectious diseases: a systematic review and network analysis of randomized-controlled trials. Journal of Breath Research 15: 047102. https://doi.org/10.1088/1752-7163/ac1ea5.
UMD (University of Maryland). 2022. Global COVID-19 Trends and Impact Survey. Joint Program in Survey Methodology, University of Maryland, College Park, MD. https://jpsm.umd.edu/landingtopic/global-covid-19-trends-and-impact-survey.
Verity, R., Okell, L. C., Dorigatti, I., et al. 2020. Estimates of the severity of coronavirus disease 2019: a model-based analysis. The Lancet: Infectious Diseases 20, 6: P669−P677. https://doi.org/10.1016/S1473-3099(20)30243-7.
Villeneuve, P. J., Ali, A., Challacombe, L., and Hebert, S. 2009. Intensive hog farming operations and self-reported health among nearby rural residents in Ottawa, Canada. BMC Public Health 9: 330. https://doi.org/10.1186/1471-2458-9-330.
Vishwamitra, N., et al. 2021. On Analyzing COVID-19-related Hate Speech Using BERT Attention. 2020 19th IEEE International Conference on Machine Learning and Applications (ICMLA). 669–76. https://par.nsf.gov/servlets/purl/10223825.
Vogel, G. 2020. “‘It's been so, so surreal.’ Critics of Sweden's lax pandemic policies face fierce backlash.” [Retitled: “Sweden’s Gamble.”] Science, October 6, 2020. https://www.science.org/content/article/it-s-been-so-so-surreal-critics-sweden-s-lax-pandemic-policies-face-fierce-backlash.
Volokh, E. 2023. No-Lockdown Sweden Seemingly Tied for Lowest All-Causes Mortality in OECD Since COVID Arrived. The Volokh Conspiracy, January 10, 2023, https://reason.com/volokh/2023/01/10/no-lockdown-sweden-seemingly-tied-for-lowest-all-causes-mortality-in-oecd-since-covid-arrived/.
Wagenmakers, E.-J., Wetzels, R., Borsboom, D., et al. 2012. An Agenda for Purely Confirmatory Research. Perspectives on Psychological Science 7, 6: 632–638. https://doi.org/10.1177/1745691612463078.
Walker, P. G. T., Whittaker, C., Watson, O. J., et al. 2020. The impact of COVID-19 and strategies for mitigation and suppression in low- and middle-income countries. Science 369, 6502: 413–422. https://doi.org/10.1126/science.abc0035.
Wang, C. C., Prather, K. A., Sznitman, J., et al. 2021. Airborne transmission of respiratory viruses. Science 373, 6558: eabd9149. https://doi.org/10.1126/science.abd9149.
Westfall, P. H. 1985. Simultaneous Small-Sample Multivariate Bernoulli Confidence Intervals. Biometrics 41, 4: 1001–1013. https://www.jstor.org/stable/2530971.
Westfall, P. H., and Young, S. S. 1993. Resampling-Based Multiple Testing. New York, NY: John Wiley & Sons.
WHO (World Health Organization). 2019. Non-pharmaceutical public health measures for mitigating the risk and impact of epidemic and pandemic influenza; Annex: Report of systematic literature reviews. No. WHO/WHE/IHM/GIP/2019.1. WHO, Geneva. https://apps.who.int/iris/bitstream/handle/10665/329439/WHO-WHE-IHM-GIP-2019.1-eng.pdf.
Wittkowski, K. M. 2020. The first three months of the COVID-19 epidemic: Epidemiological evidence for two separate strains of SARS-CoV-2 viruses spreading and implications for prevention strategies. medRxiv. https://doi.org/10.1101/2020.03.28.20036715.
Wittkowski, K. M. 2022. Knut Wittkowski Interview-Removed from YouTube. Perspectives on the Pandemic. https://www.facebook.com/perspectivesonthepandemic/videos/knut-wittkowski-interview-removed-from-youtube-perspectives-on-the-pandemic-epis/258992005297175/.
