Preface by Peter Wood
The study you have before you is an examination of the use and abuse of statistics in the sciences. Its natural audience is members of the scientific community who use statistics in their professional research. We hope, however, to reach a broader audience of intelligent readers who recognize the importance to our society of maintaining integrity in the sciences.
Statistics, of course, is not an inviting topic for most people. If we had set out with the purpose of finding a topic less likely to attract broad public attention, a study of statistical methods might well have been the first choice. It would have come in ahead of a treatise on trilobites or a rumination on rust. I know that because I have before me popular books on trilobites and rust: copies of Riccardo Levi-Setti’s Trilobites and Jonathan Waldman’s Rust: The Longest War on my bookshelf. Both books are, in fact, fascinating for the non-specialist reader.
Efforts to interest general readers in statistics are not rare, though it is hard to think of many successful examples. Perhaps the most successful was Darrell Huff’s 1954 semi-classic, How to Lie with Statistics, which is still in print and has sold more than 1.5 million copies in English. That success was not entirely due to a desire on the part of readers to sharpen their mendacity. Huff’s short introduction to common statistical errors became a widely assigned textbook in introductory statistics courses.
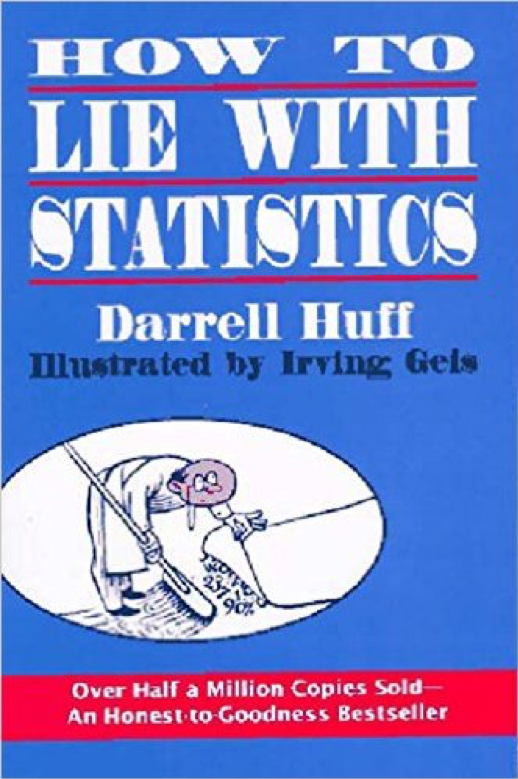
The challenge for the National Association of Scholars in putting together this report was to address in a serious way the audience of statistically literate scientists while also reaching out to readers who might quail at the mention of p-values and the appearance of sentences which include symbolic statements such as defining “statistical significance as p < .01 rather than as p < .05.”
This preface is intended mainly for those general readers. It explains why the topic is important and it includes no further mention of p-values.
Disinterested Inquiry and Its Opponents
The National Association of Scholars (NAS) has long been interested in the politicization of science. We have also long been interested in the search for truth—but mainly as it pertains to the humanities and social sciences. The irreproducibility crisis brings together our two long-time interests, because the inability of science to discern truth properly and its politicization go hand in hand.
The NAS was founded in 1987 to defend the vigorous liberal arts tradition of disciplined intellectual inquiry. The need for such a defense had become increasingly apparent in the previous decade and is benchmarked by the publication of Allan Bloom’s The Closing of the American Mind in January 1987. The founding of the NAS and the publication of Bloom’s book were coincident but unrelated except that both were responses to a deep shift in the temperament of American higher education. An older ideal of disinterested pursuit of truth was giving way to views that there was no such thing. All academic inquiry, according to this new view, served someone’s political interests, and “truth” itself had to be counted as a questionable concept.
The new, alternative view, was that college and universities should be places where fresh ideas untrammeled by hidden connections to the established structures of power in American society should have the chance to develop themselves. In practice this meant a hearty welcome to neo- Marxism, radical feminism, historicism, post-colonialism, deconstructionism, post-modernism, liberation theology, and a host of other ideologies. The common feature of these ideologies was their comprehensive hostility to the core traditions of the academy. Some of these doctrines have now faded from the scene, but the basic message—out with disinterested inquiry, in with leftist political nostrums—took hold and has become higher education’s new orthodoxy.
To some extent the natural sciences held themselves exempt from the epistemological and social revolution that was tearing the humanities (and the social sciences) apart. Most academic scientists believed that their disciplines were immune from the idea that facts are “socially constructed.” Physicists were disinclined to credit the claim that there could be a feminist, black, or gay physics. Astronomers were not enthusiastic about the concept that observation is inevitably a reflex of the power of the socially privileged.
The Pre-History of This Report
The report’s authors, David Randall and Christopher Welser, are gentle about the intertwining of the irreproducibility crisis, politicized groupthink among scientists, and advocacy-driven science. But the NAS wishes to emphasize how important the tie is between the purely scientific irreproducibility crisis and its political effects. Sloppy procedures don’t just allow for sloppy science. They allow, as opportunistic infections, politicized groupthink and advocacy-driven science. Above all, they allow for progressive skews and inhibitions on scientific research, especially in ideologically driven fields such as climate science, radiation biology, and social psychology (marriage law). Not all irreproducible research is progressive advocacy; not all progressive advocacy is irreproducible; but the intersection between the two is very large. The intersection between the two is a map of much that is wrong with modern science.
When the progressive left’s “long march through the university” began, the natural sciences believed they would be exempt, but the complacency of the scientific community was not total. Some scientists had already run into obstacles arising from the politicization of higher education. And soon after its founding, the NAS was drawn into this emerging debate. In the second issue of NAS’s journal, Academic Questions, published in Spring 1988, NAS ran two articles criticizing a report by the American Physical Society, that took strong exception to the quality of science in that report. One of the articles, written by Frederick Seitz, who was the former president of both the American Physical Society and the National Academy of Sciences, accused the Council of the American Physical Society of issuing a statement based on the report that abandoned “all pretense to being based on scientific factors.” The report and the advocacy based on it (dealing with missile defense) were, in Seitz’s view, “political” in nature.
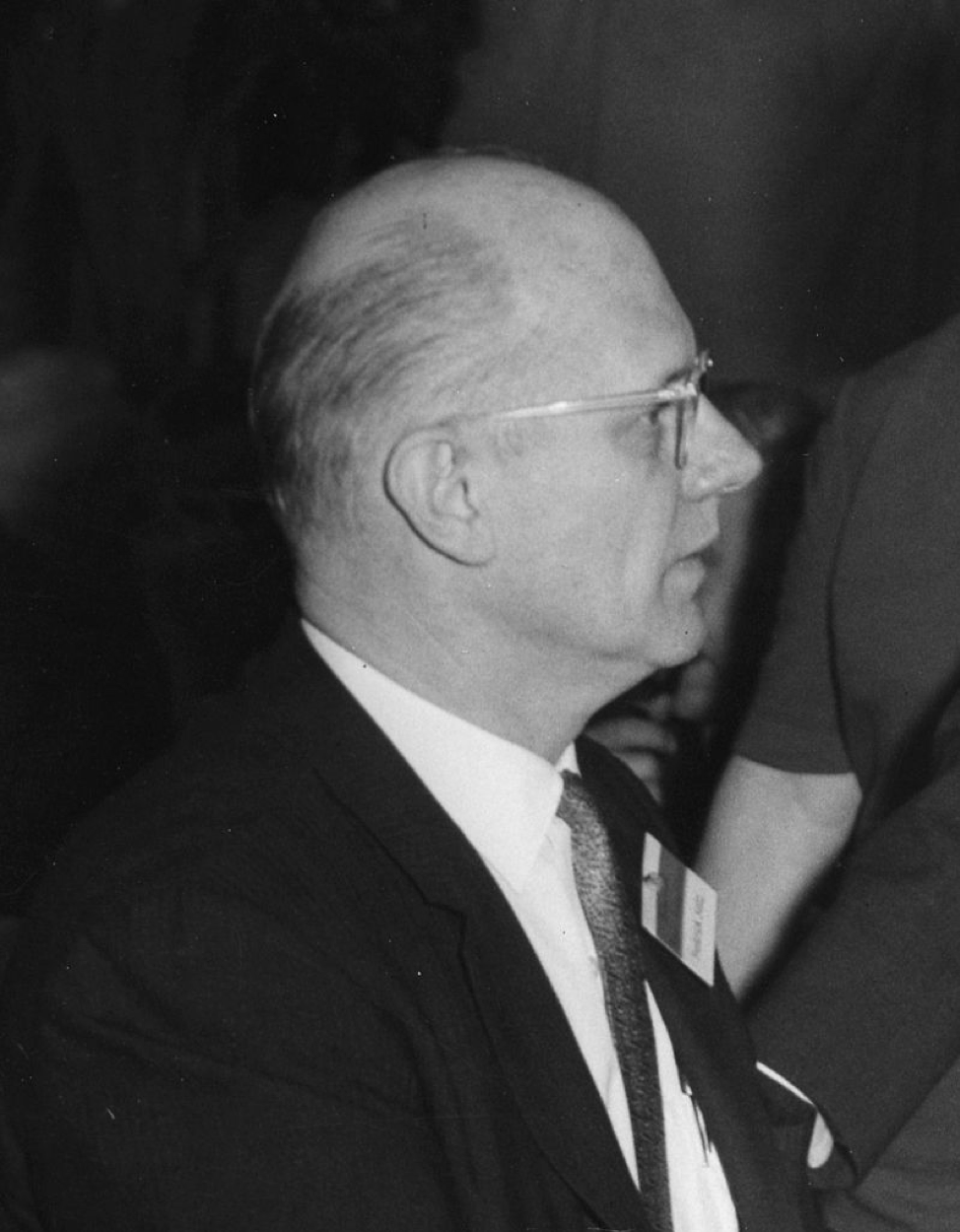
I cite this long-ago incident as part of the pedigree of this report, The Irreproducibility Crisis. In the years following the Seitz article, NAS took up a great variety of “academic questions.” The integrity of the sciences was seldom treated as among the most pressing matters, but it was regularly examined, and NAS’s apprehensions about misdirection in the sciences were growing. In 1992, Paul Gross contributed a keynote article, “On the Gendering of Science.” In 1993, Irving M. Klotz wrote on “‘Misconduct’ in Science,” taking issue with what he saw as an overly expansive definition of misconduct promoted by the National Academy of Sciences. Paul Gross and Norman Levitt presented a broader set of concerns in 1994, in “The Natural Sciences: Trouble Ahead? Yes.” Later that year, Albert S. Braverman and Brian Anziska wrote on “Challenges to Science and Authority in Contemporary Medical Education.” That same year NAS held a national conference on the state of the sciences. In 1995, NAS published a symposium based on the conference, “What Do the Natural Sciences Know and How Do They Know It?”
For more than a decade NAS published a newsletter on the politicization of the sciences, and we have continued a small stream of articles on the topic, such as “Could Science Leave the University?” (2011) and “Short-Circuiting Peer-Review in Climate Science” (2014). When the American Association of University Professors published a brief report assailing the Trump administration as “anti-science,” (“National Security, the Assault on Science, and Academic Freedom,” December 2017), NAS responded with a three-part series, “Does Trump Threaten Science?” (To be clear, we are a non-partisan organization, interested in promoting open inquiry, not in advancing any political agenda.)
The Irreproducibility Crisis builds on this history of concern over the threats to scientific integrity, but it is also a departure. In this case, we are calling out a particular class of errors in contemporary science. Those errors are sometimes connected to the politicization of the sciences and scientific misconduct, but sometimes not. The reforms we call for would make for better science in the sense of limiting needless errors, but those reforms would also narrow the opportunities for sloppy political advocacy and damaging government edicts.
Threat Assessment
Over the thirty-one year span of NAS’s work, we have noted both the triumphs of contemporary science—and they are many—but also rising threats. Some of these threats are political or ideological. Some are, for lack of a better word, epistemic. The former include efforts to enforce an artificial “consensus” on various fields of inquiry, such as climate science. The ideological threats also include the growing insistence that academic positions in the sciences be filled with candidates chosen partly on the basis of race and sex. These ideological impositions, however, are not the topic of The Irreproducibility Crisis.
This report deals with an epistemic problem, which is most visible in the large numbers of articles in reputable peer-reviewed journals in the sciences that have turned out to be invalid or highly questionable. Findings from experimental work or observational studies turn out, time and again, to be irreproducible. The high rates of irreproducibility are an ongoing scandal that rightly has upset a large portion of the scientific community. Estimates of what percentage of published articles present irreproducible results vary by discipline. Randall and Welser cite various studies, some of them truly alarming. A 2012 study, for example, aimed at reproducing the results of 53 landmark studies in hematology and oncology, but succeeded in replicating only six (11 percent) of those studies.
Irreproducibility can stem from several causes, chief among them fraud and incompetence. The two are not always easily distinguished, but The Irreproducibility Crisis deals mainly with the kinds of incompetence that mar the analysis of data and that lead to insupportable conclusions. Fraud, however, is also a factor to be weighed.
Outright Fraud
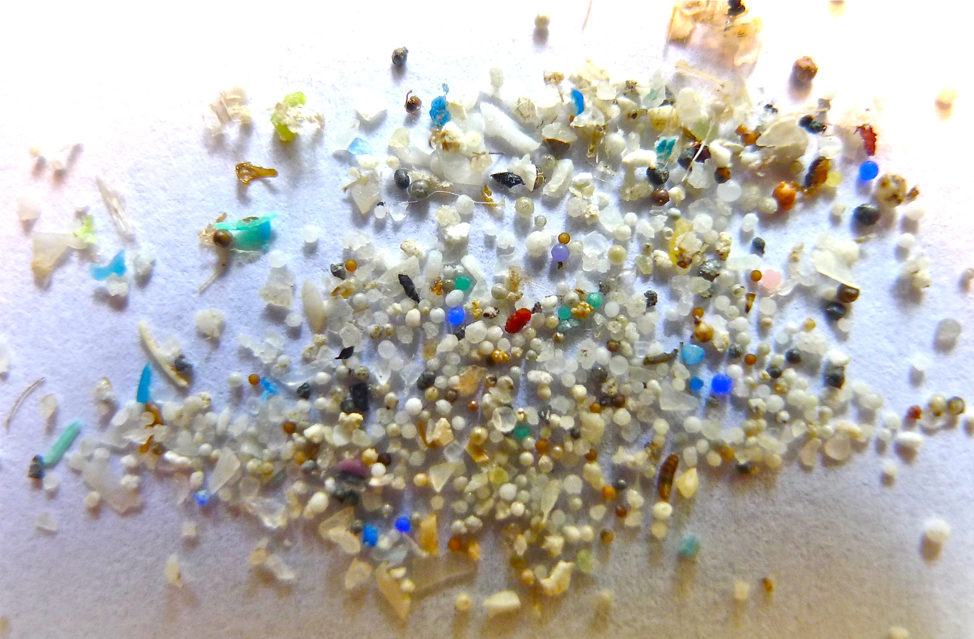
Actual fraud on the part of researchers appears to be a growing problem. Why do scientists take the risk of making things up when, over the long term, it is almost certain that the fraud will be detected? No doubt in some cases the researchers are engaged in wishful thinking. Even if their research does not support their hypothesis, they imagine the hypothesis will eventually be vindicated, and publishing a fictitious claim now will help sustain the research long enough to vindicate the original idea. Perhaps that is what happened in the recent notorious case of postdoc Oona Lönnstedt at Uppsala University. She and her supervisor, Peter Eklöv, published a paper in Science in June 2016, warning of the dangers of microplastic particles in the ocean. The microplastics, they reported, endangered fish. It turns out that Lönnstedt never performed the research that she and Eklöv reported.
The initial June 2016 article achieved worldwide attention and was heralded as the revelation of a previously unrecognized environmental catastrophe. When doubts about the research integrity began to emerge, Uppsala University investigated and found no evidence of misconduct. Critics kept pressing and the University responded with a second investigation that concluded in April 2017 and found both Lönnstedt and Eklöv guilty of misconduct. The university then appointed a new Board for Investigation of Misconduct in Research. In December 2017 the Board announced its findings: Lönnstedt had intentionally fabricated her data and Eklöv had failed to check that she had actually carried out her research as described.
The microplastics case illustrates intentional scientific fraud. Lönnstedt’s motivations remain unknown, but the supposed findings reported in the Science article plainly turned her into an environmentalist celebrity. Fear of the supposedly dire consequences of microplastic pollution had already led to the U.S. banning plastic microbeads in personal care products. The UK was holding a parliamentary hearing on the same topic when the Science article appeared. Microplastic pollution was becoming a popular cause despite thin evidence that the particles were dangerous. Lönnstedt’s contribution was to supply the evidence.
In this case, the fraud was suspected early on and the whistleblowers stuck with their accusations long enough to get past the early dismissals of their concerns. That kind of self-correction in the sciences is highly welcome but hardly reliable. Sometimes highly questionable declarations made in the name of science remain un-retracted and ostensibly unrefuted despite strong evidence against them. For example, Edward Calabrese in the Winter 2017 issue of Academic Questions recounts the knowing deception by Nobel physicist Hermann J. Muller, who promoted what is called the “linear no-threshold” (LNT) dose response model for radiation’s harmful effects. That meant, in layman’s terms, that radiation at any level is dangerous. Muller had seen convincing evidence that the LNT model was false—that there are indeed thresholds below which radiation is not dangerous—but he used his 1946 Nobel Prize Lecture to insist that the LNT model be adopted. Calabrese writes that Muller was “deliberately deceptive.”
It was a consequential deception. In 1956 the National Academy of Sciences Committees on Biological Effects of Atomic Radiation (BEAR) recommended that the U.S. adopt the LNT standard. BEAR, like Muller, misrepresented the research record, apparently on the grounds that the public needed a simple heuristic and the actual, more complicated reality would only confuse people. The U.S. Government adopted the LNT standard in evaluating risks from radiation and other hazards. Calabrese and others who have pointed out the scientific fraud on which this regulatory apparatus rests have been brushed aside and the journal Science, which published the BEAR report, has declined to review that decision.
Which is to say that if a deception goes deep enough or lasts long enough, the scientific establishment may simply let it lie. The more this happens, presumably the more it emboldens other researchers to gamble that they may also get away with making up data or ignoring contradictory evidence.
Renovation
Incompetence and fraud together create a borderland of confusion in the sciences. Articles in prestigious journals appear to speak with authority on matters that only a small number of readers can assess critically. Non-specialists generally are left to trust that what purports to be a contribution to human knowledge has been scrutinized by capable people and found trustworthy. Only we now know that a very significant percentage of such reports are not to be trusted. What passes as “knowledge” is in fact fiction. And the existence of so many fictions in the guise of science gives further fuel to those who seek to politicize the sciences. The Lönnstedt and Muller cases exemplify not just scientific fraud, but also efforts to advance political agendas. All of the forms of intellectual decline in the sciences thus tend to converge. The politicization of science lowers standards, and lower standards invite further politicization.
The NAS wants to foster among scientists the old ethic of seeking out truth by sticking with procedures that rigorously sift and winnow what scientific experiment can say confidently from what it cannot. We want science to seek out truth rather than to engage in politicized advocacy. We want science to do this as the rule and not as the exception. This is why we call for these systemic reforms.
The NAS also wants to banish the calumny of progressive advocates, that anyone who criticizes their political agenda is ‘anti-science.’ This was always hollow rhetoric, but the irreproducibility crisis reveals that it is precisely the reverse of the situation. The progressive advocates, deeply invested in the sloppy procedures, the politicized groupthink, and the too-frequent outright fraud, are the anti-science party. The banner of good science—disinterested, seeking the truth, reproducible—is ours, not theirs.
We are willing to put this contention to the experiment. We call for all scientists to submit their science to the new standards of reproducibility—and we will gladly see what truths we learn and what falsehoods we will unlearn.
For all that, The Irreproducibility Crisis deals with only part of a larger problem. Scientists are only human and are prey to the same temptations as anyone else. To the extent that American higher education has become dominated by ideologies that scoff at traditional ethical boundaries and promote an aggressive win-at-all-costs mentality, reforming the technical and analytic side of science will go only so far towards restoring the integrity of scientific inquiry. We need a more comprehensive reform of the university that will instill in students a lifelong fidelity to the truth. This report, therefore, is just one step towards the necessary renovation of American higher education. The credibility of the natural sciences is eroding. Let’s stop that erosion and then see whether the sciences can, in turn, teach the rest of the university how to extract itself from the quicksand of political advocacy.
Executive Summary
The Nature of the Crisis
A reproducibility crisis afflicts a wide range of scientific and social-scientific disciplines, from epidemiology to social psychology. Improper research techniques, lack of accountability, disciplinary and political groupthink, and a scientific culture biased toward producing positive results together have produced a critical state of affairs. Many supposedly scientific results cannot be reproduced reliably in subsequent investigations, and offer no trustworthy insight into the way the world works.
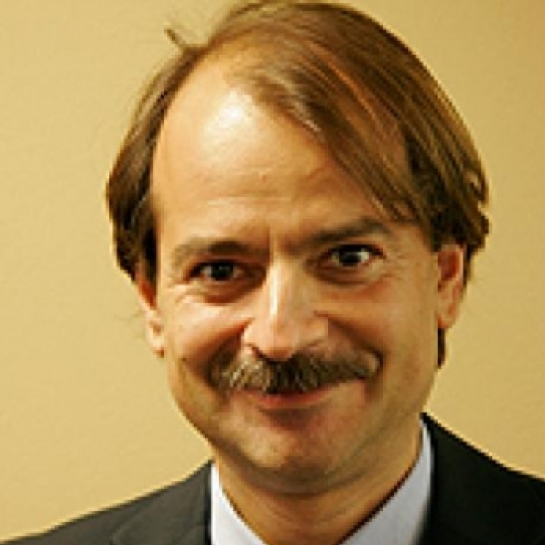
In 2005, Dr. John Ioannidis argued, shockingly and persuasively, that most published research findings in his own field of medicine were false. Contributing factors included 1) the inherent limitations of statistical tests; 2) the use of small sample sizes; 3) reliance on small numbers of studies; 4) willingness to publish studies reporting small effects; 5) the prevalence of fishing expeditions to generate new hypotheses or explore unlikely correlations; 6) flexibility in research design; 7) intellectual prejudices and conflicts of interest; and 8) competition among researchers to produce positive results, especially in fashionable areas of research. Ioannidis demonstrated that when you accounted for all these factors, a majority of research findings in medicine—and in many other scientific fields—were probably wrong.
Ioannidis’ alarming article crystallized the scientific community’s awareness of the reproducibility crisis. Subsequent evidence confirmed that the crisis of reproducibility had compromised entire disciplines. In 2012 the biotechnology firm Amgen tried to reproduce 53 “landmark” studies in hematology and oncology, but could only replicate six. In that same year the director of the Center for Drug Evaluation and Research at the Food and Drug Administration estimated that up to three-quarters of published biomarker associations could not be replicated. A 2015 article in Science that presented the results of 100 replication studies of articles published in prominent psychological journals found that only 36% of the replication studies produced statistically significant results, compared with 97% of the original studies.
Many common forms of improper scientific practice contribute to the crisis of reproducibility. Some researchers look for correlations until they find a spurious “statistically significant” relationship. Many more have a poor understanding of statistical methodology, and thus routinely employ statistics improperly in their research. Researchers may consciously or unconsciously bias their data to produce desired outcomes, or combine data sets in such a way as to invalidate their conclusions. Researchers able to choose between multiple measures of a variable often decide to use the one which provides a statistically significant result. Apparently legitimate procedures all too easily drift across a fuzzy line into illegitimate manipulations of research techniques.
Many aspects of the professional environment in which researchers work enable these distortions of the scientific method. Uncontrolled researcher freedom makes it easy for researchers to err in all the ways described above. The fewer the constraints on their research designs, the more opportunities for them to go astray. Lack of constraints allows researchers to alter their methods midway through a study as they pursue publishable, statistically significant results. Researchers often justify midstream alteration of research procedures as “flexibility,” but in practice such flexibility frequently justifies researchers’ unwillingness to accept a negative outcome. A 2011 article estimated that providing four “degrees of researcher freedom”—four ways to shift the design of the experiment while it is in progress—can lead to a 61% false-positive rate.
The absence of openness in much scientific research poses a related problem. Researchers far too rarely share data and methodology once they complete their studies. Scientists ought to be able to check and critique one another’s work, but a great deal of research can’t be evaluated properly because researchers don’t always make their data and study protocols available to the public. Sometimes unreleased data sets simply vanish because computer files are lost or corrupted, or because no provision is made to transfer data to up-to-date systems. In these cases, other researchers lose the ability to examine the data and verify that it has been handled correctly.
Another factor contributing to the reproducibility crisis is the premium on positive results. Modern science’s professional culture prizes positive results far above negative results, and also far above attempts to reproduce earlier research. Scientists therefore steer away from replication studies, and their negative results go into the file drawer. Recent studies provide evidence that this phenomenon afflicts such diverse fields as climate science, psychology, sociology, and even dentistry.
Groupthink also inhibits attempts to check results, since replication studies can undermine comfortable beliefs. An entire academic discipline can succumb to groupthink and create a professional consensus with a strong tendency to dismiss results that question its foundations. The overwhelming political homogeneity of academics has also created a culture of groupthink that distorts academic research, since researchers may readily accept results that confirm a liberal world-view while rejecting “conservative” conclusions out of hand. Political groupthink particularly affects those fields with obvious policy implications, such as social psychology and climate science.
Just the financial consequences of the reproducibility crisis are enormous. A 2015 study estimated that researchers spent around $28 billion annually in the United States alone on irreproducible preclinical research into new drug treatments. Irreproducible research in several disciplines distorts public policy and public expenditure in areas such as public health, climate science, and marriage and family law. The gravest casualty of all is the authority that science ought to have with the public, but which it has been forfeiting through its embrace of practices that no longer serve to produce reliable knowledge.
Many researchers and interested laymen have already started to improve the practice of science. Scientists, journals, foundations, and the government have all taken concrete steps to alleviate the crisis of reproducibility. But there is still much more to do. The institutions of modern science are enormous, not all scientists accept the nature and extent of the crisis, and the public has scarcely begun to realize the crisis’s gravity. Fixing the crisis of reproducibility will require a great deal of work. A long-term solution will need to address the crisis at every level: technical competence, institutional practices, and professional culture.
The National Association of Scholars proposes the following list of 40 specific reforms that address all levels of the reproducibility crisis. These suggested reforms are not comprehensive—although we believe they are more comprehensive than any previous set of recommendations. Some of these reforms have been proposed before; others are new. Some will elicit broad assent from the scientific community; we expect others to arouse fierce disagreement. Some are meant to provoke constructive critique.
We do not expect every detail of these proposed reforms to be adopted. Yet we believe that any successful reform program must be at least as ambitious as what we present here. If not these changes, then what? We proffer this program of reform to spark an urgently needed national conversation on how precisely to solve the crisis of reproducibility.
STATISTICAL STANDARDS
- Researchers should avoid regarding the p-value as a dispositive measure of evidence for or against a particular research hypothesis.
- Researchers should adopt the best existing practice of the most rigorous sciences and define statistical significance as p < .01 rather than as p < .05.
- In reporting their results, researchers should consider replacing either-or tests of statistical significance with confidence intervals that provide a range in which a variable’s true value most likely falls.
DATA HANDLING
- Researchers should make their data available for public inspection after publication of their results.
- Researchers should experiment with born-open data—data archived in an open-access repository at the moment of its creation, and automatically time-stamped.
RESEARCH PRACTICES
- Researchers should pre-register their research protocols, filing them in advance with an appropriate scientific journal, professional organization, or government agency.
- Researchers should adopt standardized descriptions of research materials and procedures.
PEDAGOGY
- Disciplines that rely heavily upon statistics should institute rigorous programs of education that emphasize the ways researchers can misunderstand and misuse statistical concepts and techniques.
- Disciplines that rely heavily upon statistics should educate researchers in the insights provided by Bayesian approaches.
- Basic statistics should be integrated into high school and college math and science curricula, and should emphasize the limits to the certainty that statistics can provide.
UNIVERSITY POLICIES
- Universities judging applications for tenure and promotion should require adherence to best-existing-practice standards for research techniques.
- Universities should integrate survey-level statistics courses into their core curricula and distribution requirements.
PROFESSIONAL ASSOCIATIONS
- Each discipline should institutionalize regular evaluations of its intellectual openness by committees of extradisciplinary professionals.
PROFESSIONAL JOURNALS
- Professional journals should make their peer review processes transparent to outside examination.
- Some professional journals should experiment with guaranteeing publication for research with pre-registered, peer-reviewed hypotheses and procedures.
- Every discipline should establish a professional journal devoted to publishing negative results.
SCIENTIFIC INDUSTRY
- Scientific industry should advocate for practices that minimize irreproducible research, such as Transparency and Openness Promotion (TOP) guidelines for scientific journals.
- Scientific industry, in conjunction with its academic partners, should formulate standard research protocols that will promote reproducible research.
PRIVATE PHILANTHROPY
- Private philanthropy should fund scientists’ efforts to replicate earlier research.
- Private philanthropy should fund scientists who work to develop better research methods.
- Private philanthropy should fund university chairs in “reproducibility studies.”
- Private philanthropy should establish an annual prize, the Michelson-Morley Award, for the most significant negative results in various scientific fields.
- Private philanthropy should improve science journalism by funding continuing education for journalists about the scientific background to the reproducibility crisis.
GOVERNMENT FUNDING
- Government agencies should fund scientists’ efforts to replicate earlier research.
- Government agencies should fund scientists who work to develop better research methods.
- Government agencies should prioritize grant funding for researchers who pre-register their research protocols and who make their data and research protocols publicly available.
- Government granting agencies should immediately adopt the National Institutes of Health (NIH) standards for funding reproducible research.
- Government granting agencies should provide funding for programs to broaden statistical literacy in primary, secondary, and post-secondary education.
GOVERNMENT REGULATION
- Government agencies should insist that all new regulations requiring scientific justification rely solely on research that meets strict reproducibility standards.
- Government agencies should institute review commissions to determine which existing regulations are based on reproducible research, and to rescind those which are not.
FEDERAL LEGISLATION
- Congress should pass an expanded Secret Science Reform Act to prevent government agencies from making regulations based on irreproducible research.
- Congress should require government agencies to adopt strict reproducibility standards by measures that include strengthening the Information Quality Act.
- Congress should provide funding for programs to broaden statistical literacy in primary, secondary, and post-secondary education.
STATE LEGISLATION
- State legislatures should reform K-12 curricula to include courses in statistics literacy.
- State legislatures should use their funding and oversight powers to encourage public university administrations to add statistical literacy requirements.
GOVERNMENT STAFFING
- Presidents, governors, legislative committees, and individual legislators should employ staff trained in statistics and reproducible research techniques to advise them on scientific issues.
JUDICIARY REFORMS
- Federal and state courts should adopt a standard approach, which explicitly accounts for the crisis of reproducibility, for the use of science and social science in judicial decision-making.
- Federal and state courts should adopt a standard approach to overturning precedents based on irreproducible science and social science.
- A commission of judges should recommend that law schools institute a required course on science and statistics as they pertain to the law.
- A commission of judges should recommend that each state incorporate a science and statistics course into its continuing legal education requirements for attorneys and judges.
Introduction
Brian Wansink’s Disastrous Blog Post
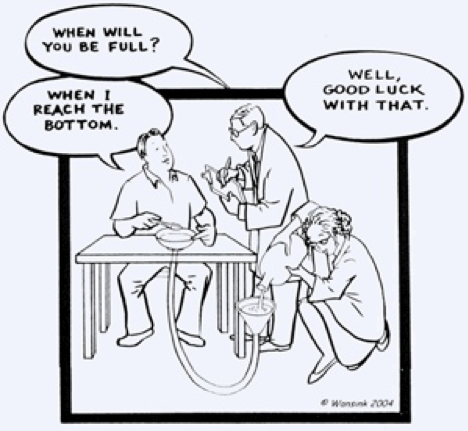
In November 2016, Brian Wansink got himself into trouble.1 Wansink, the head of Cornell University’s Food and Brand Lab and a professor at the Cornell School of Business, has spent more than twenty-five years studying “eating behavior”—the social and psychological factors that affect how people eat. He’s become famous for his research on the psychology of “mindless eating.” Wansink argues that science shows we’ll eat less on smaller dinner plates,2 and pour more liquid into short, wide glasses than tall, narrow ones.3 In August 2016 he appeared on ABC News to claim that people eat less when they’re told they’ve been served a double portion.4 In March 2017, he came onto Rachael Ray’s show to tell the audience that repainting your kitchen in a different color might help you lose weight.5
But Wansink garnered a different kind of fame when, giving advice to Ph.D. candidates on his Healthier and Happier blog, he described how he’d gotten a new graduate student researching food psychology to be more productive:
When she [the graduate student] arrived, I gave her a data set of a self-funded, failed study which had null results (it was a one month study in an all-you-can-eat Italian restaurant buffet where we had charged some people ½ as much as others). I said, “This cost us a lot of time and our own money to collect. There’s got to be something here we can salvage because it’s a cool (rich & unique) data set.” I had three ideas for potential Plan B, C, & D directions (since Plan A [the one-month study with null results] had failed). I told her what the analyses should be and what the tables should look like. I then asked her if she wanted to do them. … Six months after arriving, … [she] had one paper accepted, two papers with revision requests, and two others that were submitted (and were eventually accepted).6
Over the next several weeks, Wansink’s post prompted outrage among the community of internet readers who care strongly about statistics and the scientific method.7 “This is a great piece that perfectly sums up the perverse incentives that create bad science,” wrote one.8 “I sincerely hope this is satire because otherwise it is disturbing,” wrote another.9 “I have always been a big fan of your research,” wrote a third, “and reading this blog post was like a major punch in the gut.”10 And the controversy didn’t die down. As the months passed, the little storm around this apparently innocuous blog post kicked up bigger and bigger waves.
But what had Wansink done wrong? In essence, his critics accused him of abusing statistical procedures to create the illusion of successful research. And thereby hangs a cautionary tale—not just about Brian Wansink, but about the vast crisis of reproducibility in all of modern science.
The words reproducibility and replicability are often used interchangeably, as in this essay. When they are distinguished, replicability most commonly refers to whether an experiment’s results can be obtained in an independent study, by a different investigator with different data, while reproducibility refers to whether different investigators can use the same data, methods, and/or computer code to come up with the same conclusion.11 Goodman, Fanelli, and Ioannidis suggested in 2016 that scientists should not only adopt a standardized vocabulary to refer to these concepts but also further distinguish between methods reproducibility, results reproducibility, and inferential reproducibility.12 We use the phrase “crisis of reproducibility” to refer without distinction to our current predicament, where much published research cannot be replicated or reproduced.
The crisis of reproducibility isn’t just about statistics—but to understand how modern science has gone wrong, you have to understand how scientists use, and misuse, statistical methods.
How Researchers Use Statistics
Much of modern scientific and social-scientific research seeks to identify relationships between different variables that seem as if they ought to be linked. Researchers may want to know, for example, whether more time in school correlates with higher levels of income,13 whether increased carbohydrate intake tends to be associated with a greater risk of heart disease,14 or whether scores for various personality dimensions on psychometric tests help predict voting behavior.15
But it isn’t always easy for scientists to establish the existence of such relationships. The world is complicated, and even a real relationship—one that holds true for an entire population—may be difficult to observe. Schooling may generally have a positive effect on income, but some Ph.D.s will still work as baristas and some high school dropouts will become wealthy entrepreneurs. High carbohydrate intake may increase the risk of heart disease on average, but some paleo-dieters will drop dead of heart attacks at forty and some junk food addicts will live past ninety on a regime of doughnuts and French fries. Researchers want to look beneath reality’s messy surface and determine whether the relationships they’re interested in will hold true in general.
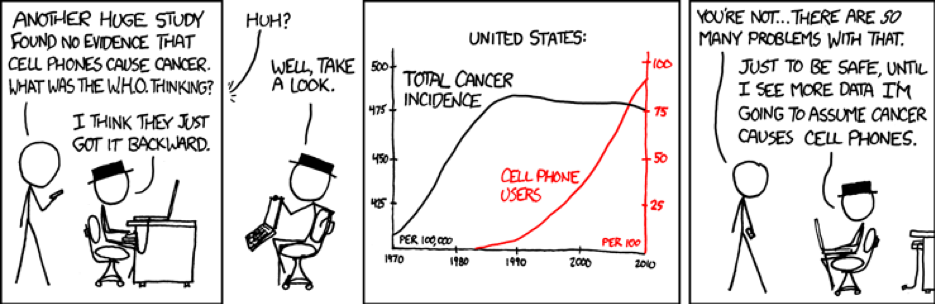
In dozens of disciplines ranging from epidemiology16 to environmental science17 to psychology18 to sociology,19 researchers try to do this by gathering data and applying hypothesis tests, also called tests of statistical significance. Many such tests exist, and researchers are expected to select the test that is most appropriate given both the relationship they wish to investigate and the data they have managed to collect.
In practice, the hypothesis that forms the basis of a test of statistical significance is rarely the researcher’s original hypothesis that a relationship between two variables exists. Instead, scientists almost always test the hypothesis that no relationship exists between the relevant variables. Statisticians call this the null hypothesis. As a basis for statistical tests, the null hypothesis is usually much more convenient than the researcher’s original hypothesis because it is mathematically precise in a way that the original hypothesis typically is not. Each test of statistical significance yields a mathematical estimate of how well the data collected by the researcher supports the null hypothesis. This estimate is called a p-value.
The p-value is a number between zero and one, representing a probability based on the assumption that the null hypothesis is actually true. Given that assumption, the p-value indicates the frequency with which the researcher, if he repeated his experiment by collecting new data, would expect to obtain data less compatible with the null hypothesis than the data he actually found. A p-value of .2, for example, means that if the researcher repeated his research over and over in a world where the null hypothesis is true, only 20% of his results would be less compatible with the null hypothesis than the results he actually got.
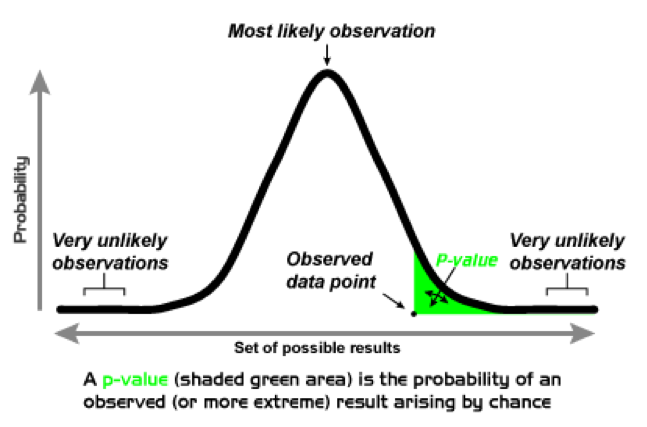
A very low p-value means that, if the null hypothesis is true, the researcher’s data are rather extreme. It should be rare for data to be so incompatible with the null hypothesis. But perhaps the null hypothesis is not true, in which case the researcher’s data would not be so surprising. If nothing is wrong with the researcher’s procedures for data collection and analysis, then the lower the p-value, the less likely it becomes that the null hypothesis is correct.
In other words: the lower the p-value, the more reasonable it is to reject the null hypothesis, and conclude that the relationship originally hypothesized by the researcher does exist between the variables in question. Conversely, the higher the p-value, and the more typical the researcher’s data would be in a world where the null hypothesis is true, the less reasonable it is to reject the null hypothesis. Thus, the p-value provides a rough measure of the validity of the null hypothesis—and, by extension, of the researcher’s “real hypothesis” as well.
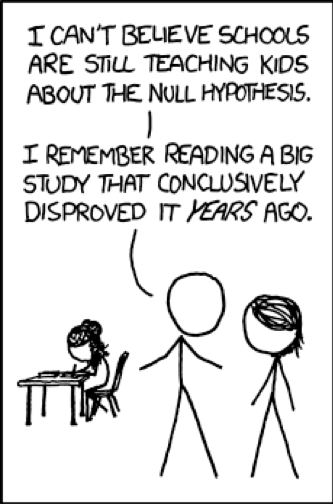
Say a scientist gathers data on schooling and income and discovers that in his sample each additional year of schooling corresponds, on average, to an extra $750 of annual income. The scientist applies the appropriate statistical test to the data, where the null hypothesis is that there is no relation between years of schooling and subsequent income, and obtains a p-value of .55. This means that more than half the time he would expect to see a correspondence at least as strong as this one even if there were no underlying relationship between time in school and income. A p-value of .01, on the other hand, would indicate a much greater probability that some relationship of the sort the scientist originally hypothesized actually exists. If there is no truth in the original hypothesis, and the null hypothesis is true instead, the sort of correspondence the scientist observed should occur only a small fraction of the time.
p < .05
Scientists can interpret results like these fairly easily: p = .55 means a researcher hasn’t found good evidence to support his original hypothesis, while p = .01 means the data seems to provide his original hypothesis with strong support. But what about p-values in between? What about p = .1, a 10% probability of data even less supportive of the null hypothesis occurring just by chance, without an underlying relationship?
Over time, researchers in various disciplines decided to adopt clear cutoffs that would separate strong evidence against the null hypothesis from weaker evidence against the null hypothesis. The idea was to ensure that the results of statistical tests weren’t used too loosely, in support of unsubstantiated conclusions. Different disciplines settled on different cutoffs: some adopted p < .1, some p < .05, and the most rigorous adopted p < .01. Nowadays, p < .05 is the most common cutoff. Scientists in most disciplines call results that meet that criterion “statistically significant.” p < .05 provides a pretty rigorous standard, which should ensure that researchers will incorrectly reject the null hypothesis—incorrectly infer that they have found evidence for their original hypothesis—no more than 5% of the time.
But no more than 5% of the time is still some of the time. A scientist who runs enough statistical tests can expect to get “statistically significant” results one time in twenty just by chance alone. And if a researcher produces a statistically significant result—if it meets that rigorous p < .05 standard established by professional consensus—it’s far too easy to present that result as publishable, even if it’s just a fluke, an artifact of the sheer number of statistical tests the researcher has applied to his data.
A strip from Randall Munroe’s webcomic xkcd illustrates the problem.20 A scientist who tries to correlate the incidence of acne with consumption of jelly beans of a particular color, and who runs the experiment over and over with different colors of jelly beans, will eventually get a statistically significant result. That result will almost certainly be meaningless—in Munroe’s version, the experimenters come up with p < .05 one time out of twenty, which is exactly how often a scientist would expect to see a “false positive” as a result of repeated tests. An unscrupulous researcher, or a careless one, can keep testing pairs of variables until he gets that statistically significant result that will convince people to pay attention to his research. Statisticians use the term “p-hacking” to describe the process of using repeated statistical tests to produce a result with spurious statistical significance.21 Which brings us back to Brian Wansink.
Wansink’s Dubious Science
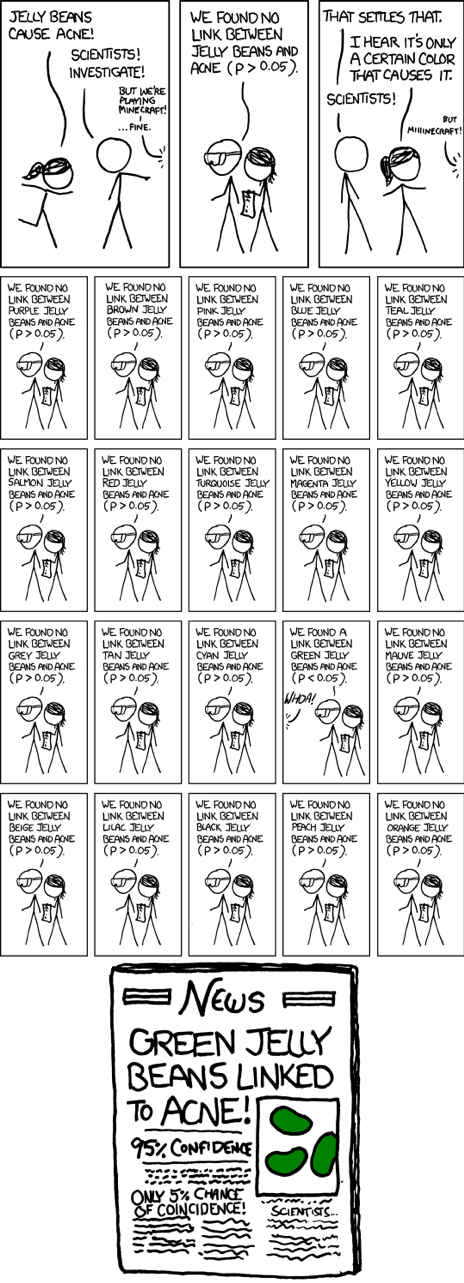
Wansink admitted that his data provided no support in terms of statistical significance for his original research hypothesis. So he gave his data set to a graduate student and encouraged her to run more tests on the data with new research hypotheses (“Plan B, C, & D”) until she came up with statistically significant results. Then she submitted these results for publication—and they were accepted. But how many tests of statistical significance did she run, relative to the number of statistically significant results she got? And how many “backup plans” should researchers be allowed? Researchers who use the scientific method are supposed to formulate hypotheses based on existing data and then gather new data to put their hypotheses to the test. But a scientist whose original hypothesis doesn’t pan out isn’t supposed to use the data he’s gathered to come up with a new hypothesis that he can “support” using that same data. A scientist who does that is like the Texan who took pot shots at the side of his barn and then painted targets around the places where he saw the most bullet holes.22
It’s easy to be a sharpshooter that way, which is why the procedure that Wansink urged on his graduate student outraged so many commenters. As one of them wrote: “What you describe Brian does sound like p-hacking and HARKing [hypothesizing after the results are known].”23 Wansink’s procedures had hopelessly compromised his research. He had, in effect, altered his research procedures in the middle of his experiment and authorized p-hacking to obtain a publishable result.