Wojick, D. E., and Michaels, P. J. 2015. Is the Government Buying Science or Support? A Framework Analysis of Federal Funding-induced Biases. Cato Working Paper No. 29. Washington, DC: Cato Institute. https://www.cato.org/sites/cato.org/files/pubs/pdf/working-paper-29.pdf.
World Population Review. 2020. Top 10 Countries with Highest Human Development Index, 2020 Report – United Nations; Standard of Living by Country; Quality of Life by Country. World Population Review, Walnut, CA, 2023. https://web.archive.org/web/20201028150533/https://worldpopulationreview.com/country-rankings/standard-of-living-by-country.
Xiao, J., Shiu, E. Y. C., Gao, H., et al. 2020. Nonpharmaceutical Measures for Pandemic Influenza in Nonhealthcare Settings—Personal Protective and Environmental Measures. Emerging Infectious Diseases 26, 5: 967−975. https://doi.org/10.3201/eid2605.190994.
Yong, E. 2018. Psychology’s Replication Crisis Is Running Out of Excuses. The Atlantic, November 19, 2018. https://www.theatlantic.com/science/archive/2018/11/psychologys-replication-crisis-real/576223/.
Young, S. S., Bang, H., and Oktay, K. 2009. Cereal-induced gender selection? Most likely a multiple testing false positive. Proceedings of the Royal Society B: Biological Sciences 276, 1660: 1211−1212. https://doi.org/10.1098/rspb.2008.1405.
Young, S. S., and Karr, A. 2011. Deming, Data and Observational Studies: A Process out of Control and Needing Fixing. Significance 8, 3: 116−120. https://doi.org/10.1111/j.1740-9713.2011.00506.x.
Young, S. S. 2017. Air quality environmental epidemiology studies are unreliable. Regulatory Toxicology and Pharmacology 86: 177−180. http://dx.doi.org/10.1016/j.yrtph.2017.03.009.
Young, S. S., and Miller, H. 2018. Junk Science Has Become a Profitable Industry. Who Will Stop It? Real Clear Science, November 26, 2018. https://www.realclearscience.com/articles/2018/11/26/junk_science_has_become_a_profitable_industry_110810.html.
Young, S. S., and Kindzierski, W. B. 2019a. Combined background information for meta-analysis evaluation. arXiv. https://arxiv.org/abs/1808.04408.
Young, S. S., and Kindzierski, W. B. 2019b. Evaluation of a meta-analysis of air quality and heart attacks, a case study. Critical Reviews in Toxicology 49, 1: 85−94. https://doi.org/10.1080/10408444.2019.1576587.
Young, S. S., Acharjee, M. K., and Das, K. 2019c. The reliability of an environmental epidemiology meta-analysis, a case study. Regulatory Toxicology and Pharmacology 102: 47–52. https://doi.org/10.1016/j.yrtph.2018.12.013.
Young, S. S., Kindzierski, W. B., and Randall, D. 2021a. Shifting Sands, Unsound Science and Unsafe Regulation Report 1. Keeping Count of Government Science: P-Value Plotting, P-Hacking, and PM2.5 Regulation. New York, NY: National Association of Scholars. https://www.nas.org/reports/shifting-sands-report-i.
Young, S. S., and Kindzierski, W. B. 2021b. Standard meta-analysis methods are not robust. arXiv. https://arxiv.org/abs/2110.14511 [stat.ME].
Young, S. S., Cheng, K.-C., Chen, J. H., et al. 2022a. Reliability of a Meta-analysis of Air Quality−Asthma Cohort Studies. International Journal of Statistics and Probability, 11, 2: 61−76. https://doi.org/10.5539/ijsp.v11n2p61.
Young, S. S., and Kindzierski, W. B. 2022b. Research Plan Lockdowns. Researchers.One. https://researchers.one/articles/22.11.00005v1.
Young, S. S., Kindzierski, W. B., and Randall, D. 2022c. Shifting Sands, Unsound Science and Unsafe Regulation Report 2. Flimsy Food Findings: Food Frequency Questionnaires, False Positives, and Fallacious Procedures in Nutritional Epidemiology. New York, NY: National Association of Scholars. https://www.nas.org/reports/shifting-sands-report-ii.
Young, S. S., and Kindzierski, W. B. 2023a. Reproducibility of Health Claims in Meta-Analysis Studies of COVID Quarantine (Stay-at-Home) Orders. International Journal of Statistics and Probability 12, 1: 54–65. https://doi.org/10.5539/ijsp.v12n1p54.
Young, S. S., and Kindzierski, W. B. 2023b. Statistical reproducibility of meta-analysis research claims for medical mask use in community settings to prevent COVID infection. arXiv. https://arxiv.org/abs/2301.09189.
Zavalis, E. A., and Ioannidis, J. P. A. 2022. A meta-epidemiological assessment of transparency indicators of infectious disease models. PLOS One 17, 10: e0275380. https://doi.org/10.1371/journal.pone.0275380.
Zeeman, E. C. 1976. Catastrophe Theory. Scientific American 234, 4: 65–83. https://doi.org/10.1038/scientificamerican0476-65.
Zhu, N., Zhang, D., Wang, W., et al. 2020. China Novel Coronavirus Investigating and Research Team: A Novel Coronavirus from Patients with Pneumonia in China, 2019. New England Journal of Medicine 382: 727–733. https://doi.org/10.1056/NEJMoa2001017.
Zhu, Y., Li, Y., and Xu, X. 2022. Suicidal ideation and suicide attempts in psychiatric patients during the COVID-19: A systematic review and meta-analysis. Psychiatry Research 317: 114837. https://doi.org/10.1016/j.psychres.2022.114837.
Zimring, J. C. 2019. What Science Is and How It Really Works. Cambridge, MA: Cambridge University Press.
1 David Randall and Christopher Welser, The Irreproducibility Crisis of Modern Science: Causes, Consequences, and the Road to Reform (National Association of Scholars, 2018), https://www.nas.org/reports/the-irreproducibility-crisis-of-modern-science.
2 Fixing Science: Practical Solutions for the Irreproducibility Crisis, YouTube, https://www.youtube.com/watch?v=eee6KloEUR4&list=PL-mariB2b6NugvvjAFeAjK-_-Y6wXCkvM; “Conference Follow-up: Fixing Science,” National Association of Scholars, February 19, 2020, https://www.nas.org/blogs/article/conference-follow-up-fixing-science.
3 “UPDATED: NAS Public Comment on Strengthening Transparency in Regulatory Science,” National Association of Scholars, June 19, 2018, https://www.nas.org/blogs/article/updated_nas_public_comment_on_strengthening_transparency_in_regulatory_scie; Peter Wood, “NAS Comments on EPA's Proposed Supplemental Notice of Proposed Rulemaking,” March 23, 2020, https://www.nas.org/blogs/article/nas-comment-on-epas-proposed-supplemental-notice-of-proposed-rulemaking; “Comments on EPA’s Final Rule, ‘Strengthening Transparency’,” National Association of Scholars, January 12, 2021, https://www.nas.org/blogs/article/nas-comments-on-epas-final-rule-strengthening-transparency.
4 “Episode #51: Rabble Rousing with Lee Jussim,” https://www.nas.org/blogs/media/episode-51-rabble-rousing-with-lee-jussim; “Legally Wrong: When Courts and Science Meet with Nathan Schachtman,” https://www.nas.org/blogs/media/legally-wrong-when-politics-and-science-meet-with-nathan-schactman; David Randall, “Bad Science Makes for Bad Government,” National Association of Scholars, September 19, 2019, https://www.nas.org/blogs/article/bad-science-makes-for-bad-government; Edward Reid, “Irreproducibility and Climate Science,” National Association of Scholars, May 17, 2018, https://www.nas.org/blogs/article/irreproducibility_and_climate_science.
5 Stanley Young, Warren Kindzierski, and David Randall, Shifting Sands: Report I Keeping Count of Government Science: P-Value Plotting, P-Hacking, and PM2.5 Regulation (National Association of Scholars, 2021), https://www.nas.org/reports/shifting-sands-report-i.