That wasn’t all Wansink had done wrong. Wansink’s inadvertent admissions led his critics to look closely at all aspects of his published research, and they soon found basic statistical mistakes throughout his work. Wansink had made more than 150 statistical errors in four papers alone, including “impossible sample sizes within and between articles, incorrectly calculated and/or reported test statistics and degrees of freedom, and a large number of impossible means and standard deviations.” He’d made further errors as he described his data and constructed the tables that presented his results.24 Put simply, a lot of Wansink’s numbers didn’t add up.
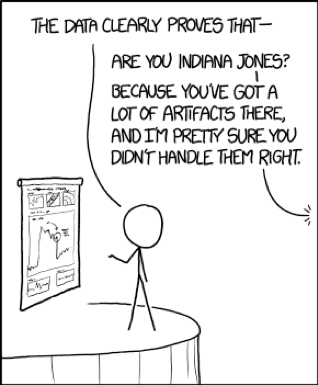
Wansink’s critics found more problems the closer they looked. In March 2017 a graduate student named Tim van der Zee calculated that critics had already made serious, unrebutted allegations about the reliability of 45 of Wansink’s publications. Collectively, these publications spanned twenty years of research, had appeared in twenty-five different journals and eight books, and— most troubling of all—had been cited more than 4,000 times.25 Wansink’s badly flawed research tainted the far larger body of scientific publications that had relied on the accuracy of his results.
Wansink seems oddly unfazed by this criticism.26 He acts as if his critics are accusing him of trivial errors, when they’re really saying that his mistakes invalidate substantial portions of his published research. Statistician Andrew Gelman,27 the director of Columbia University’s Applied Statistics Center,28 wondered on his widely-read statistics blog what it would take for Wansink to see there was a major problem.
Let me put it this way. At some point, there must be some threshold where even Brian Wansink might think that a published paper of his might be in error—by which I mean wrong, really wrong, not science, data not providing evidence for the conclusions. What I want to know is, what is this threshold? We already know that it’s not enough to have 15 or 20 comments on Wansink’s own blog slamming him for using bad methods, and that it’s not enough when a careful outside research team finds 150 errors in the papers. So what would it take? 50 negative blog comments? An outside team finding 300 errors? What about 400? Would that be enough? If the outsiders had found 400 errors in Wansink’s papers, then would he think that maybe he’d made some serious errors[?]29
Wansink and his employer, Cornell University, have not even fully addressed the first round of criticism about Wansink’s work,30 much less the graver follow-up critiques.31
But Wansink’s apparent insouciance may reflect a real feeling that he hasn’t done anything much wrong. After all, lots of scientists conduct their research in much the same way.
Wansink is Legion
Wansink acted like many of his peers. Even if most researchers aren’t as careless as Wansink, the research methods that landed Wansink in hot water are standard operating practice across a range of scientific and social-scientific disciplines. So too are many other violations of proper research methodology. In recent years a growing chorus of critics has called attention to the existence of a “reproducibility crisis”—a situation in which many scientific results are artifacts of improper research techniques, unlikely to be obtained again in any subsequent investigation, and therefore offering no reliable insight into the way the world works.
In 2005, Dr. John Ioannidis, then a professor at the University of Ioannina Medical School in Greece, made the crisis front-page news among scientists. He argued, shockingly and persuasively, that most published research findings in his own field of biomedicine probably were false. Ioannidis’ argument applied to everything from epidemiology to molecular biology to clinical drug trials.32 Ioannidis began with the known risk of a false positive any time researchers employed a test of statistical significance; he then enumerated a series of additional factors that tended to increase that risk. These included 1) the use of small sample sizes;33 2) a willingness to publish studies reporting small effects; 3) reliance on small numbers of studies; 4) the prevalence of fishing expeditions to generate new hypotheses or explore unlikely correlations;34 5) flexibility in research design; 6) intellectual prejudices and conflicts of interest; and 7) competition among researchers to produce positive results, especially in fashionable areas of research. Ioannidis demonstrated that when you accounted for all the factors that compromise modern research, a majority of new research findings in biomedicine—and in many other scientific fields—were probably wrong.
Ioannidis accompanied his first article, which provided theoretical arguments for the existence of a reproducibility crisis, with a second article that provided convincing evidence of its reality. Ioannidis compared 49 highly cited articles in clinical research to later studies on the same subjects. 45 of these articles had claimed an effective intervention, but “7 (16%) were contradicted by subsequent studies, 7 others (16%) had found effects that were stronger than those of subsequent studies, 20 (44%) were reproduced, and 11 (24%) remained largely unchallenged.” In other words, subsequent investigations provided support for fewer than half of these influential publications.35 A 2014 article co-authored by Ioannidis on 37 reanalyses of data from randomized clinical trials also found, with laconic understatement, that 13 of the reanalyses (35%) “led to interpretations different from that of the original article.”36 Perhaps Ioannidis had put it too strongly back in 2005 when he wrote that a majority of published research findings might be false. In medicine, the proportion may be more like one third. But that number would still be far too high—especially given the huge and expanding costs of medical research—and it still suggests the crisis is real.
The Scope of the Crisis
Ioannidis’ alarming papers crystallized the scientific community’s awareness of the reproducibility crisis— and not just among scientists conducting medical research. Ioannidis said that his arguments probably applied to “many current scientific fields.” Did they? To the same extent? If so many findings from clinical trials didn’t reproduce, what did that suggest for less rigorous disciplines, such as psychology, sociology, or economics?
Scientists scrutinizing their own fields soon discovered that many widely reported results didn’t replicate.37 In the field of psychology, researchers’ reexamination of “power posing”—stand more confidently and you will be more successful—suggested that the original result had been a false positive.38 In sociology, reexamination brought to light major statistical flaws in a study that claimed that beautiful people have more daughters.39 Andrew Gelman judged that a study of the economic effects of climate change contained so many errors that “the whole analysis [is] close to useless as it stands.”40
Some of the research that failed to reproduce had been widely touted in the media. “Stereotype threat” as an explanation for poor academic performance? Didn’t reproduce.41 “Social priming,” which argues that unnoticed stimuli can significantly change behavior? Didn’t reproduce that well,42 and one noted researcher in the field was an outright fraud.43 Tests of implicit bias as predictors of discriminatory behavior? The methodology turned out to be dubious,44 and the test of implicit bias may have been biased itself.45 Oxytocin (and therefore hugs, which stimulate oxytocin production) making people more trusting? A scientist conducting a series of oxytocin experiments came to believe that he had produced false positives—but he had trouble publishing his new findings.46
Deep-rooted “perceptual” racial bias? The argument depended on several research reports all producing positive results, and a statistical analysis revealed that the probability that such a series of experiments would all yield positive results was extremely low, even if the effects in question were real.
The probability that five studies like these would all be uniformly successful is ... 0.070; and the low value suggests that the reported degree of success is unlikely to be replicated by future studies with the same sample sizes and design. Indeed, the probability is low enough that scientists should doubt the validity of the experimental results and the theoretical ideas presented.47
Not every famous study failed to reproduce. Scholars have criticized the Milgram Experiment (1963)48—in which Stanley Milgram induced large numbers of study participants to give electric shocks (they believed) to unseen “experimental subjects,” up to the point of torture and death— for both shoddy research techniques and data manipulation.49 Yet the experiment substantially reproduced twice, in 2009 and 2015.50 The Milgram Experiment seemed too amazing to be true, and it may have been conducted sloppily the first time around—but replication provided significant confirmation. The crisis of reproducibility doesn’t mean that all recent research findings are wrong—just a large number of them.51
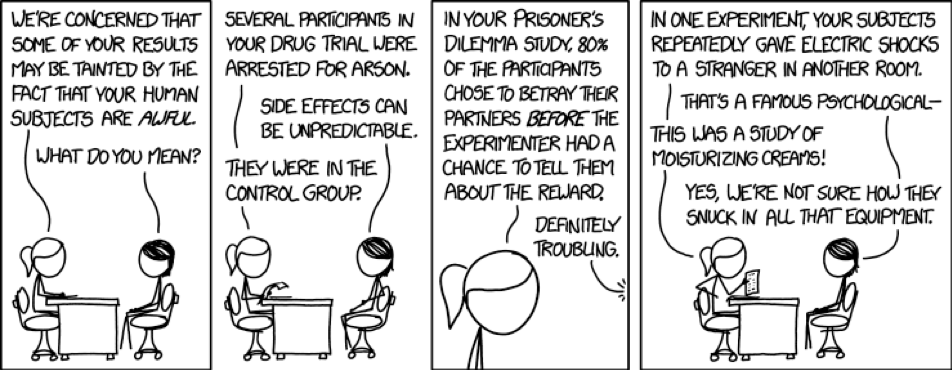
Recent evidence suggests that the crisis of reproducibility has compromised entire disciplines. In 2012 the biotechnology firm Amgen tried to reproduce 53 “landmark” studies in hematology and oncology, but could only replicate 6 (11%).52 That same year Janet Woodcock, director of the Center for Drug Evaluation and Research at the Food and Drug Administration, “estimated that as much as 75 per cent of published biomarker associations are not replicable.”53 A 2015 article in Science that presented the results of an attempt to replicate 100 articles published in three prominent psychological journals in 2008 found that only 36% of the replication studies produced statistically significant results, compared with 97% of the original studies—and on average the effects found in the replication studies were half the size of those found in the original research.54 Another study in 2015 could not reproduce a majority of a sample of 67 reputable economics articles.55 A different study in the economics field successfully reproduced a larger proportion of research, but a great deal still failed to reproduce: 61% of the replication efforts (11 out of 18) showed a significant effect in the same direction as the original research, but with an average effect size reduced by one-third.56
In 2005, scientists could say that Ioannidis’ warnings needed more substantiation. But we now have a multitude of professional studies that corroborate Ioannidis. Wansink provides a particularly vivid illustration of Ioannidis’ argument.
Why does so much research fail to replicate? Bad methodology, inadequate constraints on researchers, and a professional scientific culture that creates incentives to produce new results—innovative results, trailblazing results, exciting results—have combined to create the reproducibility crisis.
Problematic Science
Flawed Statistics
The reproducibility crisis has revealed many kinds of technical problems in medical studies; and Wansink committed a large number of them in his behavioral research. Several researchers have narrowed their focus and studied the effects of p-hacking on scientific research. Megan Head’s 2015 study looked at p-values in papers across a range of disciplines and found evidence that p-hacking is “widespread throughout science.”57 However, Head and her co-authors downplayed the significance of that finding and argued that most p-hacking probably just confirmed hypotheses that were fundamentally true. A 2016 paper coauthored by Ioannidis seemed to demolish those reassurances,58 but another paper revisiting Head’s study argued that she and her co-authors overestimated the evidence for p-hacking.59 A separate paper that examined social science data found “encouragingly little evidence of false-positives or p-hacking in rigorous policy research,”60 but the qualifier “rigorous” sidesteps the question of how much policy research does not meet rigorous standards. Still, these initial results suggest that while p-hacking significantly afflicts many disciplines, it is not pervasive in any of them.
P-hacking may not be as widespread as one might fear, but it appears that many scientists who routinely use p-values and statistical significance testing misunderstand those concepts, and therefore employ them improperly in their research.61 In March 2016, the Board of Directors of the American Statistical Association issued a “Statement on Statistical Significance and p-Values” to address common misconceptions. The Statement’s six enunciated principles included the admonition that “by itself, a p-value does not provide a good measure of evidence regarding a model or hypothesis.”62
Such warnings are vital, but, as the Wansink affair illustrates, scientists also make many other sorts of errors in their use of statistical tests.63 The mathematics of advanced statistical methods are difficult, and many programs of study do not adequately train their graduates to master them.64 The development of powerful statistical software also makes it easy for scientists who don’t fully understand statistics to let their computers perform statistical tests for them. Jeff Leek, one of the authors of the popular blog Simply Statistics, put it bluntly in 2014: “The problem is not that people use p-values poorly, it is that the vast majority of data analysis is not performed by people properly trained to perform data analysis.”65
Faulty Data
Statistical analysis isn’t the only way research goes wrong. Scientists also produce supportive statistical results from recalcitrant data by fiddling with the data itself. Researchers commonly edit their data sets, often by excluding apparently bizarre cases (“outliers”) from their analyses. But in doing this they can skew their results: scientists who systematically exclude data that undermines their hypotheses bias their data to show only what they want to see.
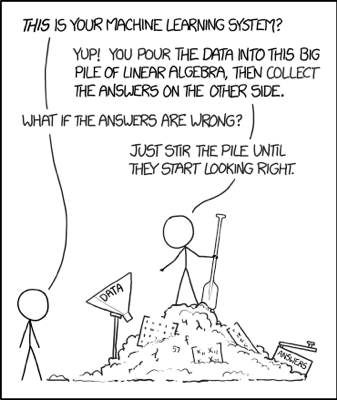
Scientists can easily bias their data unintentionally, but some deliberately reshape their data set to produce a particular outcome. One anonymized survey of more than 2,000 psychologists found that 38% admitted to “deciding whether to exclude data after looking at the impact of doing so on the results.”67 Few researchers have published studies of this phenomenon, but anecdotal evidence suggests it is widespread. In neuroscience,
there may be (much) worse things out there, like the horror story someone (and I have reason to believe them) told me of a lab where the standard operating mode was to run a permutation analysis by iteratively excluding data points to find the most significant result. … The only difference from [sic] doing this and actually making up your data from thin air ... is that it actually uses real data – but it might as well not for all the validity we can expect from that.68
Researchers can also bias their data by ceasing to collect data at an arbitrary point, perhaps the point when the data that has already been collected finally supports their hypothesis. Conversely, a researcher whose data doesn’t support his hypothesis can decide to keep collecting additional data until it yields a more congenial result. Such practices are all too common. The survey of 2,000 psychologists noted above also found that 36% of those surveyed “stopped data collection after achieving the desired result.”69
Another sort of problem arises when scientists try to combine, or “harmonize,” multiple preexisting data sets and models in their research—while failing to account sufficiently for how such harmonization magnifies the uncertainty of their conclusions. Claudia Tebaldi and Reto Knutti concluded in 2007 that the entire field of probabilistic climate projection, which often relies on combining multiple climate models, had no verifiable relation to the actual climate, and thus no predictive value. Absent “new knowledge about the [climate] processes and a substantial increase in computational resources,” adding new climate models won’t help: “our uncertainty should not continue to decrease when the number of models increases.”70
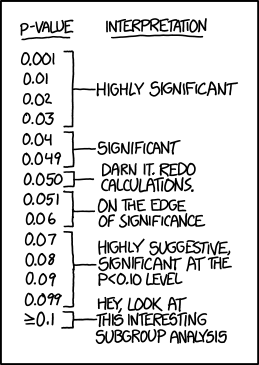
Pervasive Pitfalls
Necessary and legitimate research procedures drift surprisingly easily across the line into illegitimate manipulations of the techniques of data collection and analysis. Researcher decisions that seem entirely innocent and justifiable can produce “junk science.” In a 2014 article in the American Scientist, Andrew Gelman and Eric Loken called attention to the many ways researchers’ decisions about how to collect, code, analyze, and present data can vitiate the value of statistical significance.71 Gelman and Loken cited several researchers who failed to find a hypothesized effect for a population as a whole, but did find the effect in certain subgroups. The researchers then formulated explanations for why they found the postulated effect among men but not women, the young but not the old, and so on. These researchers’ procedures amounted not only to p-hacking but also to the deliberate exclusion of data and hypothesizing after the fact: they were guaranteed to find significance somewhere if they examined enough subgroups.
Researchers allowed to choose between multiple measures of an imperfectly defined variable often decide to use the one which provides a statistically significant result. Gelman and Loken called attention to a study that purported to find a relationship between women’s menstrual cycles and their choice of what color shirts to wear.72 They pointed out that the researchers framed their hypothesis far too loosely:
Even though Beall and Tracy did an analysis that was consistent with their general research hypothesis—and we take them at their word that they were not conducting a “fishing expedition”—many degrees of freedom remain in their specific decisions: how strictly to set the criteria regarding the age of the women included, the hues considered as “red or shades of red,” the exact window of days to be considered high risk for conception, choices of potential interactions to examine, whether to combine or contrast results from different groups, and so on.73
Would Beall and Tracy’s hypothesis have produced statistically significant results if they had made different choices in analyzing their data? Perhaps. But a belief in the very hypothesis whose validity they were attempting to confirm could have subtly influenced at least some of their choices.
Facilitating Falsehood
The Costs of Researcher Freedom
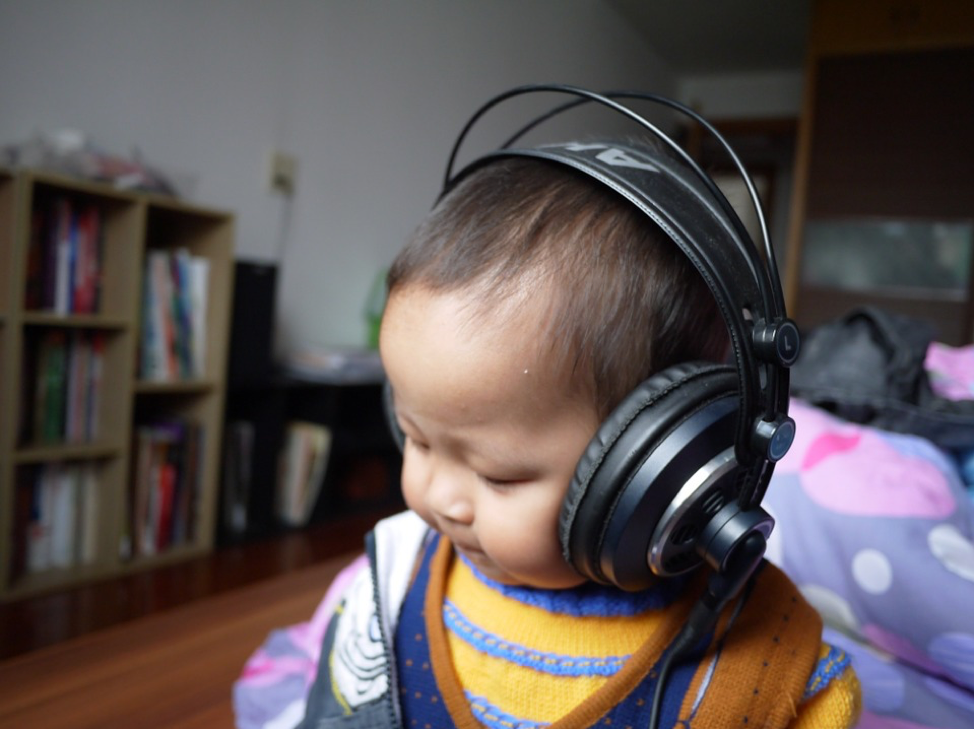
Why do researchers get away with sloppy science? In part because, far too often, no one is watching and no one is there to stop them. We think of freedom as a good thing, but in the realm of scientific experimentation, uncontrolled researcher freedom makes it easy for scientists to err in all the ways described above.74 The fewer the constraints on scientists’ research designs, the more opportunities for malfeasance—and, as it turns out, a lot of scientists will go astray, deliberately or accidentally. For example, lack of constraints allows researchers to alter their methods midway through a study—changing hypotheses, stopping or recommencing data collection, redefining variables, “fine-tuning” statistical models—as they pursue publishable, statistically significant results. Researchers often justify midstream alteration of research procedures as flexibility or openness to new evidence75—but in practice such “flexibility” frequently subserves scientists’ unwillingness to accept a negative result.
Researchers sometimes have good reasons to alter a research design before a study is complete—for example, if a proposed drug in a clinical trial appears to be causing harm to the experimental subjects.76 (Though scientists can take even this sort of decision too hastily.77) But researchers also stop some clinical trials early on the grounds that a treatment’s benefits are already apparent and that it would be wrong to continue denying that treatment to the patients in the control group. Such truncated clinical trials pose grave ethical hazards: as one discussion put it, truncated trials “systematically overestimate treatment effects” and can violate “the ethical research requirement of scientific validity.”78 Moreover, a 2015 article in the Journal of Clinical Epidemiology indicated that “most discontinuations of clinical trials were not based on preplanned interim analyses or stopping rules.”79 In other words, most decisions to discontinue were done on the fly, without regard for the original research design. The researchers changed methodology midstream.
A now-famous 2011 article by Simmons, Nelson, and Simonsohn estimated that providing four “degrees of researcher freedom”—four ways to shift the design of an experiment while it is in progress—can lead to a 61% false-positive rate. Or, as the subtitle of the article put it, “Undisclosed Flexibility in Data Collection and Analysis Allows Presenting Anything as Significant.” Simmons and his co-authors demonstrated their point by running an experiment to see if listening to selected songs will make you, literally, younger. Their flexible research design produced data that revealed an effect of 18 months, with p = .040.80
Absence of Openness
Lack of openness also contributes to the reproducibility crisis. Investigators far too rarely share data and methodology once they complete their studies. Scientists ought to be able to check and critique one another’s work, but many studies can’t be evaluated properly because researchers don’t make their data and procedures available to the public. We’ve seen that small changes in research design can have large effects on researchers’ conclusions. Yet once scientists publish their research, those small changes vanish from the record, and leave behind only the statistically significant result. For example, the methods used in meta-analyses to harmonize cognitive measures across data sets “are rarely reported.”81 But someone reading the results of a meta-analysis can’t understand it properly without a detailed description of the harmonization methods and of the codes used in formatting the data.
Moreover, data sets often come with privacy restrictions, usually to protect personal, commercial, or medical information. Some restrictions make sense—but others don’t. Sometimes unreleased data sets simply vanish—for example, those used in environmental science.82 Data sets can disappear because of archival failures, or because of a failure to plan how to transfer data into new archival environments that will provide reliable storage and continuing access. In either case, other researchers lose the ability to examine the underlying data and verify that it has been handled properly.