6 Andrew Gelman and Eric Loken, “The garden of forking paths: Why multiple comparisons can be a problem, even when there is no “fishing expedition” or “p-hacking” and the research hypothesis,” Miscellaneous Psychology Papers 140 (2013): 1272–1280. http://www.stat.columbia.edu/~gelman/research/unpublished/p_hacking.pdf.
7 Cf. William M. Briggs Uncertainty: The Soul of Modeling, Probability, & Statistics (New York, NY: Springer, 2016); especially the We Must Do Something Fallacy and the Epidemiologist Fallacy.
8 NAS Statement on the Response to COVID-19 in Higher Education, November 19, 2021, https://www.nas.org/blogs/statement/nas-statement-on-the-response-to-covid-19-in-higher-education.
9 Lavezzo (2020); Members (2020).
10 Sachs (2022).
11 Normile (2021); OECD (2020).
12 Verity (2020).
13 Adam (2020).
14 Klein (2020).
15 Paterlini (2020).
16 Ferguson (2020); Giesecke (2020); Levine (2020); Vogel (2020); Wittkowski (2020); Wittkowski (2022).
17 Volokh (2023).
18 Florida (2020).
19 AIER (2020); and see Allen (2022).
20 Karadimas (2022).
21 Young (2021a); Young (2022c).
22 IQA (2001); Kuhn (2016).
23 Benjamini (1995); Westfall (1993).
24 Benjamini (1995); Westfall (1993).
25 Young (2021a).
26 NASEM (2019).
27 Rothman (1990).
28 Baker (2016); Sarewitz (2012).
29 Randall (2018); Young (2021a).
30 Al-Marzouki (2005); Couzin (2006); Redman (2013); Ritchie (2020).
31 Buchanan (2004); Young (2021a).
32 Baker (2016); Sarewitz (2012).
33 Boos (2011).
34 Briggs (2017); Briggs (2019); Chambers (2017); Clyde (2000); Gelman (2014); Harris (2017); Hubbard (2015).
35 Benjamin (2018); Johnson (2013).
36 NASEM (2016); NASEM (2019).
37 Baker (2016).
38 Begley (2012); and see Diener (2018) [psychology]; Franco (2014) [social sciences]; Gerber (2008) [sociology]; and Michaels (2008) [climate science].
39 Gelman (2014).
40 NASEM (2016).
41 Young (2021a).
42 Young (2022c).
43 Confounded Error does not address p-hacking.
44 Popper (1963).
45 Adiga (2020); Biggerstaff (2022); Brauer (2017); Ferguson (2006); GAO (2020); Kretzschmar (2009).
46 Adiga (2020); GAO (2020).
47 E.g., Colbourn (2020); Pachetti (2020); Prem (2020); Verity (2020); Walker (2020).
48 Adiga (2020).
49 Bertozzi (2020).
50 Biggerstaff (2022).
51 Chappell (2020).
52 For critiques of lockdown recommendations see Bendavid (2021); Chin (2021); Ioannidis (2021e); Melnick (2020).
53 Collins (2021).
54 Nixon (2022).
55 Ioannidis (2022d); and see Chin (2020); Howick (2022); Levitt (2022a); Levitt (2022b); Zavalis (2022).
56 Ioannidis (2022d).
57 Ioannidis (2022d).
58 Ioannidis (2022d).
59 E.g., Axfors (2022); Ballin (2022); Bendavid (2021); Boccia (2020); Chin (2020); Chin (2021); Ewers (2021); Howick (2022); Ioannidis (2020a); Ioannidis (2020b); Ioannidis (2020c); Ioannidis (2020d); Ioannidis (2021a); Ioannidis (2021b); Ioannidis (2021c); Ioannidis (2021d); Ioannidis (2021e); Ioannidis (2021f); Ioannidis (2021g); Ioannidis (2022a); Ioannidis (2022b); Ioannidis (2022c); Ioannidis (2022d); Ioannidis (2022e); Ioannidis (2022f); Ioannidis (2022g); Janiaud (2021); Lee (2022); Levitt (2022a); Levitt (2022b); Melnick (2020); Pezzullo (2022); Pezzullo (2023); Pilz (2022a); Pilz (2022b); Schippers (2022); Zavalis (2022).