In February 2017, a furor that highlighted the problem of limited scientific openness erupted in the already contentious field of climate science. John Bates, a climate scientist who had recently retired from the National Oceanic and Atmospheric Administration (NOAA), leveled a series of whistleblowing accusations at his colleagues.83 He focused on the failure by Tom Karl, the head of NOAA’s National Centers for Environmental Information, to archive properly the dataset that substantiated Karl’s 2015 claim to refute evidence of a global warming hiatus since the early 2000s.84 Karl’s article had been published shortly before the Obama administration submitted its Clean Power Plan to the 2015 Paris Climate Conference, and it had received extensive press coverage.85 Yet Karl’s failure to archive his dataset violated NOAA’s own rules—and also the guidelines of Science, the prestigious journal that had published the article. Bates’ criticisms touched off a political argument about the soundness of Karl’s procedures and conclusions, but the data’s disappearance meant that no scientist could re-examine Karl’s work. Supporters and critics of Karl had to conduct their argument entirely in terms of their personal trust in Karl’s professional reliability. Practically, the polarized nature of climate debate meant that most disputants believed or disbelieved Karl depending upon whether they believed or disbelieved his conclusions. Science should not work that way—but without the original data, scientific inquiry could not work at all.
Both scientists and the public should regard skeptically research built upon private data. Gelman responded appropriately, if sarcastically, to Wansink’s refusal to share his data on privacy grounds:
Some people seem to be upset that Wansink isn’t sharing his data. If he doesn’t want to share the data, there’s no rule that he has to, right? It seems pretty simple to me: Wansink has no obligation whatsoever to share his data, and we have no obligation to believe anything in his papers. No data, no problem, right?86
The Wages of Sin: the Professional Culture of Science
The crisis of reproducibility arises at the nuts-and-bolts level from the technical mishandling of data and statistics. Uncontrolled researcher freedom and a lack of openness enable scientific malfeasance or the innocent commission of serious methodological mistakes. At the highest level, however, the crisis of reproducibility also derives from science’s professional culture, which provides incentives to handle statistics and data sloppily and to replace rigorous research techniques with a results-oriented framework. The two most dangerous aspects of this professional culture are the premium on positive results and groupthink.
The Premium on Positive Results
Modern science’s professional culture prizes positive results, and offers relatively few rewards to those who fail to find statistically significant relationships in their data. It also esteems apparently groundbreaking results far more than attempts to replicate earlier research. Ph.D.s, grant funding, publications, promotions, lateral moves to more prestigious universities, professional esteem, public attention—they all depend upon positive results that seem to reveal something new. A scientist who tries to build his career on checking old findings or publishing negative results isn’t likely to get very far. Scientists therefore steer away from replication studies, and they often can’t help looking for ways to turn negative results into positive ones. If those ways can’t be found, the negative results go into the file drawer.
Common sense says as much to any casual observer of modern science, but a growing body of research has documented the extent of the problem. As far back as 1987, a study of the medical literature on clinical trials showed a publication bias toward positive results.87 Later studies provided further evidence that the phenomenon affects an extraordinarily wide range of fields, including the social sciences generally,88 climate science,89 psychology,90 sociology,91 research on drug education,92 research on informational technology in education,93 research on “mindfulness-based mental health interventions,”94 and even dentistry.95
Groupthink
Public knowledge about the pressure to publish is fairly widespread. The effects of groupthink on scientific research are less widely known, less obvious, and far more insidious.
Academic psychologist Irving Janis invented the concept of groupthink—“a psychological drive for consensus at any cost that suppresses dissent and appraisal of alternatives in cohesive decision making groups.”96 Ironically, groupthink afflicts academics themselves, and contributes significantly to science’s crisis of reproducibility. Groupthink inhibits attempts to reproduce results that provide evidence for what scientists want to believe, since replication studies can undermine congenial conclusions. When a result appears to confirm its professional audience’s preconceptions, no one wants to go back and double-check whether it’s correct.
An entire academic discipline can succumb to groupthink, and create a professional consensus with a strong tendency to reinforce itself, reject results that question its foundations, and dismiss dissenters as troublemakers and cranks.97 Examples of groupthink can be found throughout the history of science. A generation of obstetricians ignored Ignaz Semmelweis’ call for them to wash their hands before delivering babies.98 Groupthink also contributed to the consensus among nutritionists that saturated fats cause heart disease, and to their refusal to consider the possibility that sugar was the real culprit.99
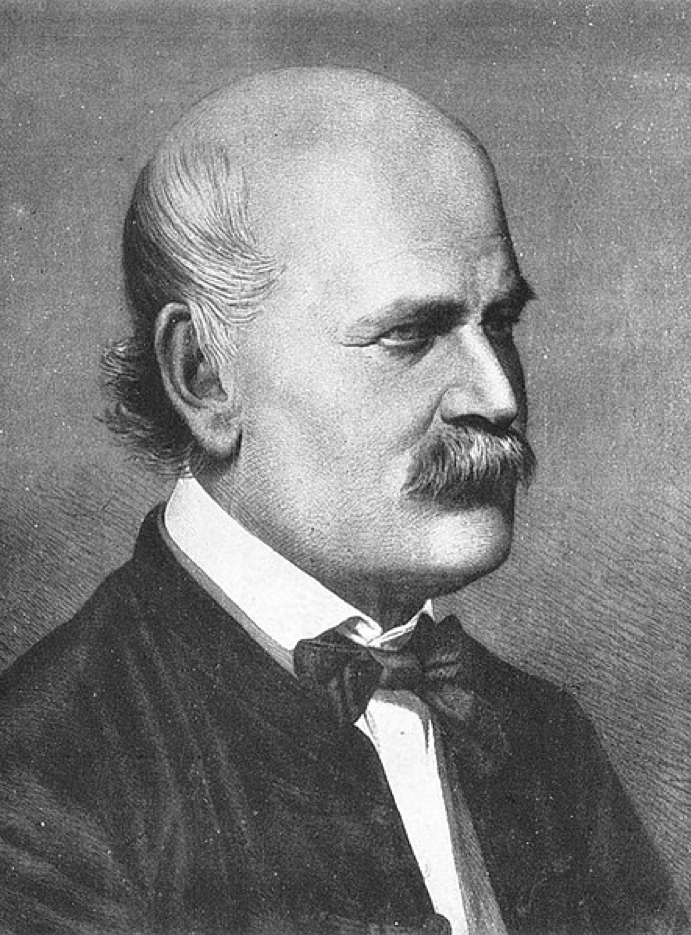
Some of the groupthink afflicting scientific research is political. Numerous studies have shown that the majority of academics are liberals and progressives, with relatively few moderates and scarcely any conservatives among their ranks.100 Social psychologist Jonathan Haidt made this point vividly at the Society for Personality and Social Psychology’s annual conference in 2011, when he asked the audience to indicate their political affiliations.
[Haidt began] by asking how many considered themselves politically liberal. A sea of hands appeared, and Dr. Haidt estimated that liberals made up 80 percent of the 1,000 psychologists in the ballroom. When he asked for centrists and libertarians, he spotted fewer than three dozen hands. And then, when he asked for conservatives, he counted a grand total of three.101
The Heterodox Academy, which Haidt helped found in 2015, argues that the overwhelming political homogeneity of academics has created groupthink that distorts academic research.102 Scientists readily accept results that confirm liberal political arguments,103 and frequently reject contrary results out of hand. Political groupthink particularly affects some fields with obvious political implications, such as social psychology104 and climate science.105 Climatologist Judith Curry testified before Congress in 2017 about the pervasiveness of political groupthink in her field:
The politicization of climate science has contaminated academic climate research and the institutions that support climate research, so that individual scientists and institutions have become activists and advocates for emissions reductions policies. Scientists with a perspective that is not consistent with the consensus are at best marginalized (difficult to obtain funding and get papers published by ‘gatekeeping’ journal editors) or at worst ostracized by labels of ‘denier’ or ‘heretic.’106
But politicized groupthink can bias scientific and social-scientific research in any field that acquires political coloration.
Like-minded academics’ ability to define their own discipline by controlling publication, tenure, and promotions exacerbates groupthink. These practices silence and purge dissenters, and force scientists who wish to be members of a field to give “correct” answers to certain questions. The scientists who remain in the field no longer realize that they are participating in groupthink, because they have excluded any peers who could tell them so.
Dire Consequences
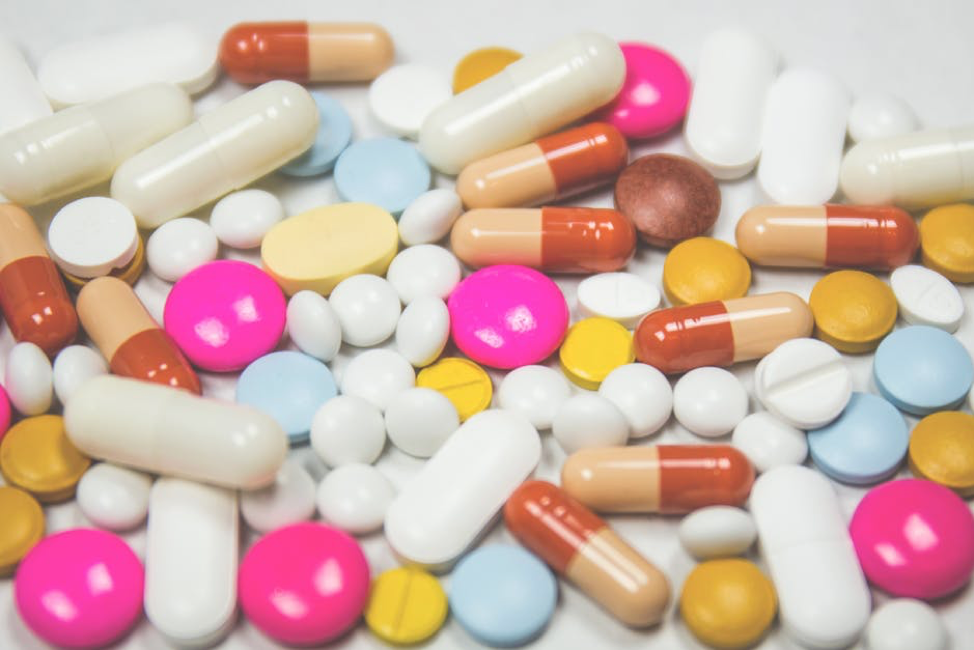
Just the financial consequences of the reproducibility crisis are enormous: a 2015 study estimated that researchers spent around $28 billion annually in the United States alone on irreproducible preclinical research for new drug treatments.107 Drug research inevitably will proceed down some blind alleys—but the money isn’t wasted so long as scientists know they came up with negative results. Yet it is waste, and waste on a massive scale, to spend tens of billions of dollars on research that scientists mistakenly believe produced positive results.
Beyond the dollars and cents, ordinary citizens, policymakers, and scientists make an immense number of harmful decisions on the basis of irreproducible research. Individuals cumulatively waste large amounts of money and time as they practice “power poses” or follow Brian Wansink’s weight-loss advice. The irreproducible research of entire disciplines distorts public policy and public expenditure in areas such as public health, climate science, and marriage and family law. The gravest casualty of all is the authority that science ought to have with the public, but which it begins to forfeit when it no longer produces reliable knowledge.
Modern science must reform itself to redeem its credibility.
What Is To Be Done?
What Has Been Done
Why didn’t Brian Wansink change his lab procedures back in 2005, when John Ioannidis published his seminal articles? Why didn’t all the other Wansinks heed the same warnings? Scientists don’t change how they conduct research overnight, and many still use the same techniques they used a generation ago. Some of their caution was reasonable—research procedures shouldn’t change on a dime. Yet a flood of evidence provides compelling confirmation that modern science must reform. A critical mass of scientists now realizes that research cannot go on in the old way.
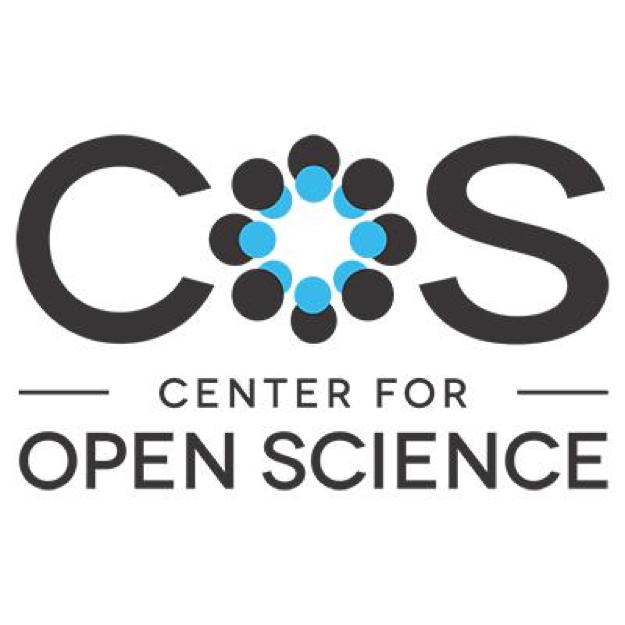
Many researchers and interested laymen have already begun to improve the practice of science. In a recent survey of 1,500 scientists published in Nature, “one-third of respondents said that their labs had taken concrete steps to improve reproducibility within the past five years. Rates ranged from a high of 41% in medicine to a low of 24% in physics and engineering.”108 At the same time, new programs and organizations have been created to take on the reproducibility crisis. A notable example of such an organization is the Center for Open Science, co-founded by two psychologists, Brian Nosek and Jeffrey Spies, and funded by the Laura and John Arnold Foundation.109 The Center’s major initiative has been Nosek’s Reproducibility Project, dedicated to estimating the reproducibility of psychological research.110 A second Reproducibility Project now focuses on cancer research.111 The Center also supports the $1 million Preregistration Challenge, which is “giving away $1,000 [each] to 1,000 researchers who preregister their projects before they publish them.”112 The Arnold Foundation, meanwhile, has become what John Ioannidis calls “the Medici of meta-research,” and funds a wide range of projects intended to solve the reproducibility crisis.113 By 2017, the Arnolds had given more than $80 million through their Research Integrity Initiative,114 including $6 million to Ioannidis’ Meta- Research Innovation Center at Stanford (METRICS), which focuses on the reproducibility of biomedical research.115
Some scientific journals have also started to fight the crisis. In 2014, Psychological Science introduced submission guidelines that asked researchers not to describe findings as “statistically significant” and to give detailed reasons for the exclusion of data from analyses. The journal also began to award “badges” for good research practices, such as making data and research protocols publicly available and preregistering research procedures prior to data collection.116 Since Psychological Science formulated these new guidelines, research published in the journal has become substantially more transparent: “the number of papers describing their criteria for excluding data from analysis increased by 53 percentage points, and the number making full datasets available increased by 36 percentage points.”117
Entirely new journals have sprung up that combat publication bias by publishing negative results. These new journals include The All Results Journals (chemistry, biology, physics, and nanotechnology),118 the Journal of Articles in Support of the Null Hypothesis (psychology),119 the Journal of Pharmaceutical Negative Results,120 the Journal of Negative Results in Biomedicine,121 and the Journal of Negative Results (ecology & evolutionary biology).122 The International Journal for Re-Views in Empirical Economics devotes itself to replication studies in economics,123 while Claremont McKenna College’s Program on Empirical Legal Studies will hold a conference in 2018 devoted to replication in that field.124 At least one international organization has joined the quest to reform science: the World Health Organization now calls for both data openness and the publication of negative results: “Researchers have a duty to make publicly available the results of their research ... Negative and inconclusive as well as positive results must be published or otherwise made publicly available.”125
The publicity about the crisis of reproducibility is itself encouraging. Andrew Gelman notes that psychology has received far more bad publicity about its practices precisely because psychology allows more open examination of its procedures and data than do its sister disciplines.126 The evidence of psychology gone wrong also serves as evidence that psychology can go right—and the same holds true for the other sciences. They possess the methodological resources and the dedicated practitioners that can make these fallen disciplines honest. Scientists have begun to right the course of modern science in the thirteen years since John Ioannidis sounded the alarm.
But they have only begun. The institutions of modern science are enormous; far from all scientists believe there is a crisis; and the campaign to fix the crisis of reproducibility still requires a great deal of work. The National Academies of Sciences, Engineering, and Medicine (NASEM) makes many useful recommendations in its publication Fostering Integrity in Research (2017)—but it is unfortunate that when NASEM recommends the establishment of an independent nonprofit Research Integrity Advisory Board (RIAB), it specifies that “the RIAB will have no direct role in investigations, regulation, or accreditation.”127 Such toothless measures will not suffice. A long-term solution will need to address the crisis at every level—technical competence, institutional practices, and professional culture.128
Better Statistics
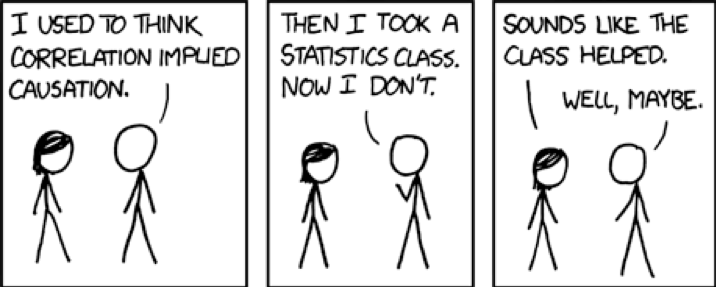
Much of the crisis of reproducibility derives from researchers’ limited understanding of their own statistical toolkits, and solving the crisis will require better statistical education for scientists and social scientists.129 As we mentioned earlier, in 2016 the American Statistical Association (ASA) issued a formal statement to call attention to the different ways a researcher can misunderstand and therefore misuse p-values. Among other admonitions, the ASA warned that “p-values do not measure the probability that the studied hypothesis is true, or the probability that the data were produced by random chance alone,” that “a p-value, or statistical significance, does not measure the size of an effect or the importance of a result,” and that “by itself a p-value does not provide a good measure of evidence regarding a model or hypothesis.”130 The basic training of researchers in disciplines that rely heavily on statistical methods ought to highlight these warnings, and others of a similar nature.
As an immediate practical measure, researchers in all disciplines should adopt the best existing practice of the most rigorous sciences, and define statistical significance as p < .01 rather than as p < .05. In 2017, 72 noted statisticians and scientists recommended in Nature Human Behavior that “for fields where the threshold for defining statistical significance for new discoveries is p < 0.05, we propose a change to p < 0.005. This simple step would immediately improve the reproducibility of scientific research in many fields.”131 Given the ease with which researchers can accidentally or deliberately manipulate p-values, p < .01 should be the loosest recognized standard of statistical significance, not the most rigorous.
A growing number of scientists now reject the idea of statistical significance altogether.132 Although the sciences and social sciences would be improved if they adopted a more rigorous standard of significance, there’s nothing magical about any particular cutoff. That’s why Psychological Science now discourages its contributors from describing their findings as statistically significant. Yet a low p-value may still bewitch readers, even if the phrase “statistically significant” doesn’t appear. Scientists should stop regarding the p-value as a dispositive measure of evidentiary support for a particular hypothesis. Basic and Applied Social Psychology (BASP) took a decisive step when it announced in 2015 that it would ban “null hypothesis significance testing procedure (NHSTP)” and cease to publish the results of tests of statistical significance. Scientists could still include such tests in their initial submissions, but “prior to publication, authors will have to remove all vestiges of the NHSTP (p-values t-values, F-values, statements about ‘significant’ differences or lack thereof, and so on.)”133
Other journals that share BASP’s judgment that the crisis of reproducibility requires major corrective measures may wish to look for alternative ways to provide a quantitative indication of the strength of a hypothesis. Such journals should consider employing confidence intervals, which provide a range in which a variable’s value most likely falls. Researchers typically use a 95% standard for confidence intervals, which means that a variable’s “true” value should fall within the indicated range 95% of the time.
Let’s return to an earlier example. If a researcher finds that, for the individuals in his data set, each additional year of schooling corresponds to an extra $750 of annual income, a confidence interval might show a 95% likelihood that, in the population as a whole, the increase in income associated with each year of schooling is between $10 and $1490. Zero is outside this range, so the research suggests that there is a real correlation between these two variables. Researchers who use a p < .05 standard would consider the result to be statistically significant. But researchers who report confidence intervals instead of p-values will at least highlight for their audience the breadth of the effect’s possible range, and therefore guard against an impulse to overstate the importance of the findings. Consider two claims. First: “There is a 95% chance that each additional year of schooling means at least $10 increased income per year, although the effect could be much larger.” Second: “Our research found a statistically significant association (p < .05) between each additional year of schooling and an increase in annual income of $750.” The first claim sounds more modest, and provides a more accurate picture of what the data actually shows.
Yet even professional scientists misunderstand confidence intervals134—and confidence intervals will mislead as much as p-values if the underlying data or statistical models are wrong.
Bayesian Inference
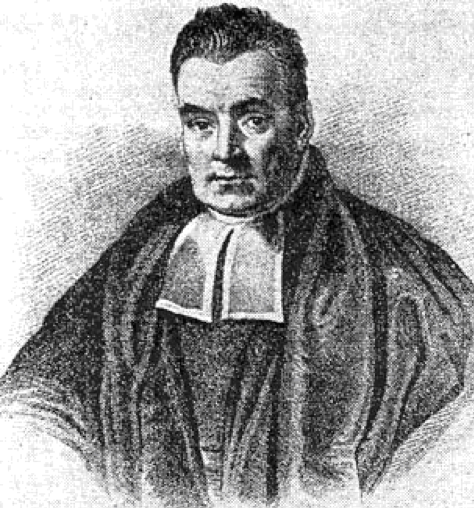
Some scientists employ the techniques of Bayesian inference135 as a way to correct researchers’ fixation on statistical significance as the way to evaluate hypotheses. Most advocates of Bayesian inference regard statistical tests as ways to update “prior probabilities”—preexisting estimates of how likely a hypothesis is to be true—rather than as definitive attempts to assess hypotheses’ validity.136 Although Bayesian statistical methods have their own limitations, Bayesians’ acknowledgment of prior probability can help both researchers and the public to avoid common statistical errors.
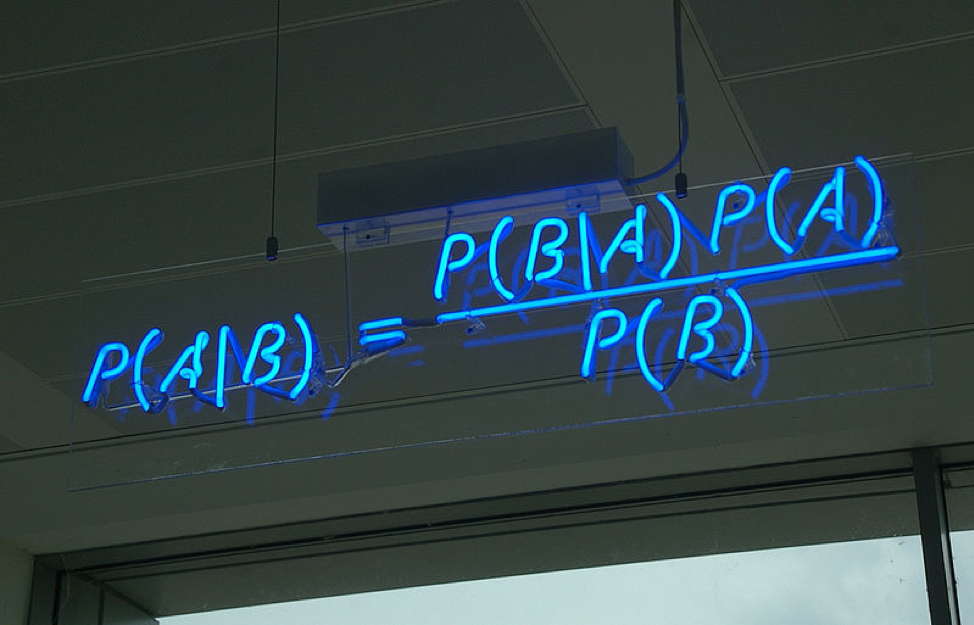
To see how Bayesian thinking works, imagine a woman named Joyce who is tested for an extremely rare disease that affects only one in ten thousand people. The test will detect the disease if it is present, but the test also has a false positive rate of 2 percent. Joyce’s test comes up positive—but this does not mean there is a 98 percent chance she has the disease. Our calculations should include the fact that Joyce’s chances of getting the disease in the first place were very low. If we take account of all the known probabilities, via a beautiful piece of mathematics called Bayes’ Theorem,137 the probability that Joyce has the disease is actually about half of one percent. The extremely low likelihood that she would have the disease in the first place more than counterbalances the low likelihood of a false positive on her test.
Additional evidence might alter these calculations. If Joyce took the test because she displayed symptoms associated with the disease, that evidence may substantially increase the likelihood that she has it. We should then estimate the probability that Joyce has symptoms but no disease. Perhaps her doctor can only guess at that probability— but her doctor should still make that guess. A central insight of Bayesianism is that a purely subjective guess is often better than not assessing a particular piece of evidence at all.
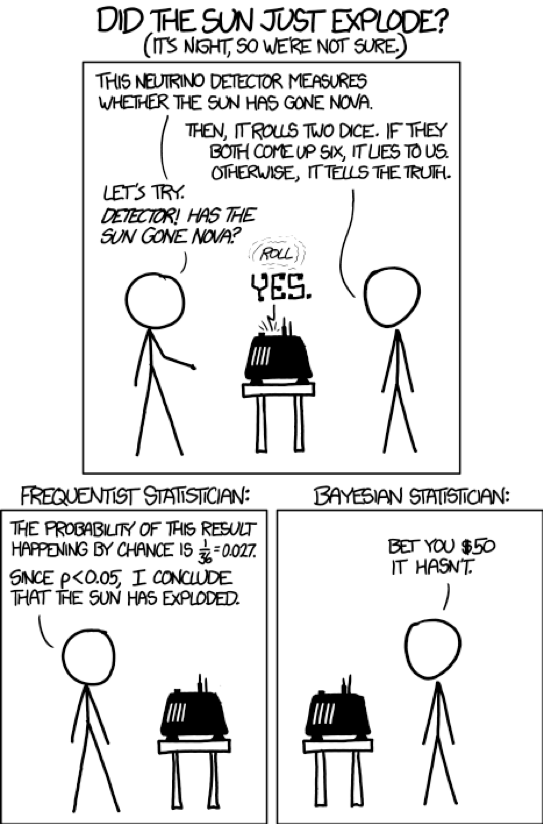
Two real murder trials, one in Maryland138 and one in the United Kingdom,139 provide a striking example of the dangers of not thinking in Bayesian terms. Both trials involved the deaths of two children in a single family, apparently from Sudden Infant Death Syndrome (SIDS). In both cases prosecutors charged a parent with murdering the children— and in both cases the prosecutors relied on statistical arguments. The prosecutors argued that since the odds of two children in one family dying of SIDS are miniscule, it was therefore overwhelmingly likely that the parent murdered the children. The juries in both cases voted to convict.
Subsequent appeals overturned both convictions, because the statistics experts in both cases failed to acquaint the juries with the relevant prior probabilities.140 In Maryland, the SIDS experts didn’t consider the possibility that SIDS might have a genetic link. In the United Kingdom, the experts didn’t tell the jury that the odds a mother would kill two of her own children were even lower than the odds that two children in one family would die of SIDS.
Let’s return to the sorts of research that created science’s reproducibility crisis. Scientists currently are far too likely to look at a statistically significant result and draw the conclusion that the hypothesis has a 95% chance of being true. A Bayesian approach foregrounds one of the most important reasons that this assumption is false—a scientist’s failure to estimate the likelihood that the hypothesis was true in the first place. If the hypothesis is unlikely to begin with—e.g., that you will become younger if you listen to a particular song—then a low p-value shrinks into near-insignificance in comparison with the tiny a priori likelihood that the theory is correct. Scientists who employ a Bayesian perspective transform their entire approach to research—and make it far less likely that they will rush to ascribe importance to a statistically significant result.
Increasing numbers of scientists believe that all scientific disciplines that resort to statistics ought to expect their members to be conversant with Bayesian approaches. Once researchers cease to regard statistical tests as conclusive assessments of the strength of a hypothesis, and use them instead as ways to adjust their estimations of the likelihood that a hypothesis is true, they will restore a salutary humility to the practice of science and banish the notion that any one study can settle an issue once and for all. A Bayesian outlook should also lead the scientific community to place greater value on studies that don’t produce low p-values, since these negative results will still allow them to improve their estimates of the truth of their hypotheses.
Less Freedom, More Openness
Unlimited freedom to tinker with a research design after data collection and analysis has already begun contributes significantly to the crisis of reproducibility. All scientists should adopt the familiar but still too rare practice of “pre-registering” their research protocols, and should file them in advance with an appropriate scientific journal, professional organization, or government agency.141 As per the recommendations of Simmons, Nelson, and Simonsohn, the psychologists who studied “degrees of researcher freedom,” pre-registered research protocols should include procedures for data collection, including instruments such as questionnaires; a list of all variables for which researchers will collect data; the rules researchers will follow to decide whether and when to terminate data collection; and detailed descriptions of the ways in which the data will be coded and analyzed.142 Peer reviewers should scrutinize research procedures during the pre-registration process, and offer warnings and suggest improvements before the research begins.
Researchers should then document all deviations from their pre-registered procedures during their research. Once they complete their study, researchers should disclose their methodology, including all documented departures from their research design and all other relevant experimental conditions. Simmons and his colleagues suggest that researchers also should provide the results of their statistical analysis under different conditions. For example, if researchers exclude some observations from their data, they should also report the results produced by including those observations.
Scientists should also make their data and all other relevant materials available to the world once they publish their research. They should include both their raw data and the datasets they constructed, and employ standardized descriptions of research materials and procedures.143 Researchers should experiment with born-open data—data archived in an open-access repository at the moment of its creation, and automatically time-stamped—as the ultimate guarantee against researcher tampering.144 The public will particularly welcome this sort of openness in fields such as climate research, where considerable controversy surrounds the handling of global temperature data.145
Scientists should consider creating an independent discipline of Experimental Design, institutionalized in university departments and with its own professional association. This discipline, building upon and providing deeper theoretical grounding for existing instruction in experimental design146 and research methods,147 should include 1) the history of scientific epistemology;148 2) the theory of complex systems, which by their nature cannot easily be modeled;149 3) the theoretical underpinnings of statistics, emphasizing its limited capacity to reduce uncertainty;150 4) the theoretical rationale for data sharing and replication experiments, integrated with a survey of their institutional architecture;151 and 5) research methods courses and practica in experimental design and observational studies. Graduate students in all sciences and social sciences should be required to take a sequence of survey courses and practica in this discipline, and introductory courses should be required for all undergraduate science and social science majors.
Scientific journals should make their own peer review processes transparent to outside examination.152 Some journals should experiment with guaranteeing publication for research with pre-registered, peer-reviewed hypotheses, no matter the result.153 If the experiment is worth doing, it should be worth publishing. Ambitious researchers should no longer be forced into the position of Brian Wansink, who recycled his Italian restaurant data not least because he could not expect to publish a negative result.
Changing Scientific Culture
A NEW PROFESSIONALISM
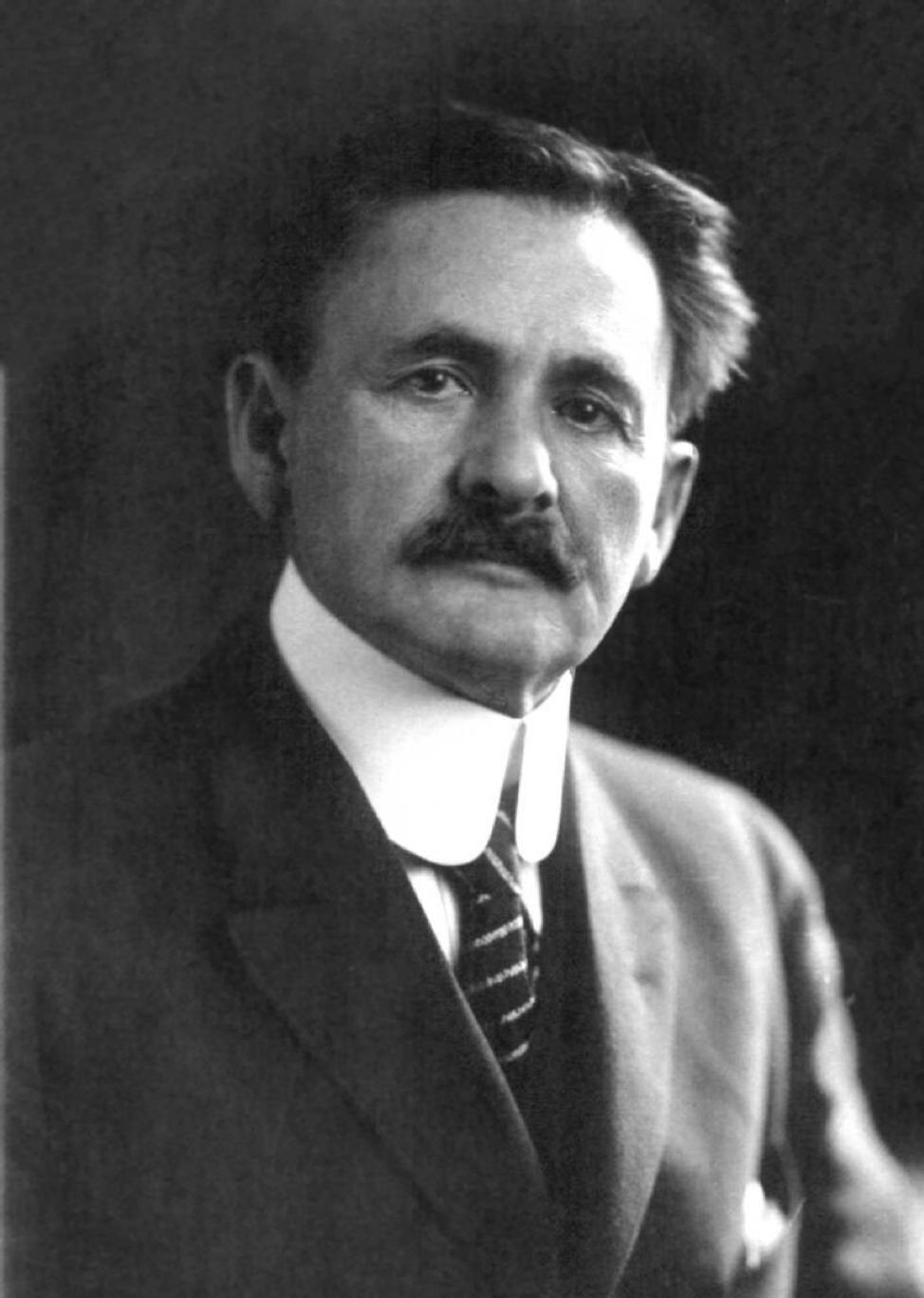
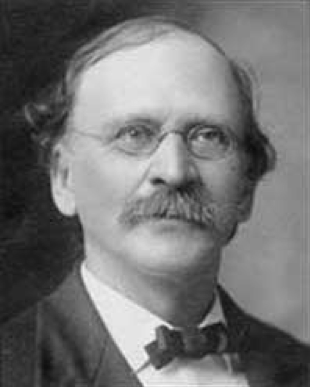
Scientists must reform the professional incentives that reward inadequate research and punish the unglamorous but essential work of checking research that has already been done. Researchers should perform more replication studies and accord greater esteem to research that produces negative results. Professional organizations, journals, and university tenure and promotion committees must all commit themselves to support these changes. Universities should tenure and promote researchers who adhere to strict methodological standards, not researchers who produce poorly grounded positive results that confirm professional prejudices. Foundations and government agencies that supply grants must also support this reformation of scientific culture by dedicating funding to scientists who seek to replicate earlier research. Foundations and government agencies should also dedicate major support to scientists who specialize in the development of better research methods.
Perhaps donors should fund university chairs in “reproducibility studies,” or establish an annual prize for the most significant negative results in various scientific fields. Such a prize might be called the Michelson-Morley Award, in honor of the invaluable negative results Albert Michelson and Edward Morley produced in 1887 in their attempt to determine the properties of “luminiferous ether”—a “failure” that eventually opened the door to Einstein’s special relativity and much of modern physics.154
Scientists will have a harder task as they tackle academic groupthink. Perhaps each discipline should institutionalize extradisciplinary critique, and establish committees staffed by professionals in other disciplines who routinely evaluate the intellectual openness of individual departments and the discipline as a whole. College and university administrations should guarantee that responsible dissenters from disciplinary orthodoxy can continue their careers.
But academics haven’t policed themselves well in the past, and they won’t likely do a good job in the future. The public outside the university must help transform modern science.
BEYOND THE UNIVERSITIES
Scientific industry—private corporations with a significant stake in scientific progress—must play a role in reforming the practices of their partners in academic science. Generally, industry needs to advocate for scientific practices that minimize irreproducible research, such as Transparency and Openness Promotion (TOP) guidelines for scientific journals. More concretely, industry needs to formulate, in conjunction with its academic partners, a set of research standards that will promote reproducible research—both for the good of science and for the good of its own bottom line.155
Yet the crisis of reproducibility goes well beyond the academic and industrial infrastructure that sustains the learned professions. It extends to our society as a whole. The crisis of science has proceeded as far as it has because the public rewards dubious science. It does so partly from ignorance, partly because it enjoys a steady diet of “new research” in the news, and partly because it likes the idea that science confirms popular prejudices. Society at large must also change its ways— not least because we depend so much for our well-being on the accuracy of scientific research, and our self-interest requires us to make the changes necessary to reform modern science.
Education reform will be the key. Science educators should integrate courses that impart a basic understanding of statistics into the nation’s high school and college curricula. Such courses would not require advanced mathematics, since students can understand the principles of statistical analysis without knowing how to derive the equation for a particular probability distribution. The courses should focus instead on the proper use and potential pitfalls of statistically-based research. In science courses generally, science educators should work to make students aware of both the characteristic vulnerabilities of modern science and the limits to the certainty that statistics can provide.
Government education policy should support these changes. State governments should reform high school curricula to include courses in statistics literacy, and use their funding and oversight powers over public universities to encourage university administrations to add statistical literacy requirements to their undergraduate curricula. The Federal government should also employ its funding and regulatory powers to encourage statistical literacy in primary, secondary, and postsecondary education.
Science journalists must also change the way they report. Too many science journalists simply reproduce press releases, which encourages researchers to pursue conclusions that produce an eye-grabbing headline. Science journalists rarely give as much attention to retractions or corrections of published research as they do to extreme and exciting new claims. In 2004, the media extensively publicized a claim by the Centers for Disease Control (CDC) that 400,000 Americans died from obesity each year.156 The media paid far less attention to the CDC’s later retraction when it discovered errors in its statistical methodology,157 and even fewer news outlets publicized the CDC researchers’ new estimate in 2005 that the number of annual deaths from obesity was only 112,000.158 Above all, science journalists have failed to make Americans aware of the reproducibility crisis itself. Most Americans don’t even know that the crisis exists.
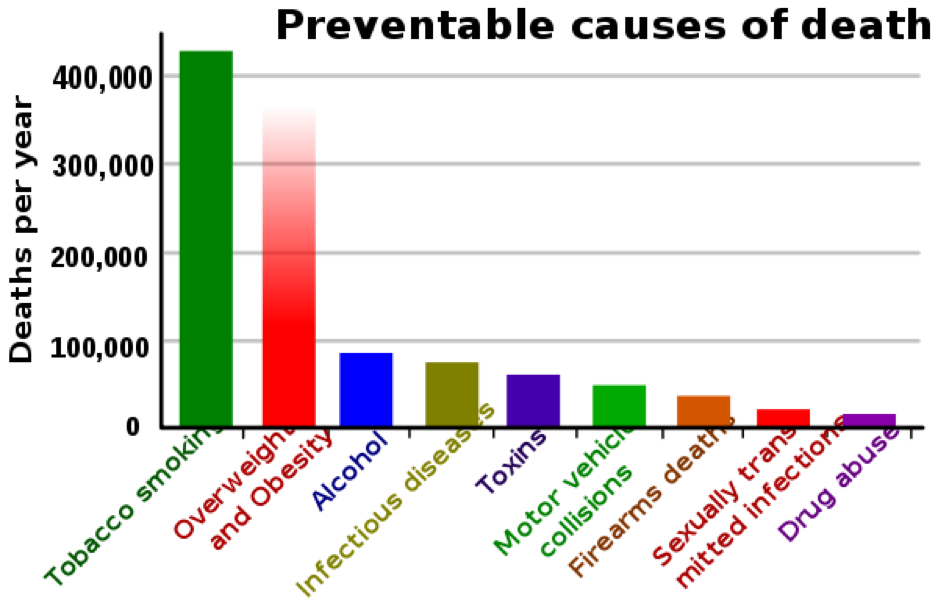
The eye-catching headline and the breathless lead will always tempt journalists. Nevertheless, science journalists should be more critical of new scientific studies. Reform of science journalism will reduce misleading popular coverage of scientific research—and thus significantly reduce the incentive to make bad science a stepping stone to fame.
Private foundations should support the reform of science journalism by funding continuing education of journalists into the scientific issues underlying the reproducibility crisis. The Medical Evidence Boot Camp, organized by the Knight Science Journalism Program at MIT, provides a good model for how foundations can help improve journalists’ coverage of science.159
Governmental Reforms
Government, which both funds and relies upon statistically-driven research, should also work to reform science. Government should spend more money on replication studies;160 prioritize grant funding for studies which pre-register their protocols and meet new best-practices standards; and require government-funded researchers to make their data and research protocols publicly available. While Federal agencies already have begun work on this front,161 government can further improve the practice of modern science swiftly and significantly by applying best existing government practices to every government agency that judges or relies upon scientific and social-scientific research. Since 2003, for example, the National Institutes of Health (NIH) has expected investigators “seeking $500,000 or more in direct costs in any single year … to include a plan for data sharing.” The NIH also supports archiving and sharing of methods and data via its support of the Immunology Database and Analysis Portal (ImmPort), and it encourages pre-registration of clinical trials via its support of the ClinicalTrials.gov website.162 It also recently redoubled its explicit emphasis on rigor and reproducibility in its granting process and its overall strategic plan.163
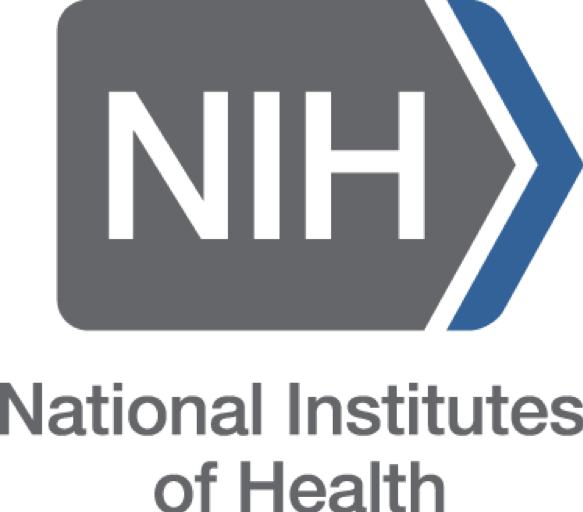
The NIH isn’t the only government agency which has started to address the crisis of reproducibility. The Office of Science and Technology Policy “has directed Federal agencies with more than $100M in R&D expenditures to develop plans to make the published results of federally funded research freely available to the public within one year of publication and requir[e] researchers to better account for and manage the digital data resulting from federally funded scientific research.”164 Still, the government’s response is only in its first stages. We recommend that other government agencies, especially the Environmental Protection Agency and the Department of Energy, adopt the NIH’s new standards.
Government, which both funds and relies upon statistically-driven research, should also work to reform science.
Implications for Policymaking
Dealing with the reproducibility crisis will involve doing more than just trying to reform the practice of science itself. The damage already done by irreproducible research will have to be repaired. Some of the most significant damage has been in the area of government policy, where legislation, regulation, and judicial precedent have sometimes been based on inadequate or dubious evidence.
Government Regulations
Federal, state, and local regulatory agencies should adopt strict reproducibility standards for assessing the science that informs the drafting of new regulations. No scientific research that fails to adhere to these reformed standards should be used to justify new regulations without legislative approval. Congress and state legislatures should also consider legislation to require regulatory agencies to adopt these standards. Both legislative and administrative policymakers should institute formal procedures to ensure that regulatory change bases itself solely on research that meets high standards of methodological transparency and statistical rigor.
Some progress is already being made in this direction. Congress is considering a Secret Science Reform Act to prohibit the Environmental Protection Agency (EPA) from “proposing, finalizing, or disseminating a covered action” unless the supporting research is “publicly available in a manner sufficient for independent analysis and substantial reproduction of research results.”165 This Act could be broadened to apply to a whole range of regulatory agencies within the Federal government.
The Federal government should also consider instituting review commissions for each regulatory agency to investigate whether existing regulations are based on well-grounded, reproducible research. These should establish the scope of the problem by identifying those regulations that rely on unreplicated or irreproducible research, and recommending which regulations should be revoked. Regulatory administrators or Congress should put these recommendations into practice by revoking all regulations that lack a proper scientific basis.
Policymakers should prioritize the review of those regulatory agencies with the greatest effect on the American economy and Americans’ individual lives. We recommend the earliest possible reproducibility assessment of regulations concerning climate change (Environmental Protection Agency (EPA), National Oceanic and Atmospheric Administration (NOAA)); air pollution (EPA); pharmaceuticals approval (Food and Drug Administration); biological effects of nuclear radiation (Department of Energy); the identification and assessment of learning disabilities (Department of Education); and dietary guidelines (United States Department of Agriculture (USDA)).