60 Ioannidis (2018).
61 Florida (2020); Inglesby (2006); Members (2020); Paterlini (2020).
62 Dreher (2020); Goh (2020); Schönweitz (2022).
63 Ioannidis (2020a). For critiques of lockdown recommendations, also see Bendavid (2021); Chin (2021); Ioannidis (2021d); Ioannidis (2021e); Melnick (2020).
64 Altman (2011a); Altman (2011b).
65 Schweder (1982).
66 An assumption of meta-analysis (regardless of how test statistics were derived, i.e., different models) is that heterogeneity among test statistics from relevant base papers is randomly distributed around the true value. See Charlton (1996). The p-value plot can be used to assess heterogeneity of the test statistics. Our experience in practice is that plots quite readily show a set of null test statistics aligned in a near 45-degree line; not perfect, but distinctly different from a set of test statistics for a true effect or where bias is at play in base papers.
67 Bordewijk (2020); Hung (1997); Schweder (1982).
68 One does not need the universe of p-values to show a null effect. Kindzierski (2021).
69 Schweder (1982). For p-value plot formation and other analysis details, see also Young (2018); Young (2019a).
70 Nelson (2018).
71 Randall (2018); Ritchie (2020).
72 Chambers (2017); Ellenberg (2014); Harris (2017); Hubbard (2015); Streiner (2018).
73 Young (2021a).
74 Carter (2019).
75 Gao (2020); Members (2020).
76 For state and local COVID-19 lockdown policies in America, see Husch Blackwell (2022).
77 Gostin (2020); Jenson (2020), Magness (2021a); Magness (2021b).
78 Schweder (1982).
79 On November 20, 2022, we used the terms ((covid[Title]) OR (sars-cov-2[Title]) AND (2020:2023[pdat])) AND (meta-analysis[Title] AND (2020:2023[pdat])).
80 Young (2022b).
81 Young (2022b).
82 Herby (2022).
83 Prati (2021).
84 Piquero (2021).
85 Zhu (2022).
86 Herby (2022).
87 Prati (2021).
88 Piquero (2021).
89 Zhu (2022).
90 Altman (2011a); Altman (2011b).
91 Young (2022b).
92 Schweder (1982).
93 Herby (2022).
94 Prati (2021).
95 Boos (2011).
96 Alderson (2004); Altman (1995); Sedgwick (2014).
97 Piquero (2021).
98 Zhu (2022).
99 Seifert (2021).
100 Inglesby (2006).
101 James (2020).
102 AIER (2020).
103 CDC (2022); Lavezzo (2020); Members (2020).
104 Patel (2020).
105 CDC (2022).
106 Bałazy (2006); Inglesby (2006).
107 Furukawa (2020).
108 Schweder (1982).
109 Altman (2011a); Altman (2011b).
110 Jefferson (2020).
111 Aggarwal (2020); Kim (2022); Nanda (2021); Ollila (2022); Tran (2021); Xiao (2020).
112 WHO (2019).
113 Liu (2021).
114 Jefferson (2020).
115 Aggarwal (2020).
116 Xiao (2020).
117 WHO (2019).
118 Executive Summary, in WHO (2019).
119 Nanda (2021).
120 Nanda (2021); WHO (2019); Xiao (2020).
121 Tran (2021).
122 Kim (2022).
123 Ollila (2022).
124 Liu (2021).
125 Young (2019b); Young (2021a); Young (2022a); Young (2022c); Young (2023a); Kindzierski (2021).
126 Young (2019b); Young (2021a); Young (2022a); Young (2022c); Young (2023a); Kindzierski (2021).
127 Liu (2021); Ollila (2022).
128 Aggarwal (2020); Tran (2021).
129 Nanda (2021); Xiao (2020).
130 Wang (2021).
131 Wang (2021).
132 Fennelly (2020); Wang (2021).
133 Kupferschmidt (2022).
134 Hardie (2016); Inglesby (2006).
135 Drummond (2022); Miller (2022).
136 UMD (2022).
137 Mask compliance data shown here are averaged from daily data representing the percent of Facebook respondents that reported wearing a mask most or all the time in the previous 5 days; data are from UMD (2022). See UMD COVID-19 Trends and Impact Survey (https://gisumd.github.io/COVID-19-API-Documentation/). Data are adjusted by Facebook for selection biases (non-response and sampling frame coverage bias).