The Courts
Federal and state judiciaries should review their treatment of scientific and social-scientific evidence in light of the crisis of reproducibility. While judges generally have maintained a degree of skepticism toward scientists’ and social scientists’ claims to provide authoritative knowledge, such claims have influenced judicial decision-making, and have helped to weave the nation’s tapestry of controlling precedent.166 This development has proceeded despite the realization that judges must now distinguish between satisfactory and subpar research, even though they usually lack professional knowledge of the technical details of scientific practices.167
Judges should make future decisions with a heightened awareness that the crisis of reproducibility has produced a generation or more of presumptively unreliable research.168 More generally, the judiciary should adopt a standard set of principles for incorporating science into judicial decision-making, perhaps as binding precedent, that explicitly account for the crisis of reproducibility. They should also adopt a standard approach to overturning precedents based on irreproducible science. Finally, a commission of judges should recommend to law schools a required course on science and statistics as they pertain to the law, so as to educate future generations of lawyers and judges about the strengths and weaknesses of statistically-driven research. The commission should also recommend that each state incorporate a science and statistics course into its continuing legal education requirements for attorneys and judges.169
Legislative and Executive Staff
A democratic polity requires representatives who can address the large areas of policy affected by science and social science with informed knowledge of the strengths and weaknesses of the claims made in the name of these disciplines. Legislators who themselves lack specialized training in statistics and the sciences should give hiring preference to legislative assistants with training in these subjects. The employment of statistically proficient personnel will allow these legislators to oversee policymaking by the administrative bureaucracy, and to judge the scientific claims made in support of campaigns to introduce new legislation. Presidents and governors should also hire special assistants with equivalent training, in order to provide them a similar ability to exercise such judgment.
A Cautious Disposition
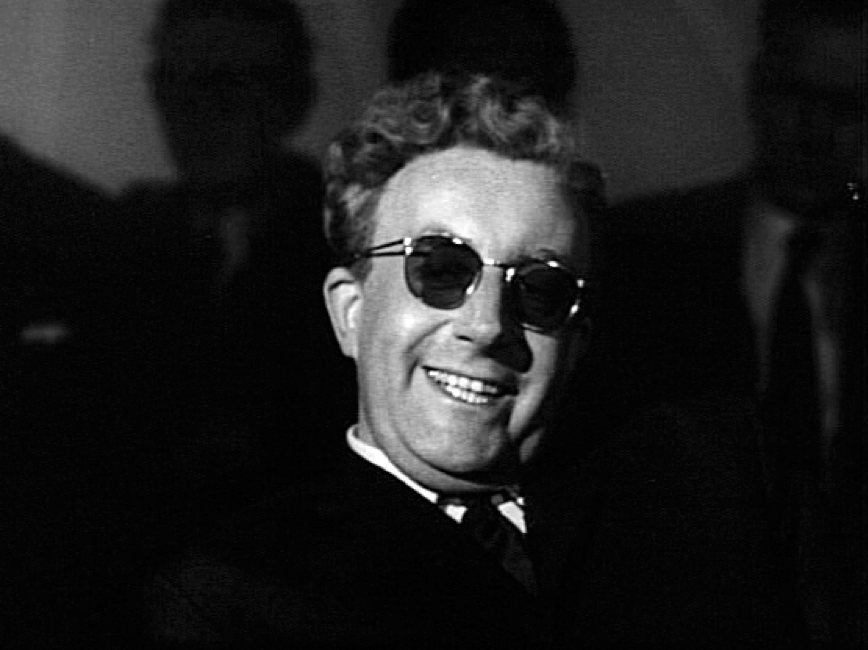
In general, legislators, judges, and bureaucrats should all look at scientific research with a warier eye. Science cannot speak with proper authority until it cleans house. Until then, responsible officials in government need not and should not automatically defer to scientists’ claims to expert knowledge. Responsible government officials should not make policy on the basis of irreproducible research.
Responsible government officials should not make policy on the basis of irreproducible research.
That rule comes with a caveat: not all research can be reproduced. Political science and economics, for example, study historical events—elections, recessions, and so on—that by their nature cannot be replicated. Politicians must continue to make policy informed by research that addresses itself to such unique circumstances. Yet they should be aware that such research, despite its merits, cannot claim the scientific authority of fully reproducible research. The authors of such research, in turn, should make policy recommendations that openly declare their research’s limited claims to scientific authority.
Transcending the Partisan Debate
The short-term thrust of these reforms may seem to favor the political agendas of American conservatives. Because many scientific and social-scientific disciplines now contain scarcely any conservatives, the combination of political groupthink with the rest of the crisis of reproducibility very likely has produced more irreproducible science that favors liberal policy. In consequence, reformed scientific standards probably will cull more science with liberal policy implications.
But reformed science isn’t “conservative” science. The implementation of new scientific protocols in pharmacology seems likely to diminish the number of test results that justify putting new drugs on the market, and therefore to reduce the profitability of several large pharmaceutical corporations—a real-world consequence that should please liberals who criticize corporate misconduct. Reformed standards may also favor other liberal policies in the end: scientists who worry about climate change have already begun to marshal crisis-of-reproducibility arguments to discredit their skeptical opponents.170 Science may be affected by liberal groupthink, but any scientist, of whatever political coloration, can rise above such limitations. After all, a great deal of the criticism of liberal groupthink in science comes from scientists who are themselves politically liberal,171 and conservative scientists are not immune to politicized groupthink. No political camp should be entirely pleased by the results of reformed scientific standards—and the reform of science will be carried on by scientists of every political persuasion. Whatever their political affiliation, all scientists and laymen who love truth more than partisan advantage should support scientific reform. Every American who cherishes the scientific pursuit of truth should seek to solve the problems that beset contemporary science.
Conclusion
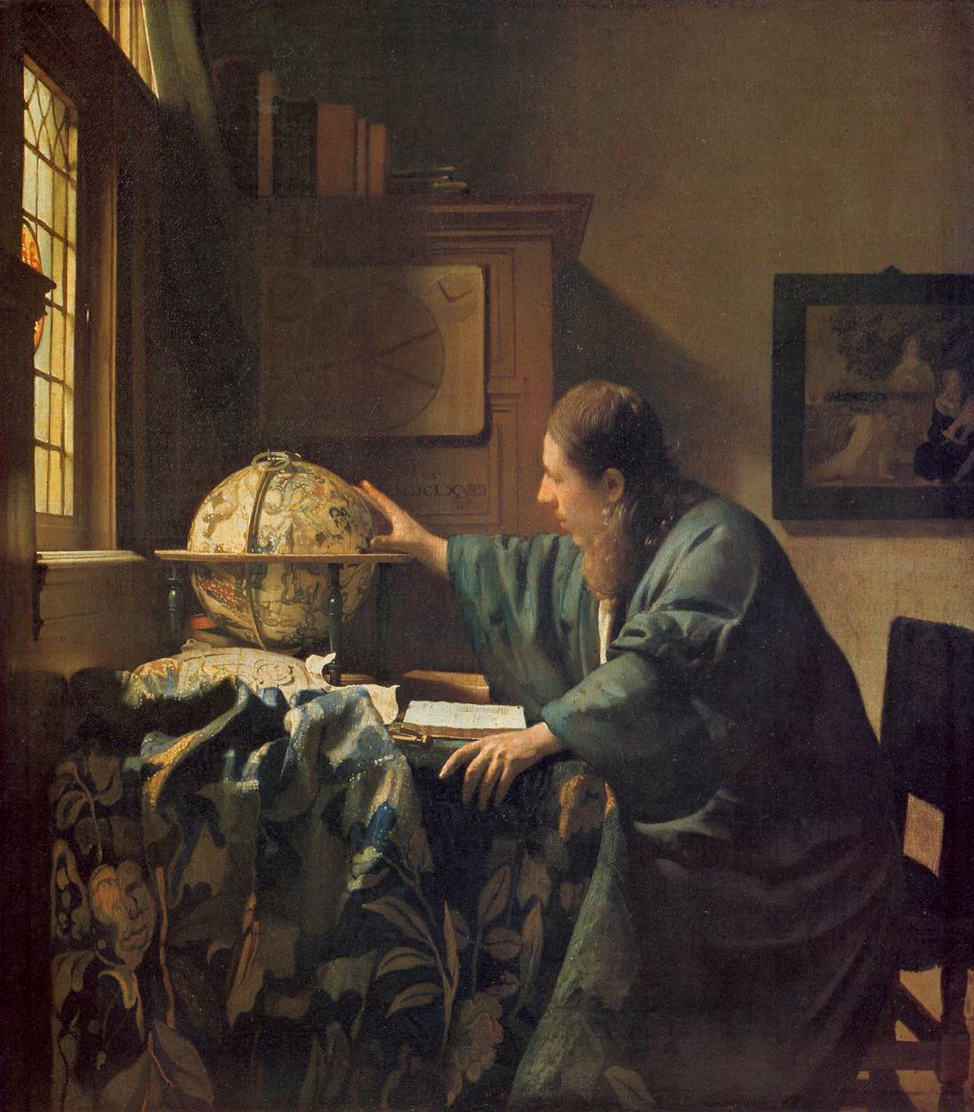
Simmons and his colleagues concluded their article on researcher freedom with an old truth that bears repetition: “Our goal as scientists is not to publish as many articles as we can, but to discover and disseminate truth.” But, as Simmons et al. acknowledge, too many scientists have lost sight of this goal.172 The foregoing recommendations would be good for science even if modern science were not in such urgent need of reform. But the existence of the irreproducibility crisis means that changes like the ones we suggest have become a matter of urgent necessity.
The battle against the present scourge of irreproducibility in science is not entirely new. Science has always imposed constraints on human nature in the service of truth. Empiricism, the obligation to gather data, forces scientists to submit their preconceptions to experimental proof. Rigorous precision, including the use of statistical methods, serves to check laziness and carelessness. Science’s struggle for empiricism and precision has always been fought against the all-too-human incentives to pursue predetermined conclusions, professional advancement—or both at once.
So the shortcomings of modern statistics-based research should not surprise us too much. Yet they have done great harm, and they undermine faith in the power and promise of science itself. We need new incentives, new institutional mechanisms, and a new awareness of all the ways in which science can go wrong.
The challenges daunt, but they should also exhilarate. We sometimes hear that professionals have thoroughly institutionalized science, and that its increasing sophistication means that it has become the province of credentialed technicians. The crisis of reproducibility shows that this is not so. The pursuit of scientific truth requires the public to scrutinize and critique the activity of scientific professionals, and to join with them to reform the practice of modern science.
Afterword by William Happer
David Randall and Christopher Welser have done a service by drawing attention to the flood of shoddy “science” that has flooded journals, conferences, and news releases in recent decades. This is a bigger problem than it used to be, although perhaps not on a per-scientist basis. We have many more scientists today than we used to.
Science has always had problems with quality control. Some particularly bizarre examples were given by Irving Langmuir in his classic lecture, “Pathological Science,”173 where he describes “N rays,” “Mitogenetic Rays,” etc. Langmuir gave a table that maps very well onto points made by Randall and Welser:
Symptoms of Pathological Science:
- The maximum effect that is observed is produced by a causative agent of barely detectable intensity, and the magnitude of the effect is substantially independent of the intensity of the cause.
- The effect is of a magnitude that remains close to the limit of detectability; or, many measurements are necessary because of the very low statistical significance of the results.
- Claims of great accuracy.
- Fantastic theories contrary to experience.
- Criticisms are met by ad hoc excuses thought up on the spur of the moment.
- Ratio of supporters to critics rises up to somewhere near 50% and then falls gradually to oblivion.
But Langmuir, a great scientist, was not immune to self-deception. As described in J. R. Fleming’s book, Fixing the Sky,174 Langmuir was convinced toward the end of his career that he and his colleagues had succeeded in controlling the weather by seeding clouds with silver iodide. Dispassionate reviews of his experiments showed no statistical evidence that they had affected the weather in any way. Langmuir, a good mathematician with a deep understanding of statistics, was fully capable of applying statistical tests himself. He did not do so. Training young scientists more rigorously in statistics may not help as much as we would like to alleviate the irreproducibility crisis.
As Randall and Welser make clear, young academic scientists are under tremendous pressure to publish. Often what they publish makes little sense, but it helps to ensure the next pay raise or promotion. Academic management, with its fixation on publications and citations, has exacerbated the irreproducibility crisis. But even in government and industry, the number of publications is often an important career determinant.
Science that touches on political agendas has contributed more than its share of problems to the irreproducibility crisis. For many years, researchers willing to demonize carbon dioxide, low-level radiation, meat products, etc., have benefited from generous funding by governments and virtue-signaling private foundations. Consider, for example, the list of harmful effects of carbon dioxide, published by “scientists,” much of it in peer-reviewed journals.175 Almost none of it is reproducible.
Many scientists think of themselves as philosopher kings, far superior to those in the “basket of deplorables.” The deplorables have a hard time understanding why scientists are so special, and why they should vote as instructed by them. More than two thousand years ago, Plato, who promoted the ideal of philosopher kings, also promoted the concept of the “noble lie,” a myth designed to persuade a skeptical population that they should be grateful to be ruled by philosopher kings.176 Our current scientific community has occasionally resorted to the noble lie, a problem that can’t be fixed by better training in statistics. Noble lies are also irreproducible and damage the credibility of science.
By eloquently drawing attention to the problem of reproducibility of “scientific” results, and by proposing ways to address the problem, Randall and Welser have done science a big favor.
William Happer is Cyrus Fogg Bracket Professor of Physics, Emeritus, at Princeton University and former Director of Energy Research of the US Department of Energy.
1 “Brian Wansink,” Charles H. Dyson School of Applied Economics and Management, Cornell University, https://dyson.cornell.edu/people/brian-wansink.
2 “The Large Plate Mistake,” Food & Brand Lab, Cornell University, https://foodpsychology.cornell.edu/discoveries/ large-plate-mistake.
3 “Glass Shape Illusions,” Food & Brand Lab, Cornell University, https://foodpsychology.cornell.edu/discoveries/ glass-shape-illusions.
4 “ABC News David Zinczenko talks with Brian Wansink about food behavior,” August 20, 2016, https://www.youtube. com/watch?v=-WuzPUMuZ6I.
5 “Is Your Kitchen Making You Fat? Decor + Organizing Tips to Help You Slim Down,” Rachael Ray Show, March 31, 2017, https://www.youtube.com/watch?v=8z6_nqECjRE.
6 Brian Wansink, “The Grad Student Who Never Said ‘No,’” Healthier & Happier, November 21, 2016, https://web.archive.org/web/20170312041524/http:/www.brianwansink.com
/phd-advice/the-grad-student-who- never-said-no.
7 “Backlash prompts prominent nutrition researcher to reanalyze multiple papers,” Retraction Watch, February 2, 2017, http://retractionwatch.com/2017/02/02/backlash-prompts-prominent-nutrition-researcher-reanalyze-multiple- papers/.
8 Ibid.
9 Andrew Gelman, “Hark, hark! the p-value at heaven’s gate sings,” Statistical Modeling, Causal Inference, and Social Science, December 15, 2016, http://andrewgelman.com/2016/12/15/hark-hark-p-value-heavens-gate-sings/.
10 “Backlash prompts prominent nutrition researcher to reanalyze multiple papers,” Retraction Watch, February 2, 2017, http://retractionwatch.com/2017/02/02/backlash-prompts-prominent-nutrition-researcher-reanalyze-multiple- papers/.
11 For an introduction to the terminology, see Wikipedia, “Reproducibility,” https://en.Wikipedia.org/wiki/ Reproducibility. For a more in-depth examination of the vocabulary, see Edo Pellizzari, et al., Reproducibility: A Primer on Semantics and Implications for Research (Research Triangle Park, NC, 2017), esp. pp. 9-11, 20-21, 25-40, https://www.rti.org/sites/default/files/resources/18127052_Reproducibility_
Primer.pdf.
12 Steven N. Goodman, Daniele Fanelli and John P. A. Ioannidis, “What does research reproducibility mean?,” Science Translational Medicine 8, 341 (2016), pp. 1-6, http://stm.sciencemag.org/content/8/341/341ps12/tab-pdf.
13 Markus Brückner and Mark Gradstein, “Income and Schooling,” Vox: CEPR’s Policy Portal, April 4, 2013, http:// voxeu.org/article/income-and-schooling.
14 Thomas L. Halton, et al., “Low-Carbohydrate-Diet Score and the Risk of Coronary Heart Disease in Women,” The New England Journal of Medicine 355 (2006), pp. 1991-2002, http://www.nejm.org/doi/full/10.1056/ NEJMoa055317#t=article.
15 Livio Raccuia, “Single-Target Implicit Association Tests (ST-IAT) Predict Voting Behavior of Decided and Undecided Voters in Swiss Referendums,” PLoS One (2016), http://journals.plos.org/plosone/article?id=10.1371/journal. pone.0163872.
16 For an introduction, see “Statistical epidemiology,” Wikipedia, https://en.Wikipedia.org/wiki/Statistical_ epidemiology. For more in-depth examinations, see David Clayton and Michael Hills, Statistical Models in Epidemiology (Oxford, 1993); M. Elizabeth Halloran and Donald Berry, eds., Statistical Models in Epidemiology, the Environment, and Clinical Trials (New York, 2000); Duncan C. Thomas, Statistical Methods in Genetic Epidemiology (Oxford, 2004); Lyle D. Broemeling, Bayesian Methods in Epidemiology (Boca Raton, FL, 2014).
17 For an introduction, see “Environmental statistics,” Wikipedia, https://en.Wikipedia.org/wiki/Environmental_ statistics. For more in-depth examinations, see Bryan F. J. Manly, Statistics for Environmental Science and Management, Second Edition (Boca Raton, FL, 2009); Pierre Legendre and Louis Legendre, Numerical Ecology, Third English Edition (Amsterdam, 2012); Song S. Qian, Environmental and Ecological Statistics with R, Second Edition (Boca Raton, FL, 2017).
18 For an introduction, see “Psychological Statistics,” Wikipedia, https://en.Wikipedia.org/wiki/Psychological_ statistics. For more in-depth examinations, see Michael Cowles, Statistics in Psychology: An Historical Perspective (London, 2005); Dieter Rasch, Klaus D. Kubinger, and Takuya Yanagida, Statistics in Psychology Using R and SPSS (Chichester, 2011); S. Alexander Haslam and Craig McGarty, Research Methods and Statistics in Psychology, Second Edition (London, 2014).
19 For an introduction, see Katarina Cobanovic and Valentina Sokolovska, “Use of statistical methods in sociology,” Proceedings of the Challenges for Analysis of the Economy, the Businesses, and Social Progress (2010), pp. 879- 92, http://www.eco.u-szeged.hu/download.php?docID=40429. For more in-depth examinations, see Adrian E. Raftery, “Statistics in Sociology, 1950-2000: A Selective Review,” Sociological Methodology 31 (2001), pp. 1-45, https://www.stat.washington.edu/raftery/Research/PDF/socmeth2001.pdf; John H. Goldthorpe, “Causation, Statistics, and Sociology,” European Sociological Review 17, 1 (2001), pp. 1-20, https://academic.oup.com/esr/article-abstract/17/1/1/502739?redirectedFrom=fulltext; Thomas J. Linneman, Social Statistics: The Basics and Beyond (New York, 2011).
20 Randall Munroe, xkcd, https://xkcd.com/882/.
21 Megan L. Head, et al., “The Extent and Consequences of P-Hacking in Science,” PLoS Biology (2015), http://journals.plos.org/plosbiology/article?id=10.1371/journal.pbio.1002106.
22 “Texas sharpshooter fallacy,” Wikipedia, https://en.Wikipedia.org/wiki/Texas_sharpshooter_fallacy. See also William C. Thompson, “Painting the target around the matching profile: the Texas sharpshooter fallacy inforensic DNA interpretation,” Law, Probability, and Risk 8 (2009), pp. 257-76, https://academic.oup.com/lpr/ article/8/3/257/926184.
23 “Backlash prompts prominent nutrition researcher to reanalyze multiple papers,” Retraction Watch, February 2, 2017, http://retractionwatch.com/2017/02/02/backlash-prompts-prominent-nutrition-researcher-reanalyze- multiple-papers/.
24 Tim van der Zee, et al., “Statistical heartburn: An attempt to digest four pizza publications from the Cornell Food and Brand Lab,” PeerJ Preprints, January 25, 2017, https://peerj.com/preprints/2748/; Tim van der Zee, “The Wanskink Dossier: An Overview,” The Skeptical Scientist, March 21, 2017, http://www.timvanderzee.com/the-wansink-dossier- an-overview/.
25 Tim van der Zee, “The Wanskink Dossier: An Overview,” The Skeptical Scientist, March 21, 2017, http://www. timvanderzee.com/the-wansink-dossier-an-overview/.
26 Andrew Gelman, “Pizzagate, or the curious incident of the researcher in response to people pointing out 150 errors in four of his papers,” Statistical Modeling, Causal Inference, and Social Science, February 3, 2017, http:// andrewgelman.com/2017/02/03/pizzagate-curious-incident-researcher-response-people-pointing-150-errors-four- papers-2/.
27 “Andrew Gelman,” Statistics Department, Columbia University, http://www.stat.columbia.edu/~gelman/.
28 “Applied Statistics Center,” Columbia University, http://iserp.columbia.edu/center/applied-statistics-center.
29 Andrew Gelman, “Pizzagate, or the curious incident of the researcher in response to people pointing out 150 errors in four of his papers,” Statistical Modeling, Causal Inference, and Social Science, February 3, 2017, http:// andrewgelman.com/2017/02/03/pizzagate-curious-incident-researcher-response-people-pointing-150-errors-four- papers-2/.
30 Andrew Gelman, “Dear Cornell University Public Relations Office,” Statistical Modeling, Causal Inference, and Social Science, April 6, 2017, http://andrewgelman.com/2017/04/06/dear-cornell-university-public-relations-office/.
31 Jordan Anaya, “Cornell and the First Law of Fooddynamics,” Medium, February 17, 2017, https://medium.com/@OmnesRes/cornell-and-the-first-law-of-fooddynamics-cb2ed34d7e7f; Nick Brown, “Some instances of apparent duplicate publication from the Cornell Food and Brand Lab,” Nick Brown’s blog, March 2, 2017, http://steamtraen. blogspot.co.uk/2017/03/some-instances-of-apparent-duplicate.html; Nick Brown, “Strange patterns in some results from the Food and Brand Lab,” Nick Brown’s blog, March 22, 2017, http://steamtraen.blogspot.co.uk/2017/03/strange-patterns-in-some-results-from.html.
32 John P. A. Ioannidis, “Why Most Published Research Findings Are False,” PLoS Med 2, 8 (2005), https://www.ncbi. nlm.nih.gov/pmc/articles/PMC1182327/.
33 See also Andrew Gelman and John Carlin, “Beyond Power Calculations: Assessing Type S (Sign) and Type M (Magnitude) Errors,” Perspectives on Psychological Science 9, 6 (2014), pp. 641-61, http://www.stat.columbia.edu/~gelman/research/published/retropower_final.pdf; Eric Loken and Andrew Gelman, “Measurement error and the replication crisis,” Science 355 (2017), pp. 584-85, http://science.sciencemag.org/content/355/6325/584; Nathan P. Lemoine, et al., “Underappreciated problems of low replication in ecological field studies,” Ecology, September 9, 2016, http://onlinelibrary.wiley.com/doi/10.1002/ecy.1506/abstract; Denes Szucs and John Ioannidis, “Empirical assessment of published effect sizes and power in the recent cognitive neuroscience and psychology literature,” PLoS Biology, March 2, 2017, http://journals.plos.org/plosbiology/article?id=10.1371/journal.pbio.2000797.
34 Leslie G. Biesecker, “Hypothesis-generating research and predictive medicine,” Genome Research 23, 7 (2013), pp. 1051-53, https://www.ncbi.nlm.nih.gov/pmc/articles/PMC3698497/.
35 John P. A. Ioannidis, “Contradicted and Initially Stronger Effects in Highly Cited Clinical Research,” JAMA 294, 2 (2005), pp. 218-28, http://jamanetwork.com/journals/jama/fullarticle/201218. See also John P. A. Ioannidis, “Why most discovered true associations are inflated,” Epidemiology 19, 5 (2008), pp. 640-48, https://www.ncbi.nlm.nih. gov/pubmed/18633328.
36 Shanil Ebrahim, et al., “Reanalyses of randomized clinical trial data,” JAMA 312, 10 (2014), pp. 1024-32, https:// www.ncbi.nlm.nih.gov/pubmed/25203082.
37 Further replication studies not mentioned in the text of this article that fail to reproduce much-publicized scientificresearch include Timothy C. Bates and Shivani Gupta, “Smart groups of smart people: Evidence for IQ as the origin of collective intelligence in the performance of human groups,” Intelligence 60 (2017), pp. 46-56, http:// www.sciencedirect.com/science/article/pii/S0160289616303282; John H. Lurquin, et al., “No Evidence of the Ego-Depletion Effect across Task Characteristics and Individual Differences: A Pre-Registered Study,” PLoS One, February 10, 2016, http://journals.plos.org/plosone/article?id=10.1371/journal.pone.0147770; E.-J. Wagenmakers, et al., “Registered Replication Report. Strack, Martin, & Stepper (1988) [Facial Feedback Hypothesis],” Perspectives on Psychological Science October 26, 2016, http://journals.sagepub.com/doi/full/10.1177/1745691616674458; Mark Regnerus, “Is structural stigma’s effect on the mortality of sexual minorities robust? A failure to replicate the results of a published study,” Social Science & Medicine 188 (2017) pp. 157-165, http://www.sciencedirect.com/science/ article/pii/S027795361630627X.
38 Andrew Gelman and Kaiser Fung, “The Power of the ‘Power Pose’,” Slate, January 19, 2016, http://www.slate.com/articles/health_and_science/science/2016/01/amy_cuddy
_s_power_pose_research_is_the_latest_example_of_scientific_overreach.html; Uri Simonsohn and Joseph Simmons, “Power Posing: Reassessing The Evidence Behind The Most Popular TED Talk,” Data Colada, May 8, 2015, http://datacolada.org/37.
39 Andrew Gelman and David Weakliem, “Of beauty, sex, and power: Statistical challenges in estimating small effects,” October 27, 2008, http://www.stat.columbia.edu/~gelman/research/unpublished/power4r.pdf.
40 Andrew Gelman, “A whole fleet of gremlins: Looking more carefully at Richard Tol’s twice-corrected paper, ‘TheEconomic Effects of Climate Change’,” Statistical Modeling, Causal Inference, and Social Science, May 27, 2014, http://andrewgelman.com/2014/05/27/whole-fleet-gremlins-looking-carefully-richard-tols-twice-corrected-paper-economic-effects-climate-change/.
41 Colleen M. Ganley, et al., “An examination of stereotype threat effects on girls’ mathematics performance,”Developmental Psychology 49, 10 (2013), pp. 1886-97, http://psycnet.apa.org/record/2013-02693-001; and see Ulrich Schimmack, “Why are Stereotype-Threat Effects on Women’s Math Performance Difficult to Replicate?,” Replicability-Index, January 6, 2015, https://replicationindex.wordpress.com/tag/stereotype-threat-and-womens- math-performance/.
42 Doug Rohrer, Harold Pashler, and Christine R. Harris, “Do Subtle Reminders of Money Change People’s Political Views?,” Journal of Experimental Psychology 144, 4 (2015), pp. e73-e85, http://uweb.cas.usf.edu/~drohrer/pdfs/ Rohrer_et_al_2015JEPG.pdf; Ulrich Schimmack, Moritz Heene, and Kamini Kesavan, “Reconstruction of a Train Wreck: How Priming Research Went off the Rails,” Replicability-Index, February 2, 2017, https://replicationindex. wordpress.com/2017/02/02/reconstruction-of-a-train-wreck-how-priming-research-went-of-the-rails/comment- page-1/, and see especially Daniel Kahneman, [Comment,] February 14, 2017, https://replicationindex.wordpress. com/2017/02/02/reconstruction-of-a-train-wreck-how-priming-research-went-of-the-rails/comment-page- 1/#comment-1454.
43 Yudhijit Bhattacharjee, “The Mind of a Con Man,” The New York Times, April 26, 2013, http://www.nytimes. com/2013/04/28/magazine/diederik-stapels-audacious-academic-fraud.html?_r=1&pagewanted=all&.
44 Rickard Carlsson and Jens Agerström, “A closer look at the discrimination outcomes in the IAT literature,” Scandinavian Journal of Psychology, April 24, 2016, http://onlinelibrary.wiley.com/doi/10.1111/sjop.12288/ abstract.
45 Hart Blanton, et al., “Toward a Meaningful Metric of Implicit Prejudice,” Journal of Applied Psychology 100, 5 (2015), pp. 1468-81, https://www.ncbi.nlm.nih.gov/pubmed/25602125.
46 Anthony Lane, et al., “Failed Replication of Oxytocin Effects on Trust: The Envelope Task Case,” PLoS One (2015), http://journals.plos.org/plosone/article?id=10.1371/journal.pone.0137000; Anthony Lane, et al., “Is there a Publication Bias in Behavioural Intranasal Oxytocin Research on Humans? Opening the File Drawer of One Laboratory” Journal of Neuroendocrinology 28, 4 (2016), http://onlinelibrary.wiley.com/doi/10.1111/jne.12384/abstract?campaign=wolacceptedarticle; Brian Resnick, “How scientists fell in and out of love with the hormone oxytocin,” Vox, April 4, 2016, https://www.vox.com/2016/4/4/11348288/oxytocin-love-hormone.
47 Gregory Francis, “Excess success for three related papers on racial bias,” Frontiers in Psychology 6, 512 (2015), https://www.ncbi.nlm.nih.gov/pmc/articles/PMC4416456/.
48 “Milgram experiment,” Wikipedia, https://en.Wikipedia.org/wiki/Milgram_experiment.
49 “Milgram experiment: Charges of data manipulation,” Wikipedia, https://en.Wikipedia.org/wiki/Milgram_experiment#
Charges_of_data_manipulation.
50 K. I. Mills, “More shocking results: New research replicates Milgram’s findings,” Monitor on Psychology (American Psychological Association) 40, 3 (2009), p. 13, http://www.apa.org/monitor/2009/03/milgram.aspx; Dariusz Dolinski, et al., “Would You Deliver an Electric Shock in 2015? Obedience in the Experimental Paradigm Developed by Stanley Milgram in the 50 Years Following the Original Studies,” Social Psychological and Personality Science, March 14, 2017, http://journals.sagepub.com/doi/10.1177/1948550617693060.
51 Ronald Bailey, “Most Scientific Findings are Wrong or Useless,” Reason.com, August 26, 2016, http://reason.com/archives/2016/08/26/most-scientific-results-are-wrong-or-use.
52 C. Glenn Begley and Lee M. Ellis, “Drug development: Raise standards for preclinical cancer research,” Nature 483 (2012), pp. 531-33, http://www.nature.com/nature/journal/v483/n7391/full/483531a.html?foxtrotcallback=true.
53 Edward R. Dougherty, “Biomarker Development: Prudence, risk, and reproducibility,” Bioessays 34 (2012), pp. 277- 79, esp. p. 279, http://onlinelibrary.wiley.com/doi/10.1002/bies.201200003/abstract.
54 Open Science Collaboration [Brian Nosek, et al.], “Estimating the reproducibility of psychological science,” Science 349 (2015), http://science.sciencemag.org/content/349/6251/aac4716.
55 Andrew C. Chang and Phillip Li, “Is Economics Research Replicable? Sixty Published Papers from Thirteen Journals Say ‘Usually Not’,” Finance and Economics Discussion Series 2015-083 (2015), https://www.federalreserve.gov/econresdata/feds/2015/files/2015083pap.pdf.
56 Colin F. Camerer, et al., “Evaluating replicability of laboratory experiments in economics,” Science 351 (2016), pp. 1433-36, http://science.sciencemag.org/content/351/6280/1433.
57 Megan L. Head, et al., “The Extent and Consequences of P-Hacking in Science,” PLoS Biology 13, 3 (2015), http:// journals.plos.org/plosbiology/articleid=10.1371/journal.pbio.1002106.
58 Stephan B. Bruns and John P. A. Ioannidis, “p-Curve and p-Hacking in Observational Research,” PLoS One, February 17, 2016, http://journals.plos.org/plosone/article?id=10.1371/journal.pone.0149144.
59 C. H. J. Hartgerink, “Reanalyzing Head et al. (2015): No widespread p-hacking after all?,” PeerJPreprints, September 12, 2016, https://www.authorea.com/users/2013/articles/31568-reanalyzing-head-et-al-2015-no-widespread-p- hacking-after-all/_show_article.
60 Sean Tanner, “Evidence of False Positives in Research Clearinghouses and Influential Journals: An Application of P-Curve to Policy Research,” 2015, https://gspp.berkeley.edu/assets/uploads/research/pdf/Tanner_p-curve_ paper_v2.0.pdf.
61 Jonathan A. C. Sterne and George Davey Smith, “Sifting the evidence—what’s wrong with significance tests?,” BMJ 322 (2001), pp. 226-31, https://www.ncbi.nlm.nih.gov/pmc/articles/PMC1119478/.
62 American Statistical Association, “ASA Statement on Statistical Significance and P-Values,”The American Statistician 70, 2 (2016), pp. 131-33, http://amstat.tandfonline.com/doi/full/10.1080/00031305.2016.1154108scroll=top
&needAccess=true#aHR0cDovL2Ftc3RhdC50YW5kZm9ubGluZS5jb20vZG9pL3BkZi8
xMC4xMDgwLzAwMDMxMzA1LjIwMTYuMTE1NDEwOD9uZWVkQWNjZX NzPXRydWVAQEAw. See also Steven Goodman, “A Dirty Dozen: Twelve P-Value Misconceptions,” Seminars in Hematology 45, 3 (2008), pp. 135-40, https://www.ncbi.nlm.nih.gov/pubmed/18582619.
63 Emili García-Berthou and Carles Alcarez, “Incongruence between test statistics and P values in medical papers,” BMC Medical Research Methodology 4, 13 (2004), https://www.ncbi.nlm.nih.gov/pubmedcmd=Search&doptcmdl=Citation&default
Field=Title%20Word&term=GarciaBerthou%5Bauthor%5D%20AND%20Incongruence
%20between%20test%20statistics%20and%20P%20values%20in%20medical%20papers; Michèle B. Nuijten, et al., “The prevalence of statistical reporting errors in psychology (1985–2013),” Behavior Research Methods 48, 4 (2016), pp. 1205-26, https://link.springer.com/article/10.3758%2Fs13428-015-0664-2.
64 Matthew H. Kramer, et al., “Statistics in a Horticultural Journal: Problems and Solutions,” Journal of the American Society for Horticultural Science 141, 5 (2016), pp. 400-06, http://journal.ashspublications.org/content/141/5/400.full; Tracey L. Weissberger, et al., “Reinventing Biostatistics Education for Basic Scientists,” PLoS Biology 14, 6 (2016), http://journals.plos.org/plosbiology/article?id=10.1371/journal.pbio.1002430.
65 Jeff Leek, “On the scalability of statistical procedures: why the p-value bashers just don’t get it,” Simply Statistics, February 14, 2014, https://simplystatistics.org/2014/02/14/on-the-scalability-of-statistical-procedures-why-the-p- value-bashers-just-dont-get-it/.
66 E.g., Thea F. van de Mortel, “Faking it: social desirability response bias in self-report research,” Australian Journal of Advanced Nursing 25, 4 (2008), pp. 40-48, http://citeseerx.ist.psu.edu/viewdoc/download?doi=10.1.1.818.5855&rep=rep1&type=pdf; and see Rob Hoskin, “The dangers of self-report,” Science for All: Brainwaves, March 3, 2012, http://www.sciencebrainwaves.com/the-dangers-of-self-report/.
67 Leslie K. John, et al., “Measuring the Prevalence of Questionable Research Practices With Incentives for Truth Telling,” Psychological Science 23, 5 (2012), pp. 524-32, https://www.cmu.edu/dietrich/sds/docs/loewenstein/ MeasPrevalQuestTruthTelling.pdf.
68 Sam Schwarzkopf, “The Pipedream of Preregistration,” The Devil’s Neuroscientist, November 28, 2014, https://devilsneuroscientist.wordpress.com/2014/11/28/the-pipedream-of-preregistration/.
69 Leslie K. John, et al., “Measuring the Prevalence of Questionable Research Practices With Incentives for Truth Telling,” Psychological Science 23, 5 (2012), pp. 524-32, https://www.cmu.edu/dietrich/sds/docs/loewenstein/ MeasPrevalQuestTruthTelling.pdf.
70 Claudia Tebaldi and Reto Knutti, “The use of the multi-model ensemble in probabilistic climate projections,”Philosophical Transactions of the Royal Society 365 (2007), pp. 2053-75, esp. p. 2068, http://rsta. royalsocietypublishing.org/content/365/1857/2053. See also Frédéric Hourdin, et al., “The Art and Science of Climate Model Tuning,” Bulletin of the American Meteorological Society, March 2017, pp. 589-602, https://journals. ametsoc.org/doi/pdf/10.1175/BAMS-D-15-00135.1.
71 Andrew Gelman and Eric Loken, “The Statistical Crisis in Science,” American Scientist 102 (2014), pp. 460-65, https://pdfs.semanticscholar.org/922b/5cffa298ad5e109acf7dbe6b7bcceb5740b4.pdf.
72 Alec T. Beall and Jessica L. Tracy, “Women Are More Likely to Wear Red or Pink at Peak Fertility,” Psychological Science 24, 9 (2013), pp. 1837-41, https://pdfs.semanticscholar.org/922b/5cffa298ad5e109acf7dbe6b7bcceb 5740b4.pdf.
73 Andrew Gelman and Eric Loken, “The Statistical Crisis in Science,” American Scientist 102 (2014), pp. 463-64, https://pdfs.semanticscholar.org/922b/5cffa298ad5e109acf7dbe6b7bcceb5740b4.pdf.
74 Joseph P. Simmons, et al., “False-Positive Psychology: Undisclosed Flexibility in Data Collection and Analysis Allows Presenting Anything as Significant,” Psychological Science 22, 11 (2011), pp. 1359-66, http://people.psych.cornell. edu/~jec7/pcd%202015-16%20pubs/Simmons%20PsySci%202011.pdf.
75 Helena Harrison, et al., “Case Study Research: Foundations and Methodological Orientations,” Forum: Qualitative Social Research 18, 1 (2017), http://www.qualitative-research.net/index.php/fqs/article/view/2655/4079.
76 John Whitehead, “Stopping clinical trials by design,” Nature Reviews Drug Discovery 3 (2004), pp. 973-77, https://www.nature.com/nrd/journal/v3/n11/full/nrd1553.html.
77 Steven E. Nissen, “ADAPT: The Wrong Way to Stop a Clinical Trial,” PLoS Clinical Trials 1, 7 (2006), http://journals.plos.org/plosclinicaltrials/articleid=10.1371/journal.pctr.0010035.
78 Paul S. Mueller, et al., “Ethical Issues in Stopping Randomized Trials Early Because of Apparent Benefit,” Annals of Internal Medicine 146, 12 (2007), pp. 878-81, http://annals.org/aim/article/735073/ethical-issues-stopping-randomized-trials-early-because-apparent-benefit.
79 Mihaela Stegert, et al., “An analysis of protocols and publications suggested that most discontinuations of clinical trials were not based on preplanned interim analyses or stopping rules,” Journal of Clinical Epidemiology (2015), https://fhs.mcmaster.ca/anesthesiaresearch/documents/PIIS089543561500267X.pdf.
80 Joseph P. Simmons, et al., “False-Positive Psychology: Undisclosed Flexibility in Data Collection and Analysis Allows Presenting Anything as Significant,” Psychological Science 22, 11 (2011), pp. 1359-66, http://journals.sagepub.com/doi/pdf/10.1177/0956797611417632.
81 Lauren E. Griffith, et al., “Statistical approaches to harmonize data on cognitive measures in systematic reviews are rarely reported,” Journal of Clinical Epidemiology 68, 2 (2015), pp. 154-62, http://www.jclinepi.com/article/S0895- 4356(14)00349-7/fulltext. For ontologically grounded remedies to provide standardized descriptions of research methods across the disciplines, see Robert Arp, Barry Smith, and Andrew D. Spear, Building Ontologies with Basic Formal Ontology (Cambridge, MA and London: 2015).
82 D. Fleischer, et al., “The implementation of initial data populations of environmental data and creation of a primary working database,” Polar Science 6, 1 (2012), pp. 97-103, http://www.sciencedirect.com/science/article/pii/S1873965212000023.
83 John Bates, “Climate scientists versus climate data,” Climate Etc., February 4, 2017, https://judithcurry.com/2017/02/04/climate-scientists-versus-climate-data/.
84 Thomas R. Karl, et al., “Possible artifacts of data biases in the recent global surface warming hiatus,” Science 348 (2015), pp. 1469-72, http://science.sciencemag.org/content/348/6242/1469.
85 Chris Mooney, “Federal scientists say there never was any global warming ‘pause’,” The Washington Post, June 4, 2015, https://www.washingtonpost.com/news/energy-environment/wp/2015/06/04/federal-scientists-say-there- never-was-any-global-warming-slowdown/?utm_term=.ffa38eaca77d.
86 Andrew Gelman, “Pizzagate, or the curious incident of the researcher in response to people pointing out 150 errors in four of his papers,” Statistical Modeling, Causal Inference, and Social Science, February 3, 2017, http:// andrewgelman.com/2017/02/03/pizzagate-curious-incident-researcher-response-people-pointing-150-errors-four- papers-2/.
87 Kay Dickersin, et al., “Publication Bias and Clinical Trials,” Controlled Clinical Trials 8, 4 (1987), pp. 343-53, http://www.sciencedirect.com/science/article/pii/0197245687901553.
88 Annie Franco, et al., “Publication bias in the social sciences: Unlocking the file drawer,” Science 345 (2014), pp. 1502- 05, http://science.sciencemag.org/content/345/6203/1502.
89 Patrick J. Michaels, “Evidence for “publication Bias” Concerning Global Warming in Science and Nature,” Energy & Environment 19, 2 (2008), pp. 287-301, http://journals.sagepub.com/doi/ abs/10.1260/095830508783900735?journalCode=eaea.
90 Anton Kühberger, et al., “Publication Bias in Psychology: A Diagnosis Based on the Correlation between Effect Size and Sample Size,” PLoS One 9, 9 (2014), http://journals.plos.org/plosone/article?id=10.1371/journal.pone.0105825.
91 Alan S. Gerber and Neil Malhotra, “Publication Bias in Empirical Sociological Research Do Arbitrary Significance Levels Distort Published Results?,” Sociological Methods and Research 37, 1 (2008), pp. 3-30, http://journals.sagepub.com/doi/abs/10.1177/0049124108318973.
92 Jim McCambridge, “A case study of publication bias in an influential series of reviews of drug education,” Drug and Alcohol Review 26, 5 (2007), pp. 463-68, https://www.ncbi.nlm.nih.gov/pubmed/17701508.
93 Leping Liu, et al., “An Examination of Publication Bias in an International Journal of Information Technology in Education,” Computers in the Schools 24, 1-2 (2007), pp. 145-63, https://eric.ed.gov/?id=EJ783492.
94 Stephanie Coronado-Montoya, et al., “Reporting of Positive Results in Randomized Controlled Trials of Mindfulness-Based Mental Health Interventions,” PLoS One 11, 4 (2016), http://journals.plos.org/plosone/article?id=10.1371/journal.pone.0153220.
95 John M. Crawford, et al., “Publication Bias and Its Implications for Evidence-Based Clinical Decision Making,” Journal of Dental Education 74, 6 (2010), pp. 593-600, http://www.jdentaled.org/content/74/6/593.full.
96 Irving L. Janis, Groupthink: Psychological Studies of Policy Decisions and Fiascoes (Boston: Houghton Mifflin,1982), p. 1. See also Lee Ross, Mark Lepper, and Michael Hubbard, “Perseverance in self-perception and social perception: biased attributional processes in the debriefing paradigm,” Journal of Personality and Social Psychology 32, 5 (1975), pp. 880-92, https://www.ncbi.nlm.nih.gov/pubmed/1185517.
97 Daniel B. Klein and Charlotta Stern, “Groupthink in Academia: Majoritarian Departmental Politics and the Professional Pyramid,” The Independent Review 13, 4 (2009), http://www.independent.org/publications/tir/article.asp?a=731.
98 “Ignaz Semmelweis,” Wikipedia, https://en.Wikipedia.org/wiki/Ignaz_Semmelweis.
99 Ian Leslie, “The sugar conspiracy,” The Guardian, April 7, 2016, https://www.theguardian.com/society/2016/apr/07/the-sugar-conspiracy-robert-lustig-john-yudkin.
100 Stanley Rothman, et al., “Politics and Professional Advancement Among College Faculty,” The Forum 3, 1 (2005), http://www.conservativecriminology.com/uploads/5/6/1/7/56173731/
rothman_et_al.pdf; Scott Jaschik, “Moving Further to the Left,” Inside Higher Ed, October 24, 2012, https://www.insidehighered.com/news/2012/10/24/survey-finds-professors-already-liberal-have-moved-further-left; Christopher Ingraham, “The dramatic shift among college professors that’s hurting students’ education,” The Washington Post, January 11, 2016, https://www.washingtonpost.com/news/wonk/wp/2016/01/11/the-dramatic-shift-among-college-professors-thats-hurting- students-education/?utm_term=.3dd925610e0f; Mitchell Langbert, et al., “Faculty Voter Registration in Economics, History, Journalism, Law, and Psychology,” Econ Journal Watch 13, 3 (2016), pp. 422-51, https://econjwatch.org/file_download/944/LangbertQuainKleinSept2016.pdf?mimetype=pdf.
101 John Tierney, “Social Scientist Sees Bias Within,” The New York Times, January 7, 2011, http://www.nytimes. com/2011/02/08/science/08tier.html?_r=0.