138 Miller (2022).
139 World Health Organization COVID-19 dashboard (https://covid19.who.int/).
140 Daily death data are from the WHO COVID-19 dashboard (https://covid19.who.int/).
141 Schlette (2020).
142 Claeson (2021a); Claeson (2021b).
143 Schlette (2020); Claeson (2021a); Claeson (2021b).
144 European Union (2023).
145 World Population Review (2020).
146 AIER (2020); James (2020).
147 Axe (2020); Boyd (2020); Briggs (2020); Dayaratna (2020); IHME (2021); Magness (2020); Magness (2021a); Magness (2021b); Members (2020); Ridley (2020); Verity (2020).
148 Anderson (2021); Chamberlain (2021); Mandavilli (2020); Miller (2022); Mouzo (2022).
149 Axe (2020).
150 Offen (2005).
151 Cooper (2019).
152 Mousavi (2021).
153 Blanding (2021).
154 BNC (2021).
155 Terris (2011).
156 Hart (2022); Nelson (2022).
157 Vishwamitra (2021).
158 Gelman and Loken (2013).
159 Cf. Briggs (2016); especially the We Must Do Something Fallacy and the Epidemiologist Fallacy.
160 California (2020); CDC (2022); Honein (2020)
161 Drabiak (2021).
162 Lander (2022).
163 Ioannidis (2022g).
164 Ioannidis (2022d); Young (2021a).
165 Cox (2020).
166 Gelman (2019).
167 Briggs (2018); and see Briggs (2016).
168 Editorial Board (2021); Hart (2022); Kulldorff (2020); Mosher (2022); Nelson (2022); Shahbaz (2020); Wittkowski (2022).
169 Fischer (2016); Goldstein (2001); Martuzzi (2007).
170 Westfall (1993)
171 Friedman (1959).
172 Case (1985); Shekelle (1985a); Shekelle (1985b).
173 Heinonen (1974).
174 Curb (1982); Labarthe (1980).
175 Shapiro (2004).
176 Mayes (1988).
177 Feinstein (1988).
178 Westfall (1993); Mayo (2018).
179 E.g., Erikssen (1980); Garrison (1976).
180 Westfall (1985).
181 Rothman (1990).
182 Westfall (1993).
183 GS (2020a).
184 Genetic epidemiologists cite Westfall (1993) fairly frequently, but not epidemiologists in other subdisciplines. As of October 2020, Westfall (1993) has been cited twice in Environmental Health Perspectives, once in the American Journal of Epidemiology, once in the International Journal of Epidemiology, and never in the Annals of Epidemiology or Epidemiology.
185 Clyde (2000).
186 GS (2020b). The two citing articles are Moolgavkar (2013); Roberts (2010).
187 Hayat (2017).
188 NASEM (1991).
189 Altman (2011a); Altman (2011b).
190 Given the assumption that the null hypothesis is actually true, the p-value indicates the frequency with which the researcher, if he repeated his experiment by collecting new data, would expect to obtain data less compatible with the null hypothesis than the data he actually found. A p-value of 0.20, for example, means that if the researcher repeated his research over and over in a world where the null hypothesis is true, only 20% of his results would be less compatible with the null hypothesis than the results he actually got.
191 NASEM (2019); Randall (2018).
192 Briggs, Trafimow, and others reject the use of p-values for analyzing and interpreting data. Briggs (2016); Briggs (2019); Trafimow (2018); and see Berger (1987); Cohen (1994). They argue that null hypothesis significance testing, p-values, and the like are irredeemably flawed and that they should never be used in any way. We do not dispute this argument—but neither do we use it in this particular critique. As risk ratios and confidence intervals are common statistical measures in epidemiology, our use of p-values is in any case as a complementary measure of confidence intervals for p-value plotting. McCormack (2013); Montgomery (2003). We do generally recommend that epidemiologists address the critique by Briggs, et al.
193 Ritchie (2020); and see Joseph (2020).
194 Randall (2018).
195 Chambers (2017); Harris (2017); Hubbard (2015); Randall (2018); Ritchie (2020).
196 Fanelli (2009); John (2012); Randall (2018); Ritchie (2020); Schwarzkopf (2014); Simonsohn (2014).
197 Bruns (2016); Head (2015); but see Hartgerink (2017); Tanner (2015).
198 NASEM (2016); NASEM (2019); Nosek (2020); Pellizzari (2017).
199 Goodman (2016).
200 We define reproducibility throughout our report as the testing and reproducing of an experiment’s underlying hypothesis using fresh data and/or a new method of analysis. Psychologists also conduct conceptual replications, “the attempt to test the same theoretical process as an existing study, but that uses methods that vary in some way from the previous study” (Crandall 2016). The biomedical literature, however, does not refer to conceptual replication (NASEM 2016), and we have not innovated by using it in this report. We note the general importance and usefulness of conceptual replication, however, and we recommend that professionals in other disciplines consider whether it can be adapted usefully for their own research procedures.
201 Halsey (2015); Ioannidis (2005); Randall (2018).
202 Baker (2016).
203 Archer (2020); Chawla (2020); Coleman (2019); Engber (2017); Gobry (2016); Hennen (2019); Herold (2018); Ioannidis (2005); Manuel (2019); NASEM (2019); Randall (2018); Yong (2018); Young (2018); Zeeman (1976); Zimring (2019).
204 Merton (1973); and see Anderson (2010); Kim (2018).
205 Sample (2019).
206 Buchanan (2004); Edwards (2017); Freese (2018); Glaeser (2006); and see Keller (2015); Shapin (1994).
207 Buchanan (2004).
208 Cecil (1985); Feinstein (1988).
209 Ritchie (2020).
210 Martino (2017); Lilienfeld (2017).
211 Cordes (1998); Kaiser (2017); Roche (1994).
212 E.g., Oreskes (2010).
213 Young (2011).
214 Open Science Collaboration (2015).
215 Bidel (2013); Chambers (2017); Harris (2017); Hubbard (2015); MacMahon (1981).
216 Feinstein (1988b); Ogden (2011); Schachtman (2011); Schroter (2008); Smith (2010).
217 E.g., EPA (n.d.).
218 Taleb (2018).
219 Randall (2018); Ritchie (2020).
220 Allison (2016).
221 Olson (2002); Nissen (2016); Randall (2018).
222 Chambers (2017); Harris (2017); Hubbard (2015); Ritchie (2020).
223 We use RCTs in the remainder of this report to refer both to “randomized controlled trials” and to “randomized clinical trials”; both terms are common in the literature, and they are roughly equivalent.
224 Dickersin (1987).
225 Franco (2014).
226 Michaels (2008).
227 Kühberger (2014).
228 Gerber (2008).
229 McCambridge (2007).
230 Coronado-Montoya (2016).
231 And see Young (2022a).
232 Ioannidis (2011).
233 Westfall (1993).
234 Chambers (2017); Glaeser (2006); Harris (2017); Hubbard (2015); Ritchie (2020); Westfall (1993).
235 Westfall (1993).
236 Young (2009).
237 Mathews (2008).
238 Young (2019a); Young (2019b); Young (2019c).
239 Schweder and Spjøtvoll applied p-value plotting to evaluate many different questions. Schweder (1982). We apply p-value plotting to evaluate meta-analyses devoted to a single question; we believe our application of p-value plotting is original.