102 Heterodox Academy: “The Problem,” https://heterodoxacademy.org/the-problem/; “Research,” https:// heterodoxacademy.org/research/; “Political Bias,” Best Practices in Science, http://bps.stanford.edu/?page_id=3371; “Publications,” Heterodox Academy, https://web.archive.org/web/20170218102047/http://heterodoxacademy.org/
resources/publications/.
103 Andrew Gelman, “‘Why this gun control study might be too good to be true’,” Statistical Modeling, Causal Inference, and Social Science, March 11, 2016, http://andrewgelman.com/2016/03/11/why-this-gun-control-study-might-be-too-good-to-be-true/.
104 José L. Duarte, et al., “Political diversity will improve social psychological science” Behavioral and Brain Sciences 38 (2015), https://www.cambridge.org/core/journals/behavioral-and-brain-sciences/article/political-diversity-will-improve-social-psychological-science1/A54AD4878AED1AFC8BA6AF54A890149F; Alice H. Eagly, “When Passionate Advocates Meet Research on Diversity, Does the Honest Broker Stand a Chance?,” Journal of Social Issues 72, 1 (2016), pp. 199-222, http://onlinelibrary.wiley.com/doi/10.1111/josi.12163/abstract; Mark Regnerus, “How different are the adult children of parents who have same-sex relationships? Findings from the New Family Structures Study,” Social Science Research 41, 4 (2012), pp. 752-70, http://www.sciencedirect.com/science/article/ pii/S0049089X12000610; Richard E. Redding, “Scientific Groupthink and Gay Parenting,” The American (AEI), December 18, 2013, http://www.aei.org/publication/scientific-groupthink-and-gay-parenting/;
105 Judith A. Curry, “Statement to the Committee of Science, Space and Technology of the United States House of Representatives,” March 29, 2017, https://curryja.files.wordpress.com/2017/03/curry-house-science-testimony-mar-17.pdf.
106 Judith Curry, et al., Climate Science: Assumptions, policy implications, and the scientific method (The Global Warming Policy Foundation: GWPF Report 24, 2017), https://www.thegwpf.org/content/uploads/2017/03/Climate- Science-March20171.pdf: Judith Curry, “Testimony of Professor Judith Curry,” p. 13. Also see Scott Adams, Dilbert, May 14, 2017, http://dilbert.com/strip/2017-05-14.
107 Leonard P. Freedman, et al., “The Economics of Reproducibility in Preclinical Research,” PLoS Biology 13, 6 (2015), http://journals.plos.org/plosbiology/article?id=10.1371/journal.pbio.1002165; and see Richard Harris, Rigor Mortis: How Sloppy Science Creates Worthless Cures, Crushes Hopes, and Wastes Billions (New York: Basic Books, 2017).
108 Monya Baker, “1,500 scientists lift the lid on reproducibility,” Science, May 25, 2016, http://www.nature.com/news/1-500-scientists-lift-the-lid-on-reproducibility-1.19970?WT.mc_id=SFB_NNEWS_1508_RHBox.
109 [“Home Page,”] The Center for Open Science, https://cos.io/; [“Home Page,”] Laura and John Arnold Foundation, http://www.arnoldfoundation.org/.
110 “Reproducibility Project: Psychology,” OSFHome, [Open Science Framework,] https://osf.io/ezcuj/.
111 “Reproducibility Project: Cancer Biology,” eLife, https://elifesciences.org/collections/9b1e83d1/reproducibility-project-cancer-biology.
112 Preregistration Challenge, The Center for Open Science, https://cos.io/prereg/.
113 Sam Apple, “John Arnold made a fortune at Enron. Now he’s declared war on bad science,” Wired, January 22, 2017, https://www.wired.com/2017/01/john-arnold-waging-war-on-bad-science/.
114 “Research Integrity,” Laura and John Arnold Foundation, http://www.arnoldfoundation.org/.
115 [“Home Page,”] Meta-Research Innovation Center at Stanford (METRICS), http://metrics.stanford.edu/.
116 Holly Else, “Journals’ statistics rules ‘help tackle reproducibility crisis’,” Times Higher Education, May 29, 2017,
117 David Giofrè, et al., “The influence of journal submission guidelines on authors’ reporting of statistics and use of open research practices,” PLoS One 12, 4 (2017), http://journals.plos.org/plosone/article?id=10.1371/journal. pone.0175583.
118 The All Results Journals, http://arjournals.com/.
119 Journal of Articles in Support of the Null Hypothesis, http://www.jasnh.com/.
120 Journal of Pharmaceutical Negative Results, http://www.pnrjournal.com/.
121 Journal of Negative Results in BioMedicine, https://jnrbm.biomedcentral.com/.
122 Journal of Negative Results, http://www.jnr-eeb.org/index.php/jnr.
123 International Journal for Re-Views in Empirical Economics, https://www.iree.eu/.
124 Michael Heise, “ELS Replication Conference--Call For Proposals--Deadline Extension,” October 19, 2017, Empirical Legal Studies, http://www.elsblog.org/the_empirical_legal_studi/conferences/.
125 “WHO Statement on Public Disclosure of Clinical Trial Results,” World Health Organization, April 9, 2015, http://www.who.int/ictrp/results/reporting/en/.
126 Andrew Gelman, “Why Does the Replication Crisis Seem Worse in Psychology?,” Slate, October 3, 2016, http://www.slate.com/articles/health_and_science/science/2016/10/why_the_
replication_crisis_seems_worse_in_ psychology.html. See also Geoff Cumming, “The New Statistics: Why and How,” Psychological Science 25, 1 (2014), pp. 7-29, http://journals.sagepub.com/doi/10.1177/0956797613504966.
127 The National Academies of Sciences, Engineering, and Medicine Fostering Integrity in Research (Washington, DC: The National Academies Press, 2017), p. 5, https://www.nap.edu/read/21896/chapter/2#5.
128 Marcus R. Munafò, et al., “A manifesto for reproducible science,” Nature Human Behavior 1 (2017), https://www.nature.com/articles/s41562-016-0021.
129 Roger Peng, “The reproducibility crisis in science: A statistical counterattack,” Significance, June 2015, pp. 30-32, http://onlinelibrary.wiley.com/doi/10.1111/j.1740-9713.2015.00827.x/epdf.
130 American Statistical Association, “ASA Statement on Statistical Significance and P-Values,” The American Statistician 70, 2 (2016), pp. 131-33, http://amstat.tandfonline.com/doi/full/10.1080/00031305.2016.1154108?scroll=top&needAccess=true#aHR0cDovL2Ftc3RhdC50YW5kZm9ubGluZS5jb20vZG9p L3BkZi8xMC4xMDgwLzAwMDMxMzA1LjIwMTYuM TE1NDEwOD9uZWVkQWNjZXNzPXRydWVAQEAw.
131 Daniel J. Benjamin, et al., “Redefine statistical significance,” Nature Human Behavior (2017), https://www.nature. com/articles/s41562-017-0189-z. But see Blakeley B. McShane, et al., “Abandon Statistical Significance,” September 21, 2017, http://www.stat.columbia.edu/~gelman/research/unpublished/abandon.pdf.
132 For critiques of the use of p-values, see William M. Briggs, “The Substitute for p-Values,” Journal of the American Statistical Association 112, 519 (2017), pp. 897-98, http://www.tandfonline.com/doi/full/10.1080/01621459.2017.1311264; David Trafimow, et al., “Manipulating the Alpha Level Cannot Cure Significance Testing: Comments on ‘Redefine Statistical Significance’,” Peer J Preprints (2017), https://www.researchgate.net/publication/321071615_Manipulating_the_alpha
_level_cannot_cure_significance_testing_-_comments_on_Redefine_statistical_significance.
133 David Trafimow and Michael Marks, “Editorial,” Basic and Applied Social Pyschology 37, 1-2 (2015), pp. 1-2, http://www.medicine.mcgill.ca/epidemiology/Joseph/courses/EPIB-621/BASP2015.pdf.
134 Rink Hoekstra, et al., “Robust misinterpretation of confidence intervals,” Psychonomic Bulletin & Review 21, 5 (2014), pp. 1157-64, http://www.ejwagenmakers.com/inpress/HoekstraEtAlPBR.pdf.
135 “Bayesian Probability,” Wikipedia, https://en.Wikipedia.org/wiki/Bayesian_probability; Peter M. Lee, Bayesian Statistics: An Introduction, Fourth Edition (Chichester, 2012).
136 For an alternate view, see Andrew Gelman and Cosma Rohilla Shalizi, “Philosophy and the practice of Bayesian statistics,” British Journal of Mathematics and Statistical Psychology 66 (2013), pp. 8-38, http://www.stat. columbia.edu/~gelman/research/published/philosophy.pdf.
137 “Bayes’ theorem,” Wikipedia, https://en.Wikipedia.org/wiki/Bayes%27_theorem.
138 “Conviction in Son’s Death Overturned; Court Criticizes Gansler’s Use of SIDS Statistics in Insurance Argument,” Barry H. Helfand & David Martella, https://www.maryland-defense-attorneys.com/conviction-in-sons-death-overturned-court-criticizes-ganslers-us.html.
139 Vincent Scheurer, “Convicted on Statistics?,” Understanding Uncertainty, https://understandinguncertainty.org/node/545.
140 In Maryland, the accused parent was later reconvicted of murder, but the prosecution did not use statistics in the second trial. David Snyder, “Md. Father Convicted Again of Smothering Infant Son,” The Washington Post, May 14, 2004, https://www.washingtonpost.com/archive/local/2004/05/14/md-father-convicted-again-of-smothering- infant-son/c1e55507-002c-4b77-b0af-80dbf1666726/?utm_term=.099084bf64b2.
141 E.g., Sean Tanner, “Evidence of False Positives in Research Clearinghouses and Influential Journals: An Application of P-Curve to Policy Research,” Observational Studies 1 (2015), pp. 18-29, http://obsstudies.org/files/pcurve_protocol.pdf.
142 Joseph P. Simmons, et al., “False-Positive Psychology: Undisclosed Flexibility in Data Collection and Analysis Allows Presenting Anything as Significant,” Psychological Science 22, 11 (2011), pp. 1359-66, http://journals.sagepub.com/doi/pdf/10.1177/0956797611417632.
143 Scientists should consider employing the standardized descriptions of research materials and procedures provided by the theory of applied ontology. Robert Arp, Barry Smith, and Andrew D. Spear, Building Ontologies with Basic Formal Ontology (Cambridge, MA and London: 2015); Nophar Geifman, et al., “Opening clinical trial data: are the voluntary data-sharing portals enough?” BMC Medicine 13, 280 (2015), https://www.researchgate.net/publication/283748831_Opening_clinical_trial_data
_Are_the_voluntary_data-sharing_portals_enough; Anita Bandrowski, et al., “The Ontology for Biomedical Investigations,” PLoS One, April 29, 2016, http://journals.plos.org/ plosone/article?id=10.1371/journal.pone.0154556; and see Satya S. Sahoo, Joshua Valdez, and Michael Rueschman,“Scientific Reproducibility in Biomedical Research: Provenance Metadata Ontology for Semantic Annotation ofStudy,” AMIA Annual Symposium Proceedings (2016), pp. 1070-79, https://www.ncbi.nlm.nih.gov/pmc/articles/PMC5333253/; Jie Zheng, et al., “The Ontology of Biological and Clinical Statistics (OBCS) for standardized and reproducible statistical analysis,” Journal of Biomedical Semantics 7, 53 (2016), https://jbiomedsem.biomedcentral.com/articles/10.1186/s13326-016-0100-2; The OBO Foundry, http://obofoundry.org.
144 Jeffrey N. Rouder, “The What, Why, and How of Born-Open Data,” Behavior Research Methods 48, 3 (2016), pp. 1062-69, http://pcl.missouri.edu/sites/default/files/Rouder-BRM_0.pdf.
145 E.g., James P. Wallace, III, et al., “On the Validity of NOAA, NASA and Hadley CRU Global Average Surface Temperature Data & The Validity of EPA’s CO2 Endangerment Finding, Abridged Research Report,” June 2017, https://thsresearch.files.wordpress.com/2017/05/ef-gast-data-research-report-062717.pdf. See also Blakeley B. McShane and Abraham J. Wyner, “A Statistical Analysis of Multiple Temperature Proxies: Are Reconstructions of Surface Temperatures over the Last 1000 Years Reliable?” The Annals of Applied Statisitics 5, 1 (2011), pp. 5-44, https://arxiv.org/pdf/1104.4002.pdf.
146 E.g, Gary W. Oehlert, A First Course in Design and Analysis of Experiments (2010), http://users.stat.umn.edu/~gary/book/fcdae.pdf; Howard J. Seltman, Experimental Design and Analysis (2015), http://www.stat.cmu. edu/~hseltman/309/Book/Book.pdf; Natalie J. Blades, G. Bruce Schaalje, and William F. Christensen, “The Second Course in Statistics: Design and Analysis of Experiments?” The American Statistician 69, 4 (2015), pp. 326-33, http://www.tandfonline.com/doi/abs/10.1080/00031305.2015.1086437?journalCode=utas20.
147 E.g., New York University—Wagner, Research Methods, https://wagner.nyu.edu/education/courses/research-methods; European Society for Medical Oncology, Methods in Clinical Cancer Research, http://www.esmo.org/Conferences/Workshops-Courses/Methods-in-Clinical-Cancer-Research-MCCR; University of Maryland, University College, Research Methods in Psychology, https://www.umuc.edu/academic-programs/course-information. cfm?course=psyc300.
148 Edward R. Dougherty, The Evolution of Scientific Knowledge: From Certainty to Uncertainty (Bellingham, WA, 2016), http://spie.org/samples/9781510607361.pdf; Peter V. Coveney, Edward R. Dougherty, and Roger R. Highfield, “Big data need big theory too,” Philosophical Transactions of the Royal Society 374 (2016), October 3, 2016, http:// rsta.royalsocietypublishing.org/content/374/2080/20160153.
149 E.g., Edward R. Dougherty and Michael L. Bittner, Epistemology of the Cell: A Systems Perspective on Biological Knowledge (Hoboken, NJ, 2011).
150 E.g., Judea Pearl, “Causal inference in statistics: An overview,” Statistics Surveys 3 (2009), pp. 96-146, http://ftp. cs.ucla.edu/pub/stat_ser/r350-reprint.pdf.
151 Story C. Landis et al, “A call for transparent reporting to optimize the value of preclinical research,” Nature 490 (2012), pp. 187-91, https://www.nature.com/nature/journal/v490/n7419/full/nature11556.html; and see Force11, https://www.force11.org/.
152 Carole J. Lee and David Moher, “Promote scientific integrity via journal peer review data,” Science 357 (2017), pp. 256-57, http://science.sciencemag.org/content/357/6348/256?ijkey=aoQ8T2TirYWfM&keytype=ref&siteid=sci.
153 Sam Schwarzkopf, “Strolling through the Garden of Forking Paths,” NeuroNeurotic, September 25, 2016, https:// neuroneurotic.net/2016/09/25/strolling-through-the-garden-of-forking-paths/.
154 Dorothy Michelson Livingston, “Michelson-Morley: The Great Failure,” The Scientist, July 13, 1987, http://www.the-scientist.com/?articles.view/articleNo/8805/title/Michelson-Morley--The-Great-Failure/.
155 B. R. Jasny, et al., “Fostering Reproducibility in industry-academia research,” Science 357 (2017), pp. 759-61, http://science.sciencemag.org/content/357/6353/759.full.
156 Ali H. Mokdad, et al., “Actual Causes of Death in the United States, 2000,” JAMA 291, 10 (2004), http://www.csdp.org/research/1238.pdf; Shaoni Bhattacharya, “Obesity to surpass tobacco as top US killer,” New Scientist, March 10, 2004, https://www.newscientist.com/article/dn4763-obesity-to-surpass-tobacco-as-top-us-killer/; Bootie Cosgrove-Mather, “Americans Eat Themselves to Death,” CBS News, March 9, 2004, https://www.cbsnews.com/ news/americans-eat-themselves-to-death/; Nanci Hellmich, “Obesity on track as No. 1 killer,” USA Today, March 9, 2004, http://usatoday30.usatoday.com/news/health/2004-03-09-obesity_x.htm; David Teather, “Obesity close to smoking as cause of death in US,” The Guardian, March 10, 2004, https://www.theguardian.com/world/2004/mar/11/usa.davidteather.
157 Gina Kolata, “Data on Deaths From Obesity Is Inflated, U.S. Agency Says,” The New York Times, November 24, 2004, http://www.nytimes.com/2004/11/24/health/data-on-deaths-from-obesity-is-inflated-us-agency-says.html?_r=0.
158 Katherine M. Flegal, et al., “Excess deaths associated with underweight, overweight, and obesity,” JAMA 293, 15 (2005), pp. 1861-67, https://www.ncbi.nlm.nih.gov/pubmed/15840860.
159 Medical Evidence Boot Camp, World Federation of Science Journalists, http://www.wfsj.org/conferences/item.php?id=205.
160 David Funder, “NSF Gets an Earful about Replication,” Funderstorms, February 25, 2014, https://funderstorms.wordpress.com/2014/02/25/nsf-gets-an-earful-about-replication/.
161 Michael Stebbins, “Expanding Public Access to the Results of Federally Funded Research,” Obama White House Archives, February 22, 2013, https://obamawhitehouse.archives.gov/blog/2013/02/22/expanding-public-access-results-federally-funded-research.
162 National Institutes of Health, NIH Data Sharing Policy, “Final NIH Statement On Sharing Research Data,” February 26, 2003, https://grants.nih.gov/grants/guide/notice-files/NOT-OD-03-032.html; ImmPort, https://immport. niaid.nih.gov/home; ClinicalTrials.gov, https://clinicaltrials.gov/ct2/home.
163 Rigor and Reproducibility, National Institutes of Health, https://grants.nih.gov/reproducibility/index.htm; K.Andrew DeSoto, “NIH-Wide Policy Doubles Down on Scientific Rigor and Reproducibility,” Observer (Association for Pyschological Science), December 2016, https://www.psychologicalscience.org/observer/nih-wide-policy-doubles-down-on-scientific-rigor-and-reproducibility; National Institutes of Health, The Office of Behavioral and SocialSciences Research, Healthier Lives through Behavioral and Social Sciences: Strategic Plan 2017-2021, https://obssr.od.nih.gov/wp-content/uploads/2016/09/OBSSR-SP-2017-2021.pdf#.
164 Michael Stebbins, “Expanding Public Access to the Results of Federally Funded Research,” February 22, 2013, Obama White House Archives, https://obamawhitehouse.archives.gov/blog/2013/02/22/expanding-public-access-results-federally-funded-research.
165 “H.R.1030 - Secret Science Reform Act of 2015,” Congress.gov, https://www.congress.gov/bill/114th-congress/house-bill/1030.
166 Wallace D. Loh, Social Research in the Judicial Process: Cases, Readings, and Text (New York, Russell Sage Foundation, 1984); Murray Levine and Barbara Howe, “The Penetration of Social Science into Legal Culture,” Law and Policy 7, 2 (1985), pp. 173-98, http://onlinelibrary.wiley.com/doi/10.1111/j.1467-9930.1985.tb00350.x/abstract; Patricia J. Falk, “The Prevalence of Social Science in Gay Rights Cases: The Synergistic Influences of Historical Context, Justificatory Citation, and Dissemination Efforts,” Wayne Law Review 41, 1 (1994), pp. 1-69, http://engagedscholarship.csuohio.edu/cgi/viewcontent.cgi?article=1290&context=fac_articles; Stephen Breyer, “Science in the Courtroom,” Issues in Science and Technology 16, 4 (2000), pp. 52-56, http://issues.org/16-4/breyer/.
167 Joëlle Anne Moreno, “Einstein on the Bench?: Exposing What Judges Do Not Know About Science and Using Child Abuse Cases to Improve How Courts Evaluate Scientific Evidence,” Ohio State Law Journal 64 (2003), pp. 531- 85, http://moritzlaw.osu.edu/students/groups/oslj/files/2012/03/64.2.moreno.pdf;
David L. Faigman, “Judges as ‘Amateur Scientists,’” Boston University Law Review 86, 5 (2006), pp. 1207-25, http://www.bu.edu/law/journals-archive/bulr/volume86n5/documents/faigmanv.2.pdf; Ben K. Grunwald, “Suboptimal Social Science and Judicial Precedent,” University of Pennsylvania Law Review 161, 5 (2013), pp. 1409-43, http://scholarship.law.upenn.edu/cgi/viewcontent.cgi?article=1390&context=penn_law_review.
168 Stephanie Tai, “Uncertainty About Uncertainty: The Impact of Judicial Decisions on Assessing Scientific Uncertainty,” Journal of Constitutional Law 11, 3 (2009), pp. 671-727, http://scholarship.law.upenn.edu/cgi/viewcontent.cgi?article=1158&context=jcl.
169 For judicial decisionmaking conducted without such courses in statistics, see Gary L. Wells, “Naked statistical evidence of liability: Is subjective probability enough?” Journal of Personality and Social Psychology 62, 5 (1992), pp. 739-752, esp. p. 745, https://public.psych.iastate.edu/glwells/Wells%20pdfs/1990-99/Wells_1992_JPSP.pdf.
170 Rasmus E. Benestad, et al., “Learning from mistakes in climate research,” Theoretical and Applied Climatology 126, 3-4 (2016), pp. 699-703, https://link.springer.com/article/10.1007/s00704-015-1597-5; and see Gretchen F.Goldman, et al., “Ensuring scientific integrity in the Age of Trump,” Science 355 (2017), pp. 696-98, http://science. sciencemag.org/content/355/6326/696.
171 Andrew Gelman, “‘Why this gun control study might be too good to be true’,” Statistical Modeling, Causal Inference, and Social Science, March 11, 2016, http://andrewgelman.com/2016/03/11/why-this-gun-control-study-might-be-too-good-to-be-true/.
172 Joseph P. Simmons, et al., “False-Positive Psychology: Undisclosed Flexibility in Data Collection and Analysis Allows Presenting Anything as Significant,” Psychological Science 22, 11 (2011), pp. 1359-66, http://journals.sagepub.com/doi/pdf/10.1177/0956797611417632.
173 Irving Langmuir, “Pathological Science,” Colloquium at the Knolls Research Laboratory, December 18, 1953, https://www.cia.gov/library/readingroom/docs/CIA-RDP96-00791R000100490001-4.pdf.
174 James Rodger Fleming, Fixing the Sky: The Checkered History of Weather and Climate Control (New York: Columbia University Press, 2010).
175 “A complete list of things caused by global warming,” Number Watch, http://www.numberwatch.co.uk/warmlist.htm.
176 Madeleine Aruffo, “Problems with the Noble Lie,” The Core Journal 23 (2014), pp. 181-87, https://www.bu.edu/av/core/journal/xxiii/Aruffo.pdf.