240 Fisher (1925); Fisher (1935); Mayo (2018).
241 An individual p-value that is extraordinarily small ( = far below 0.05), after adjustment for multiple testing, also has potential evidentiary value—but this occurs rarely in well-designed and well-executed epidemiology studies that control properly for bias and MTMM.
242 Young (2009). We acquired the data from the original researchers, who, to our knowledge, have not yet made it public. Interested scholars who wish to reproduce our analysis should contact the original researchers.
243 De Souto Barreto (2019).
244 Mathews (2009).
245 Lee (2012).
246 Li (2017).
247 Mayo (2018).
248 Mathews (2009).
249 Grossman (2005).
250 Carter (2019).
251 Chen (2013); Glass (1976); Stroup (2000).
252 De Vrieze (2018).
253 Ioannidis (2016).
254 Rothstein (2005); Thornton (2000).
255 Palpacuer (2019).
256 Cecil (1985); Wojick (2015).
257 Lorenc (2016).
258 Young (2019a).
259 Randall (2018); Ritchie (2020).
260 Ritchie (2020).
261 Jaeger (1998).
262 Bandholm (2017).
263 Young (2011); Young (2017).
264 Bandholm (2017).
265 Westfall (1993).
266 Ritchie (2020).
267 Wagenmakers (2012).
268 Nilsen (2020).
269 The terms ((covid[Title]) OR (sars-cov-2[Title])) AND (meta-analysis[Title]) [timeline 2020-2023] were used on December 7, 2022.
270 Bałazy (2006).
271 Bar-On (2020); Menter (2020); Zhu (2020).
272 Mosley (1946); NIH (2017); Stanley (1944).
273 Stott (1972).
274 Clase (2020); Meyerowitz (2021); Prather (2020); Tellier (2006); Tellier (2009); Wang (2021).
275 Wang (2021).
276 Han (2013); Wang (2021).
277 Fennelly (2020); Meyerowitz (2021).
278 Scheuch (2020).
279 Scheuch (2020).
280 Belkin (1996).
281 Belkin (1996).
282 Belkin (1996).
283 Wang (2021).
284 Inglesby (2006).
285 O’Conner (2008).
286 Jefferson (2020); Liu (2021).
287 Moffatt (2000a); Rabinowitz (2015); Smith-Sivertsen (2000).
288 Borlée (2019).
289 Moffatt (2000b).
290 Marcon (2015).
291 Michaud (2018).
292 We relaxed the CENTRAL search strategy in that it excluded targeted search terms such as mask, masks, facemasks, nonpharmaceutical, randomized, or randomised. Here, we anticipated that there would not be many listings in the CENTRAL database. We performed the search using the following terms: “influenza A” OR “influenza B” OR “covid” OR “sars-cov-2” OR “respiratory” in Title Abstract Keyword AND “infectious disease” Topic AND “01 January 2020 to 07 December 2022” Custom date range.
Due to the potentially large number of COVID-19 meta-analysis studies in the PubMed database, the search strategy differed, and it included more targeted terms. These terms included: (((((((influenza[Title]) OR (covid[Title])) OR (sars-cov-2[Title])) OR (respiratory[Title])) OR (viral transmission[Title])) AND ((((nonpharmaceutical[Title]) OR (mask[Title])) OR (masks[Title])) OR (facemasks[Title]))) AND ((randomized[Title/Abstract]) OR (randomised[Title/Abstract]))) AND (("2020/01/01"[Date - Entry] : "2022/12/07"[Date - Entry])).
293 Jefferson (2020).
294 Aggarwal (2020).
295 Xiao (2020).
296 Nanda (2021).
297 Tran (2021).
298 Kim (2022).
299 Ollila (2022).
300 Carp (2012); Contopoulos-Ioannidis (2009); Ioannidis (2008); Ioannidis (2011); Kavvoura (2007).
301 Kavvoura (2007).
302 Abaluck (2022); Aiello (2010a); Barasheed (2014).
303 Barasheed (2014).
304 Aiello (2010b).
305 Chikina (2022).
306 Chikina (2022).
307 Abaluck (2022).
308 Liu (2021).